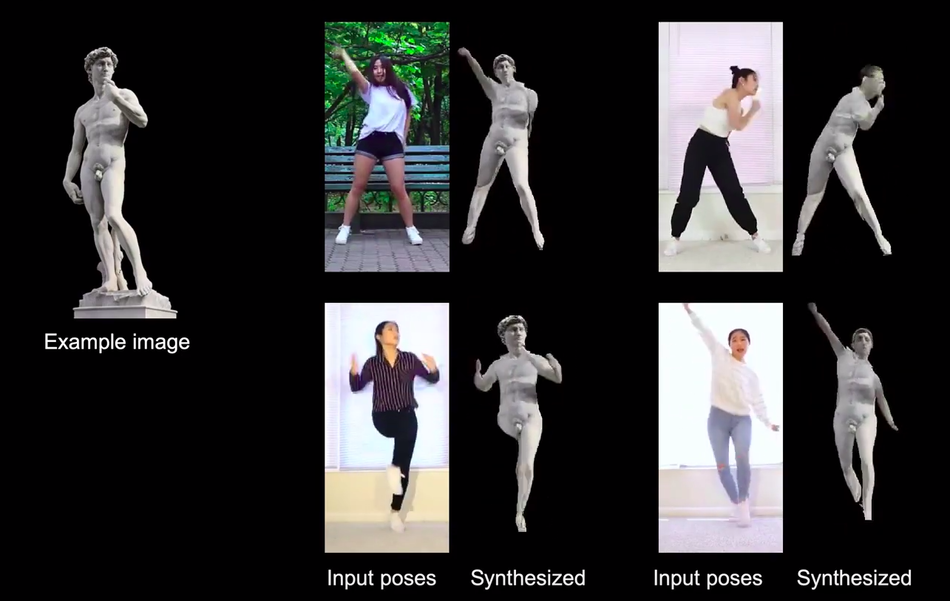
A group of researchers from NVIDIA has proposed a new method that learns to synthesize videos of unseen objects by using only a few example images of those objects.
The novel method, or more precise “framework”, aims to address the limitations of traditional video-to-video synthesis (vid2vid) methods. The major such limitation is the inability to synthesize realistic videos of unseen objects i.e. low generalization capabilities.
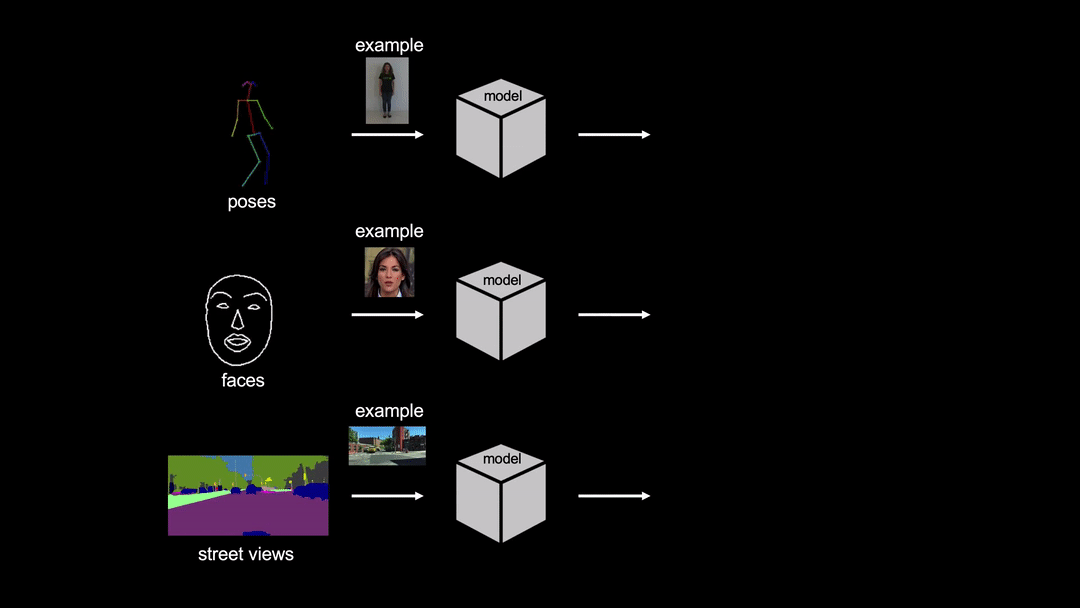
In order to tackle this problem, researchers propose few-shot video-to-video synthesis that leverages the power of an attention mechanism. They employ a specific architecture that utilizes a “network weight generation” module that can map example images to the part of the network for video synthesis. In this way, the synthesis network can take external inputs directly from the target domain.
Researchers showed that their model can learn to synthesize realistic videos of objects from the target domain having seen only a few sample images. They evaluated their solution using three video datasets: Youtube dancing videos, Street-scene videos and Face videos. Frechet Inception Distance and Pose error were used as evaluation metrics to compare the proposed method with existing methods. The quantitative evaluations showed that the method outperforms existing pose transfer methods by a large margin.
The implementation of the method was open-sourced and it is available on Github. More details about the model can be read in the paper or on the project website.