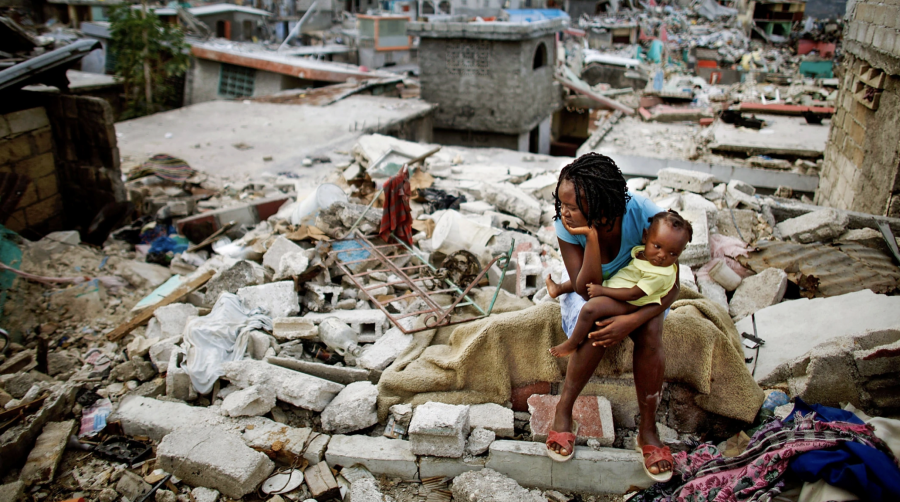
Researchers at Stanford University have developed an algorithm for removing background noise from data coming from seismic activity sensors. The model allows you to register four times more earthquake signals.
Traffic and the work of industries generate noise, making it difficult to distinguish an approaching earthquake among other vibrations in noisy cities. Algorithms trained to filter out this background noise can be especially useful for monitoring stations in and around busy earthquake-prone cities in South America, Mexico, the Mediterranean, Indonesia and Japan.
The developed UrbanDenoiser algorithm improves the capabilities of earthquake monitoring networks in cities by filtering out background seismic noise. As a result, it turns out to restore signals that were previously too weak to register. The model was trained on a dataset of 80,000 records of urban seismic noise and 33,751 records containing signals corresponding to earthquakes. The data was collected in the California areas of Long Beach and San Jacinto.
After training, the model was tested on earthquake data from 2014. UrbanDenoiser detected four times more seismic signals in the filtered data compared to the officially registered number.