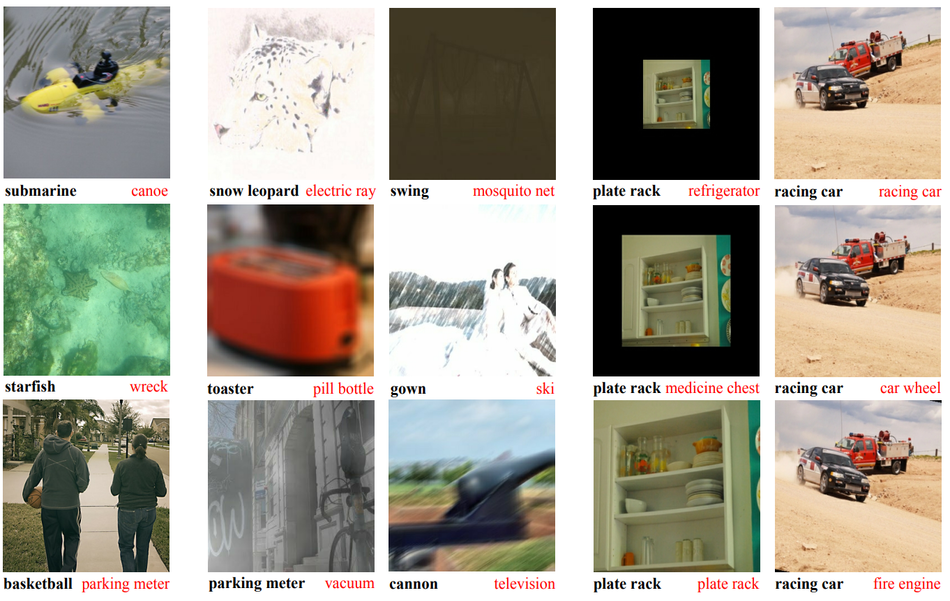
Researchers from Google Brain and Carnegie Mellon University have proposed a novel training method that improves ImageNet classification accuracy by 1%.
The new state-of-the-art image classification model is built using self-training within a student-teacher training framework. Researchers leveraged the large amounts of unlabeled data available in order to build a method that overperforms current state-of-the-art methods without the effort of manually labelling training sets.
The method, which was called “self-training with noisy student”, works by combining both labeled and unlabeled images. It takes a set of labeled images to train a teacher network model using standard cross-entropy loss used for classification. Then, this teacher model is used to generate soft and hard “pseudo” labels (continuous and one-hot encoder distributions) for the large unlabeled dataset. A student model is trained using the newly labeled dataset combined with the other labeled data, again using cross-entropy loss. The process is then iteratively repeated by using the trained student network as a teacher in order to generate new pseudo labels and train a new student network. The teacher network is trained without any noise, while the student network is trained by injecting different kind of noise: data augmentation, dropout, stochastic depth. In this way, according to researchers, the student network is forced to learn harder the more precise (not noisy) labels produced from the teacher network.
For the experiments that researchers conducted, they used EfficientNet as their base model which was initially trained as a teacher network and iteratively upgraded. Results showed that the method outperforms current state-of-the-art methods on several baselines. It achieves 1.0% better classification accuracy than the state-of-the-art method on ImageNet top-1 classification. The method was evaluated also on robustness test sets, where it improves SOTA accuracy by large margins: from 16.6% to 74.2% on ImageNet-A accuracy, from 45.7 to 31.2 on ImageNet-C mean corruption error(mCE) and from 27.8 to 16.1 on ImageNet-P for the mean flip rate (mFR) metric.