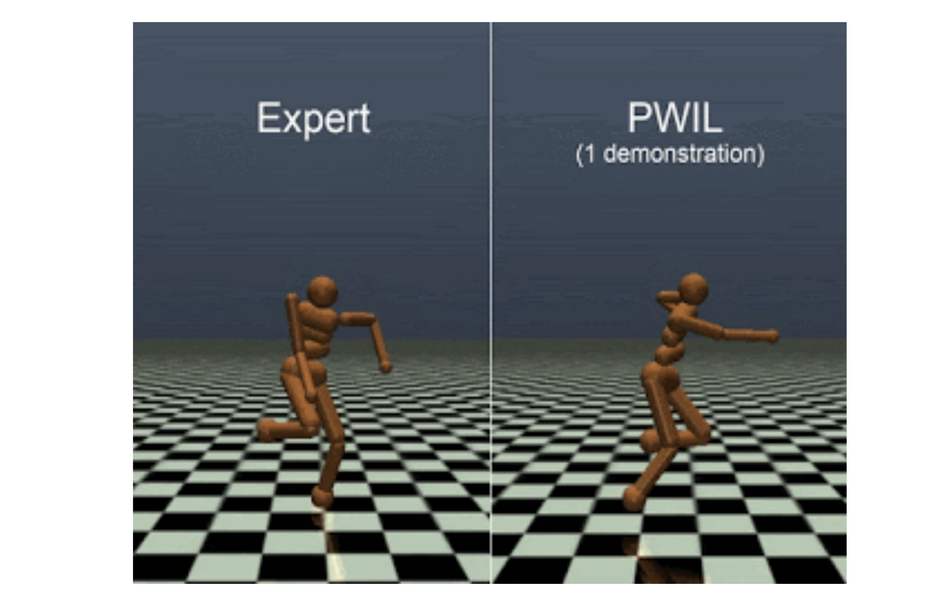
A group of researchers from Google AI has developed a new imitation learning method called Primal Wasserstein Imitation Learning (PWIL).
The newly proposed method for imitation learning does not rely on adversarial training as opposed to state-of-the-art imitation learning (IL) methods. Typically those methods employ an adversarial framework for learning behavior from an expert. In such a setting, a generator or the policy is trained to maximize the confusion of the discriminator, which in turn has to learn to distinguish an agent from an expert behavior (or state-action pairs in the particular case).
However, these methods often suffer from training instability, similar to other adversarial learning frameworks for example GANs. In order to overcome this problem, researchers propose a new non-adversarial method, which can achieve similar results without the adversarial training regime which can potentially introduce numerous problems.
The PWIL learning method is actually using the same formulation as the adversarial training, where it boils down to a distribution matching problem. The main difference is the non-adversarial training where the distance between distributions is minimized by minimizing the Wasserstein distance between the agent and the expert’s distribution (or state-action pairs). So, PWIL manages to bypass the min-max optimization performed in adversarial training methods. Researchers showed that the agent can recover expert behavior using this type of learning.
This work opens up many possibilities for exploring non-adversarial imitation learning methods. More details about the research and the conducted experiments can be read in the arxiv paper.