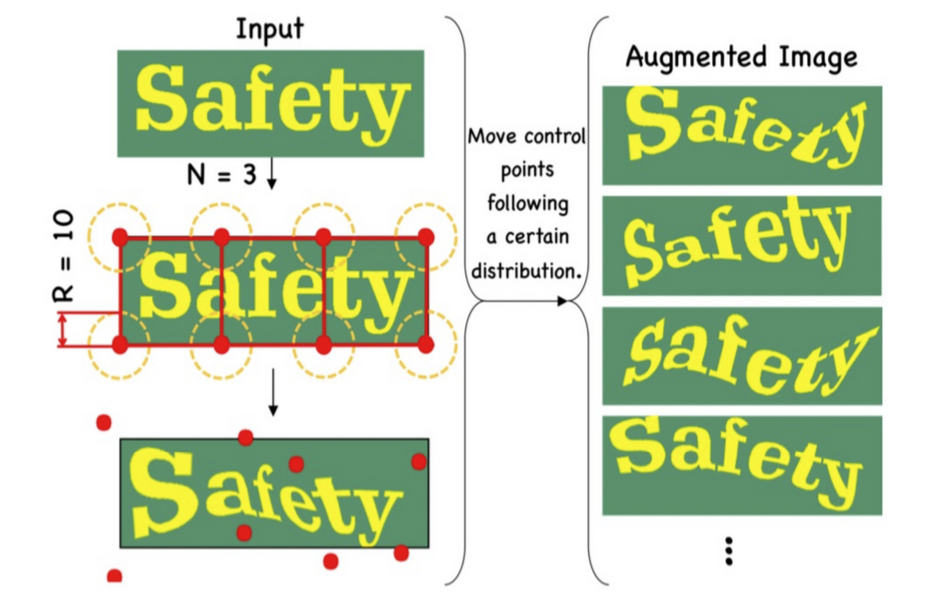
A group of researchers from Baidu has proposed and developed a novel ultra-lightweight optical character recognition system (OCR).
Their, so-called PP-OCR is based on lightweight deep neural networks with several improvements that researchers propose. The OCR framework described in their paper is a 3-stage framework that starts with a text detection module, followed by a rectification module, and finally a text recognition module.
All of the three modules employ light backbone networks to improve computational efficiency and make the method suitable for embedded applications. The first module uses a text detector based on a segmentation network whose goal is to just detect and segment the area of the image that contains the text. In the second step, a geometric transformation to the image area is applied to rectify the detection box. In the final stage – text recognition, a convolutional recurrent neural network (CRNN) is used to recognize the text from the rectified bounding box.
Researchers include several tweaks or enhancement strategies to make the method powerful and efficient at the same time. For example, they used an FPGM pruner, a PACT quantization technique, learning rate warm-up etc.
As part of this work, researchers collected a large-scale dataset for Chinese and English language. There are three smaller datasets that combine together into a large dataset for training the proposed system: a text detection dataset with 97K images, a direction classification dataset with 600k images, and a text recognition dataset that contains 17.9M images.
Researchers conducted several experiments to showcase the performance of their proposed method. The final model for Chinese character recognition that they trained is only 3.5M, and 2.8M for recognition of 63 alphanumeric characters.
The implementation and the trained lightweight model was released together with the collected dataset. Details about the training procedure and the ablation studies can be read in the paper.