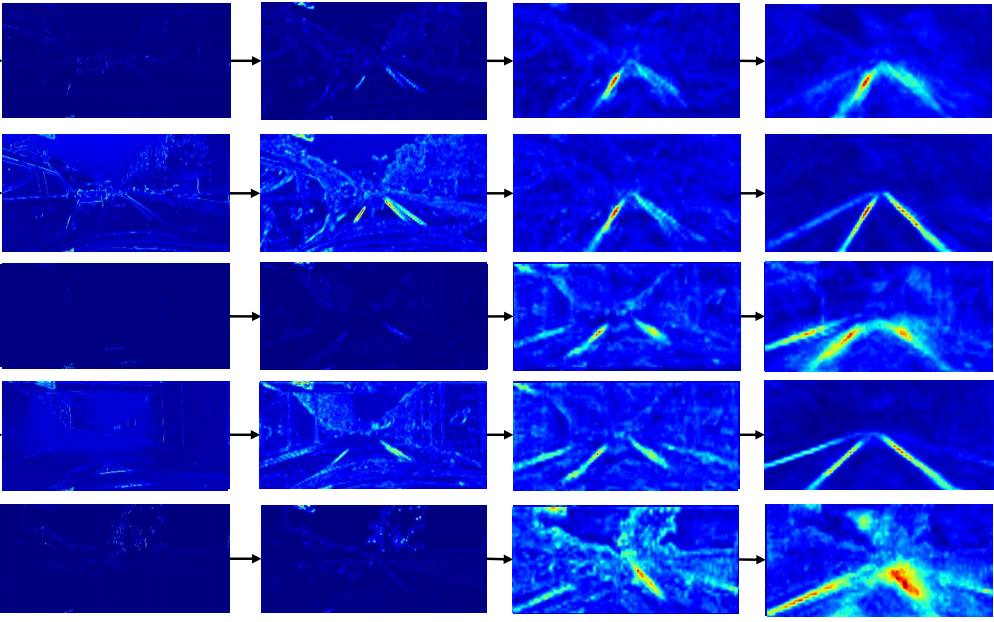
A group of researchers, led by Yuenan Hou from the Chinese University of Hong Kong has released a new state-of-the-art method for lane markings detection.
The problem of lane markings detection has attracted a lot of interest in the past decade or so. The reason for this is the fast growth of autonomous driving as a novel technology. Detecting lane markings is one of the most important tasks within the framework of self-driving vehicle systems.
In their project, Yuenan et al., take an interesting approach towards solving this problem. They proposed a method based on convolutional neural networks (CNNs) which incorporates the self-attention mechanism.
The method is based on a new knowledge distillation approach which the researchers named “Self Attention Distillation”. In fact, this allows the model to learn from itself over time, without any explicit supervision. The mechanism allows attention maps from trained models to be used as a form of supervision signal and guide the training procedure.
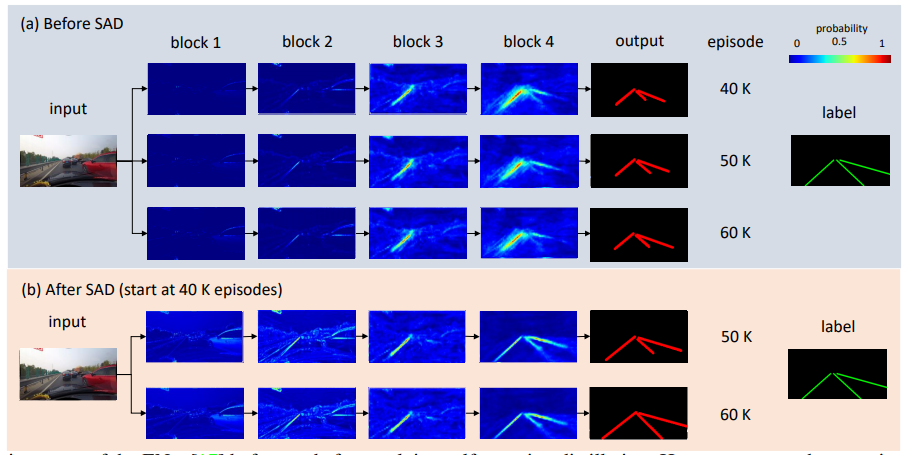
Researchers designed a lightweight convolutional neural network which incorporates the novel knowledge and attention distillation approach for solving the task of lane markings detection. According to them, the proposed SAD approach can be easily incorporated with any kind of convolutional neural network model.
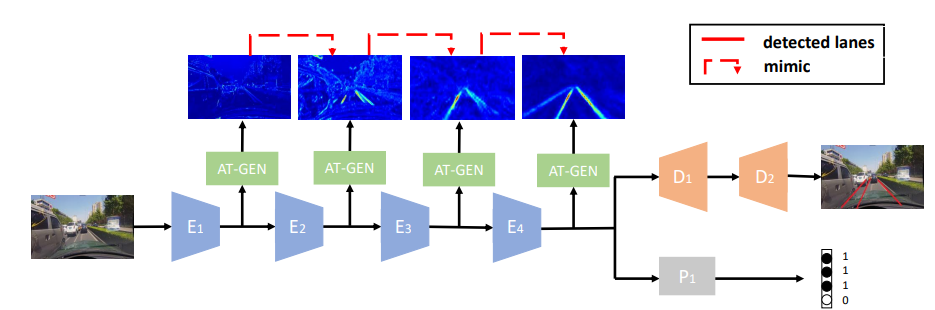
The experiments and evaluations showed that the model achieves state-of-the-art results on the popular TuSimple dataset, beating existing approaches using way fewer model parameters. As an example, the previous state-of-the-art approach – SCNN has 20 times more learnable parameters and has 10 times slower inference performance as compared with the proposed model.
The implementation of the model was open-sourced and can be found in the Github repository. More details about the method can be read in the pre-print paper published on arxiv.