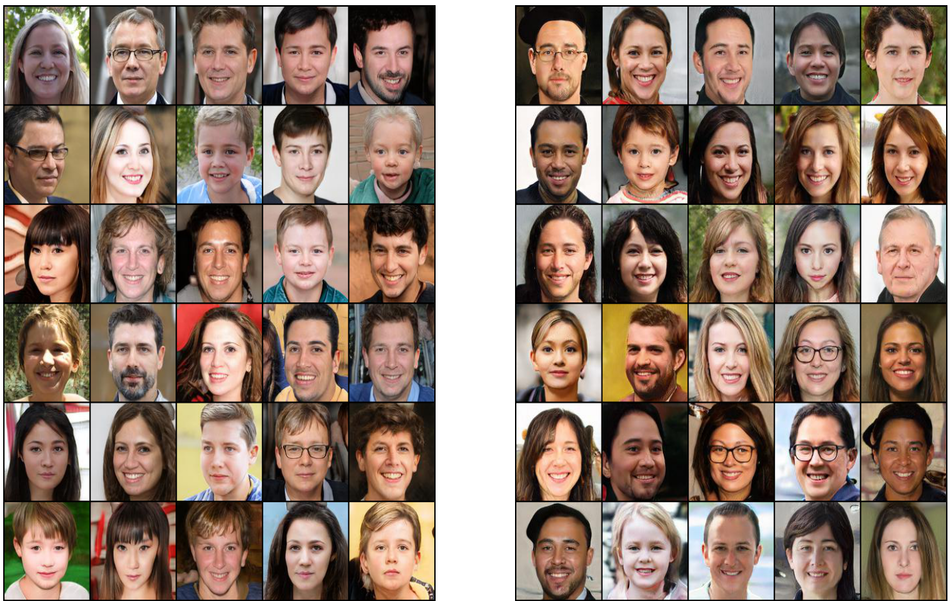
A group of researchers from Columbia University, the University of Cambridge and Google DeepMind, has proposed a novel type of Generative Adversarial Networks (GANs) – Prescribed Generative Adversarial Networks.
The new GAN model addresses two major problems and limitations of Generative Adversarial Networks in general: mode collapse and the difficulty to evaluate the generalization capabilities. GANs have had a major success in a number of generative tasks in the past several years. However, the two mentioned limitations have not been successfully overcome by researchers.
Prescribed GANs are solving these two issues by optimizing an entropy-regularized adversarial loss and by adding noise to the output of a density network. This means that PresGANs models are maximizing the entropy of the generative distribution, which in turn provides a learned distribution with preserved modes. Moreover, the added noise has additional positive effects such as stabilizing the training procedure, which for GANs is generally-known to be unstable and providing approximations of the predictive log-likelihood.
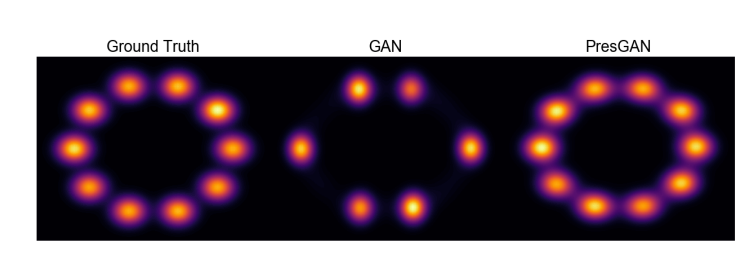
Researchers evaluated the proposed PresGANs on several datasets and showed that they can solve the problem of mode collapse and generate samples with very high perceptual quality. Moreover, they found that adversarial loss is the key component to high generated sample quality, and for this reason, GANs very often outperform models such as Variational Autoencoders (VAEs).
A more detailed description of the method and the evaluations can be found in the paper published on arxiv.