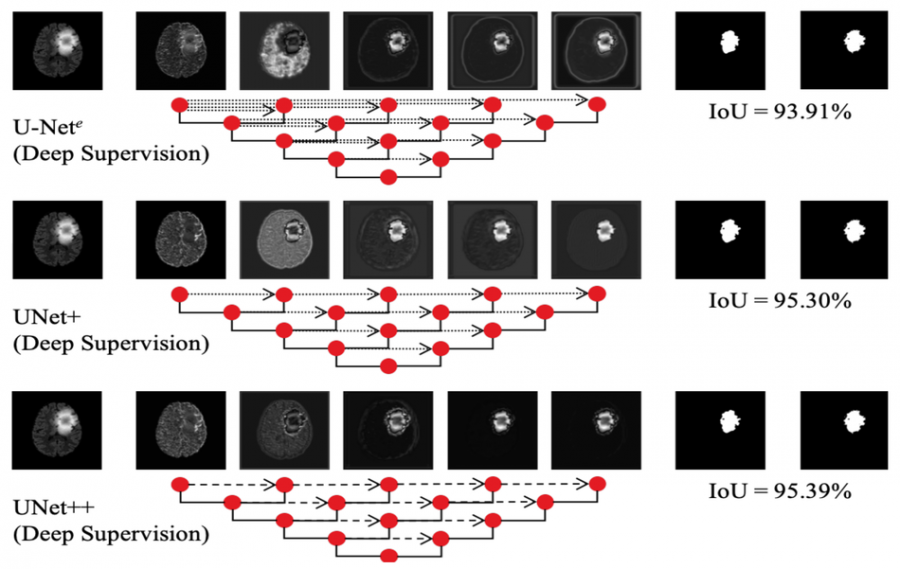
A group of researchers from Arizona State University has published a new modified UNet architecture that improves the results in image semantic segmentation. In their novel paper, researchers argue that the design of UNet – a popular and widespread model for semantic segmentation, is not optimal and they propose several modifications to make the network more robust.
In fact, researchers argue that UNet’s architecture directly enforces aggregation of features of same scale from the encoder and decoder networks. Moreover they argue that the optimal depth of UNet-shape networks is unknown and therefore a more extensive architecture search is necessary.
To alleviate these two shortcomings of the UNet architecture they propose UNet++ – an improved architecture where the unknown depth problem is solved by using an efficient ensemble of U-Nets of varying depths and where the skip connections are modified and extended across multiple scales. According to researchers, the latters allows for more flexible feature aggregation schemes and consequently provides better results in the task of semantic segmentation.
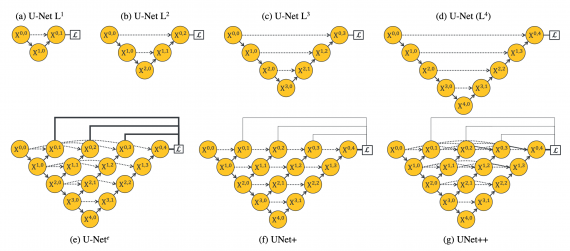
To evaluate the performance of the proposed method researchers used six different datasets from the medical imaging domain and several segmentation models, whose architecture is based on the UNet schema. The evaluations showed the improvements that UNet++ introduces over fixed-depth models such as UNet and Mask-RCNN. Moreover, researchers investigated pruning the UNet++ model and they showed that pruned UNet++ achieves significant speedup with only minor degradations in performance.
The implementation of the new method was open-sourced and it’s available here under the MIT license. More in detail about the method or the evaluations can be read in the pre-print paper.