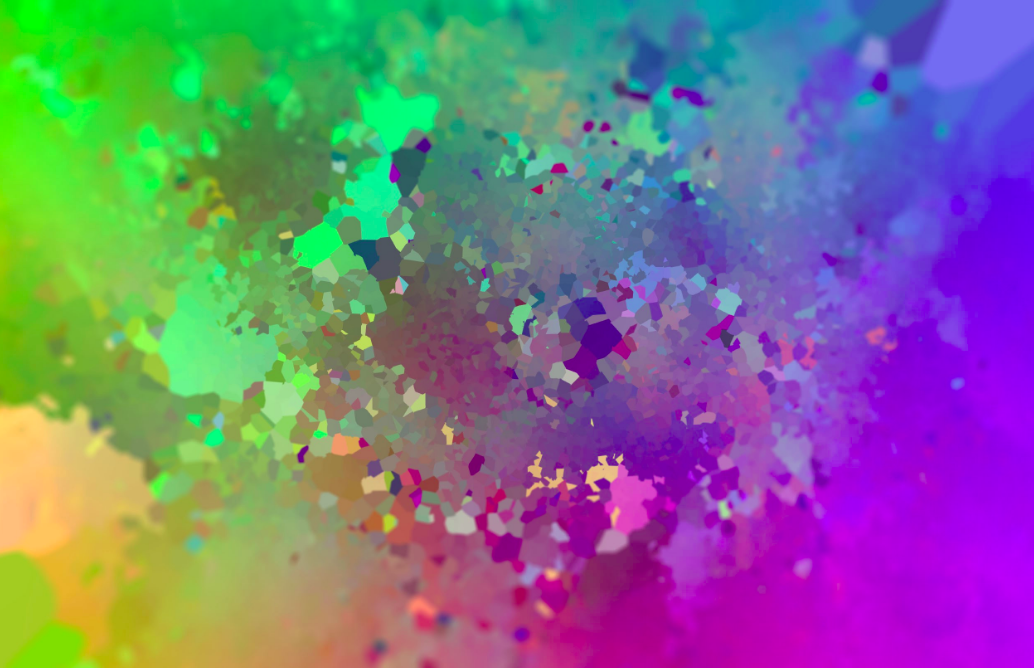
Researchers from the University of Amsterdam proposed a method that can translate visual art into music. Their method, called SynVAE or Synesthetic Variational Autoencoder leverages the power of deep autoencoder networks to learn a mapping between an image (a visual signal) and an audio signal.
A specific architecture was designed by the researchers that uses already proven models for visual and auditive data: β-VAE and MusicVAE proposed in the past few years. The beta-Variational Autoencoder (β-VAE) takes an image as input and encodes it into a lower-dimensional latent representation. Then, the latent vector is passed to the audio autoencoder (the MusicVAE) in order to encode it into a latent representation in a different latent space. The MusicVAE encoded vector is then passed to a decoder that reconstructs the image. This allows training of the architecture to be performed in a completely unsupervised manner. In the end, the reconstruction model has learned to encode an image (or visual art) into music using the reconstruction mechanism and the MusicVAE autoencoder.
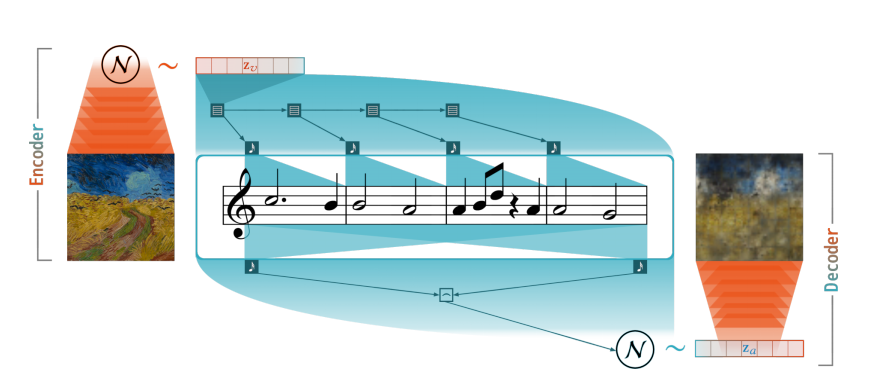
In the absence of audio-visual ground truth, researchers defined a loss function using KL divergence metric and fixed the weights of the MusicVAE model in order to encourage high-variability in the latent space.
Researchers performed a quantitative and qualitative evaluation of the method using MNIST and Behance Artistic Media Datasets. They conveyed a human evaluation experiment where people were asked to judge the correspondence between a musical sample and the image which it was generated from. The results showed that the musical samples were matched with an accuracy of up to 73%.
More about SynVAE can be read in the paper published on arxiv.