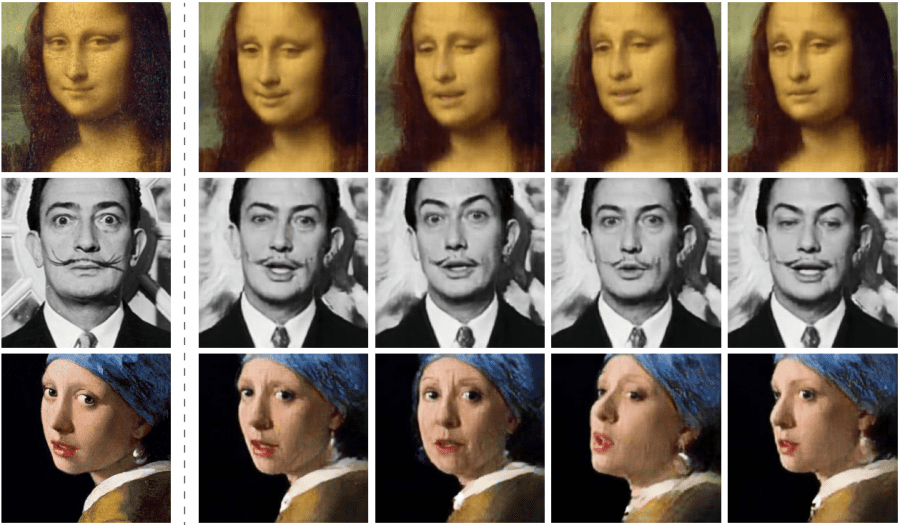
Researchers from Samsung AI and Skolkovo Institute of Science and Technology have presented a neural network that can create realistic fake videos of a talking head, given only a few images of that person.
In their paper, named “Few-Shot Adversarial Learning of Realistic Neural Talking Head Models”, researchers propose a method that is able to generate a personalized talking head model without the need for a large number of images of a single person.
Arguing that in practical scenarios such videos need to be generated only using a few image samples or even a single one, they designed a few-shot learning scheme.
The proposed architecture contains three modules: a generator network, an embedder network, and a discriminator network. The architecture was designed in such a way that it disentangles the pose and person’s facial features and exploits the adversarial learning technique to generate realistic videos.
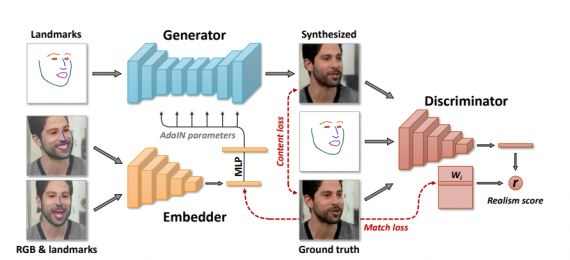
The embedder network is the module which extracts pose-independent features of the person in the given frame. It is supposed to learn the person’s identity and generate low-dimensional embeddings. These embeddings are then fed in the generator network as AdaIN parameters (Adaptive Instance Normalization). This allows convolutional layers to be modulated with the latent embeddings containing person-specific information.
The generator network takes facial landmarks as input (as well as the embeddings and the ground truth image) and it is supposed to produce a synthetic image sample of a person as a video frame.
Finally, the discriminator network should learn to discriminate the distributions and force the generator to produce samples from the realistic distribution.
Researchers trained the system in a supervised manner using two datasets with talking head videos: VoxCeleb1 and VoxCeleb2. The evaluation showed that the proposed method is able to learn to generate a talking head video from as little as one single image sample. However, the best results are reported with the model trained using 32 images.