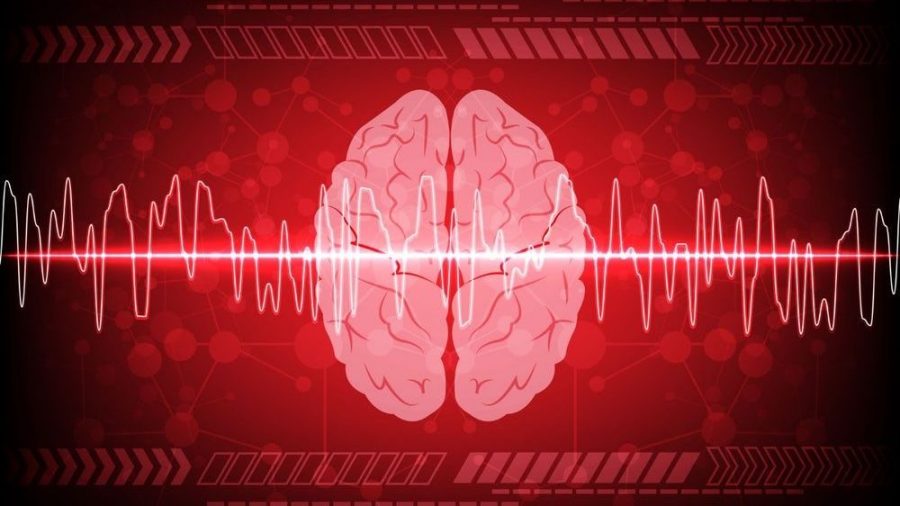
In an article published on 29 January in Nature, researchers from Columbia University, New York presented a method for reconstructing intelligible speech from the human auditory cortex using deep neural networks.
How the human auditory system extracts perceptually relevant acoustic features of speech is still an unresolved question. To find the possible answer, researchers led by Hassan Akbari have proposed a method that combines the recent advances in deep learning with the latest innovations in speech synthesis technologies to reconstruct closed-set intelligible speech from the human auditory cortex.
The problem tackled is, in fact, an inverse mapping that is supposed to find the best approximation of the speech from the neural activity. This means that the problem is non-trivial and more complex speech reconstruction techniques are required in order to solve it.
For this reason, and the success of deep learning applied in acoustic and audio signal processing, the researchers decided to examine a neural network approach. According to them, deep neural network models can improve reconstruction accuracy by imposing more complete constraints on the reconstructed audio by better modeling the statistical properties of the speech signal.
They examined the effect of three factors on the reconstruction accuracy: 1) the regression technique (linear regression versus nonlinear deep neural network), 2) the representation of the speech intended for reconstruction (auditory spectrogram versus speech vocoder parameters), and 3) the neural frequency range used for regression.
Finally, they show that a deep neural network model that directly estimates the parameters of a speech synthesizer from all neural frequencies achieves the highest subjective and objective scores on a digit recognition task. More about the dataset and the neural network architecture used can be read in the original article in Nature. Additionally, researchers provide subjective and objective evaluations of the reconstructed speech. Also, the code was open-sourced and it can be found here.
It is worth mentioning that reconstructing speech from the neural responses recorded from the human auditory cortex opens up the possibility of using this technique as a speech brain-computer interface (BCI) to restore speech in severely paralyzed patients. The proposed method and framework are just a next step towards this goal.