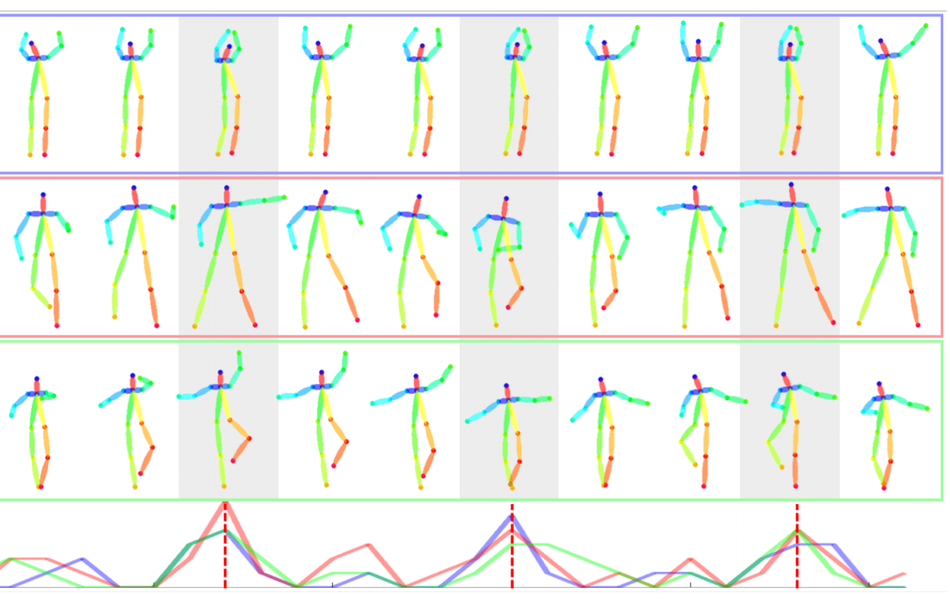
A group of researchers from the University of California and NVIDIA has published a method for modeling the dance-to-music process of humans.
Their model is able to learn how to decompose a dance into a series of basic dance units according to given input music. Emphasizing that learning to model the music-to-dance process in a non-trivial and challenging task, researchers decouple the problem and frame it into a synthesis-by-analysis framework, where a model learns how to move first and then learns how to compose a dance.
The problem of learning to dance according to some music rhythm was tackled from the perspective of generative modeling, and researchers designed a framework that utilizes Generative Adversarial Networks (GANs) and Variational Autoencoders (VAEs). There exist three larger phases (or modules) in the proposed framework: decomposition, composition, and testing. Within this framework, in the decomposition phase, a kinematic beat detector is used to segment dance units from real dancing sequences. These segments are then modeled using a DU-VAE model. In the next phase – composition, an MM-GAN network is used to learn how to organize the individual dance units into a coherent dance conditioned on the given music. Last, during the testing phase, a music style extractor is used to extract style and beats, which are later used for synthesizing a dance in a recurrent manner.
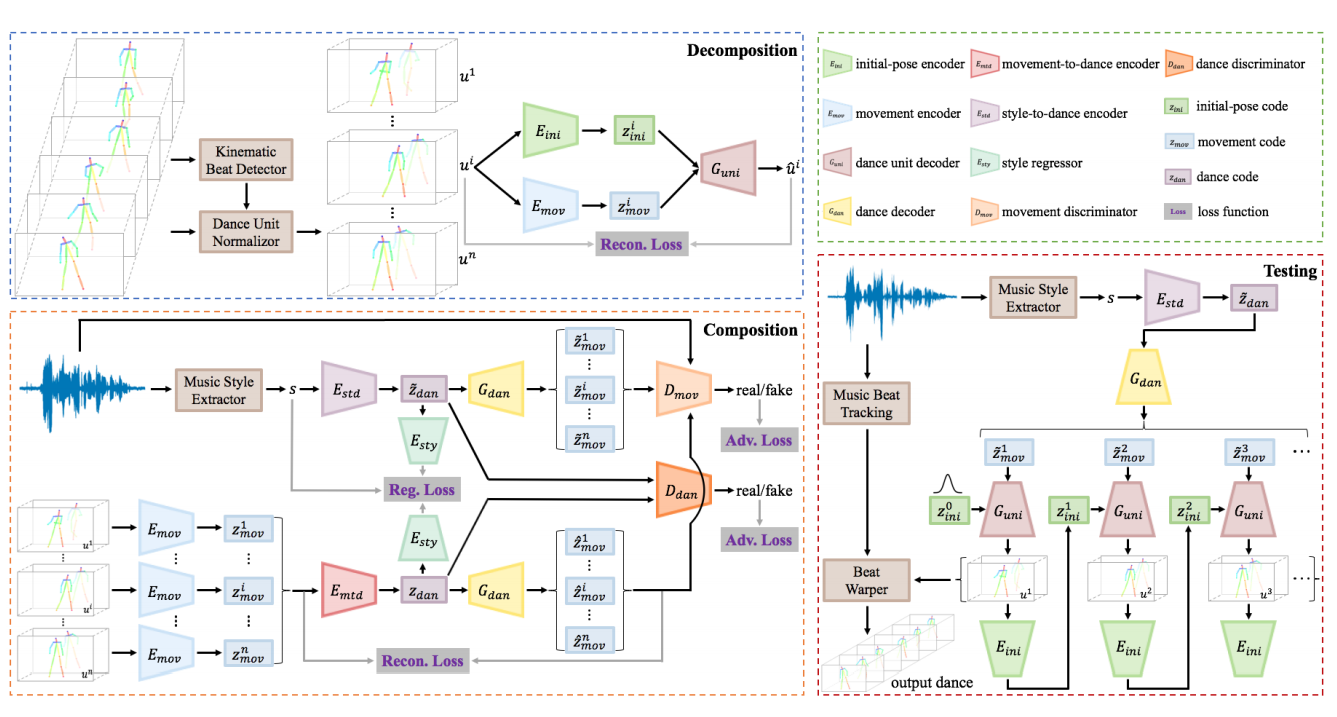
In order to evaluate the proposed method, researchers decided to perform a user study and qualitatively assess the capabilities of their method. Consistency of the style and motion realism were the kind of parameters that were evaluated within the study. The results of the study show that the method is able to generate naturalistic and musically meaningful dancing patterns.