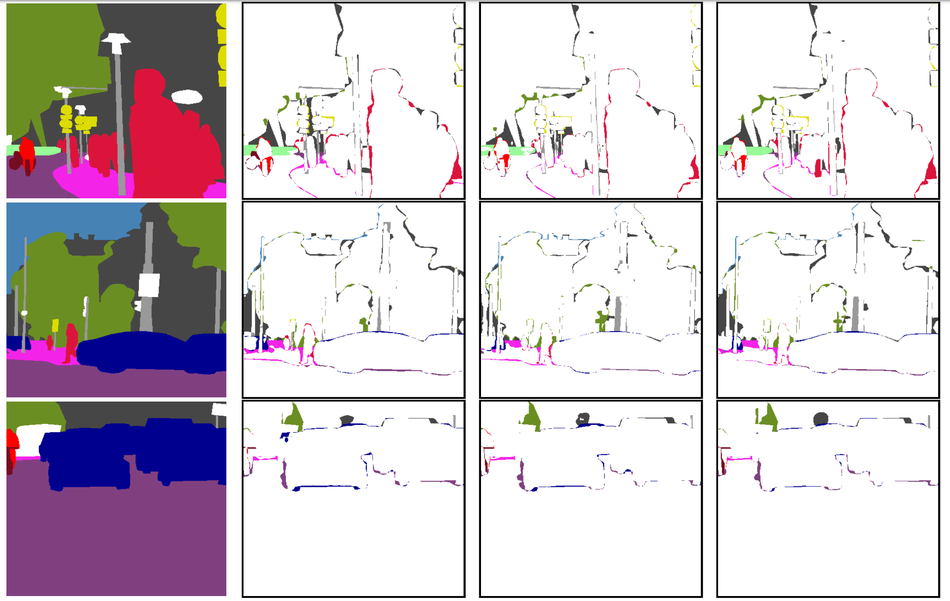
Researchers from the University of Chinese Academy of Sciences, University of Science and Technology in China and Microsoft have joined forces on a project to develop a boundary refinement method for segmentation.
The resulting method, called SegFix manages to reduce significantly the boundary errors in segmentation, produced by almost any segmentation model. It relies on a novel framework for boundary refinement that is in fact model-agnostic. Within this framework, a segmentation mask is refined by using a so-called offset map which is learned from data. The proposed method learns a binary boundary map along with a direction or offsets map. In other words, the method tries to first localize the boundary pixels and label them, and afterward, it identifies the corresponding “interior pixel” for each pixel of each object.
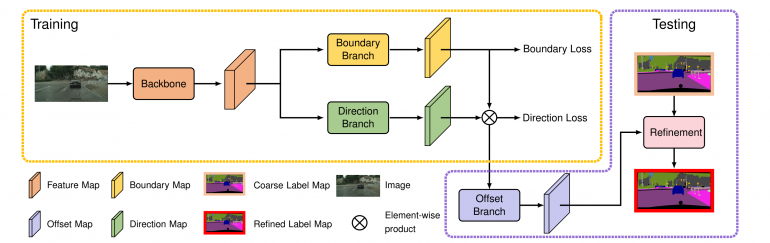
In this way a segmentation mask can be corrected or refined at the boundaries, knowing the object’s interior pixels and pixel directions. According to researchers, the proposed method archieves real-time inference speed and it is able to reduce boundary errors for various state-of-the-art models on popular datasets such as CityScapes, ADE20K, and GTA5.
The implementation of the method was open-sourced and is available on Github. More details about the novel refinement framework can be read in the paper published on arxiv.