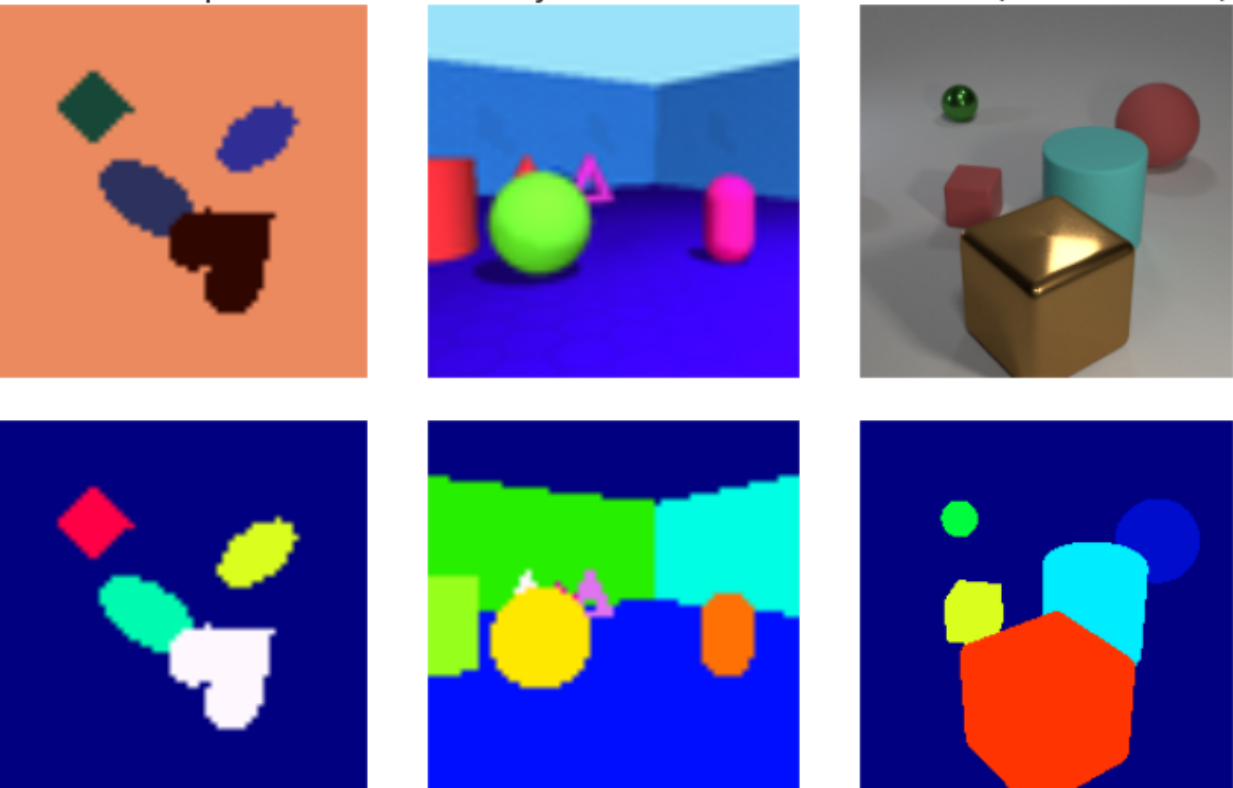
Deepmind researchers have released new datasets for multi-object representation learning. The datasets (4 in total) contain multi-object scene images, where each of the images is accompanied by ground truth data in the form of segmentation masks for all objects present.
The purpose of these datasets is to be used for the development of scene decomposition methods. Researchers released 4 large datasets:
1. Multi-dSprites (a dataset containing images of 2D shapes in the form of sprites)
Multi-dSprites consists of three different versions of the same dataset: binarized (where images have 2-3 white sprites), color sprites on grayscale and colored sprites on a background. The dataset contains 1 million data points for each of these versions.
2. Objects Room (images of room scenes with 3D objects)
The second dataset in this series is the Objects Room dataset. It contains 1 million scenes with up to three objects per scene.
3. CLEVR (high quality rendered images of scenes from CLEVR dataset)
Researchers adapted the CLEVR dataset released in 2017 by Johnson et al. and produced segmentation masks for the scenes. They generated new images so, the new dataset is different than the original CLEVR dataset.
4. Tetrominoes (a dataset of Tetris-like shapes)
The last dataset is the Tetrominoes dataset which contains images of Tetris-like shapes. The images are of size 35×35 and each image contains three shapes (tetrominoes) from a set of 17 shapes/orientations and each shape is colored with 6 colors.
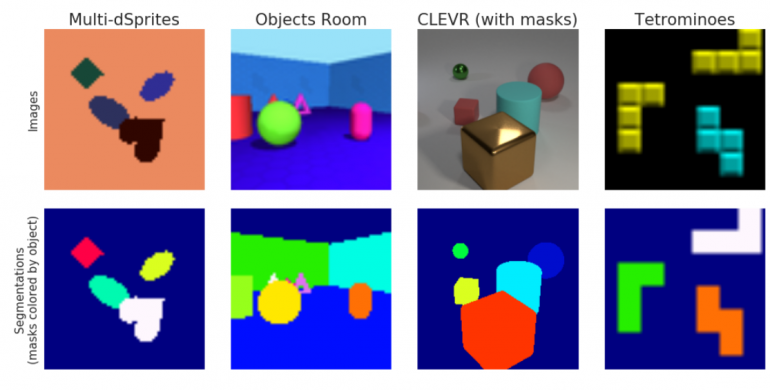
All the datasets are open-sourced and are available from the Google Cloud Storage, each of them stored as a TFRecords file, for ease of use with Tensorflow. Researchers also provided instructions on how to load and use the data from the dataset.