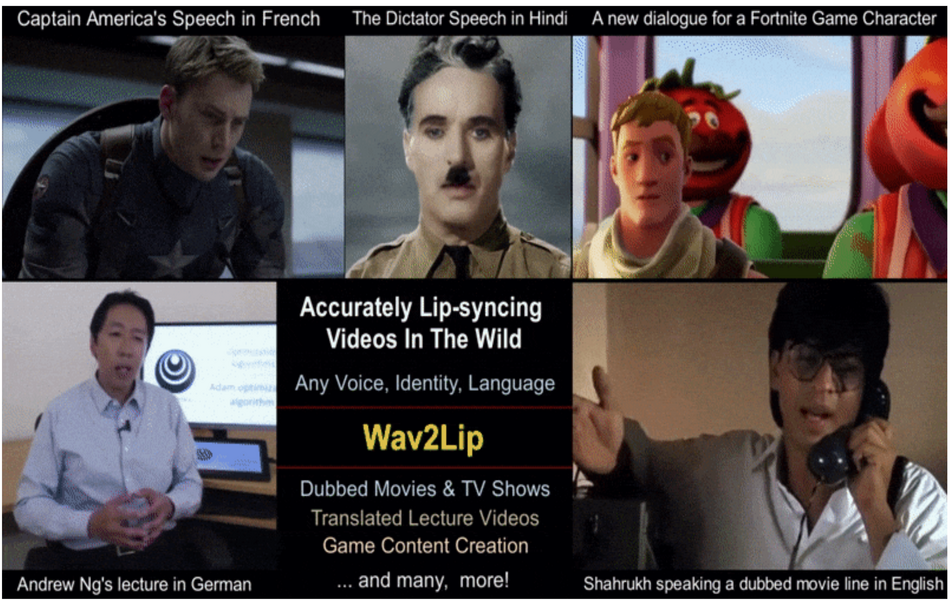
A group of researchers has developed and released a novel deep neural network that can convert a video and audio signal into a lip-synced video.
In their recent paper, the researchers explore the problem of lip-syncing of a talking face video, where the goal is to match the target speech segment to the lip and face expression of the person in the video. They argue that existing approaches and models struggle to produce satisfactory results and they attribute this to two identified reasons. According to them, the first reason is the inadequate loss functions that were employed when training these models (such as L1 reconstruction loss and LipGAN discriminator loss) and the second reason is poorly trained discriminator network with noisy and generated data.
In order to overcome these problems, researchers propose an approach where they decouple the learning problem and the learning framework into two major components: learning accurate lip-sync by introducing a sync loss and learning to generate qualitative visual results via a visual quality discriminator. For the first part, they use an already proven “expert” model – SyncNet, which was built to correct lip-sync errors. This model was fine-tuned with the generated face videos in order to further improve its accuracy and stability. A diagram of the proposed framework can be seen in the image below.
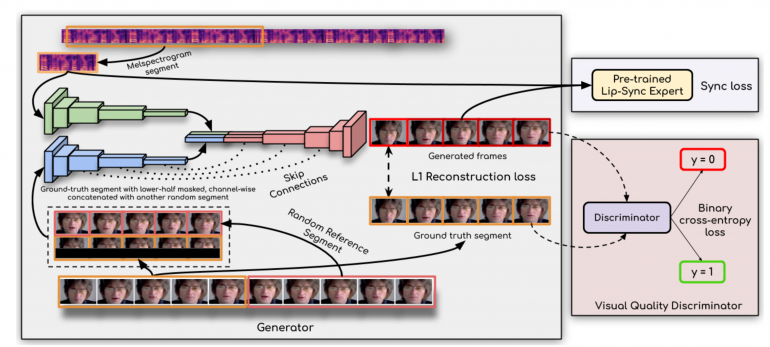
Researchers trained the proposed model on a single dataset – LRS2, but they tested it using 3 additional datasets. Results suggest that the novel Wav2Lip model outperforms all existing models by a significant margin.
The implementation of the method was open source and can be found on Github. More details about the method and the experiments can be read in the paper or in the project’s official website.