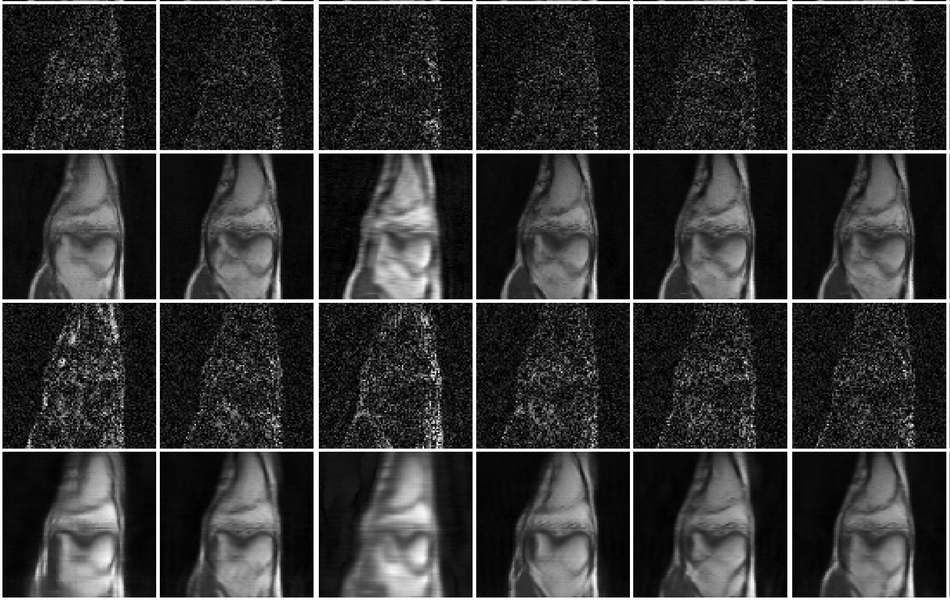
A group of researchers from Facebook AI Research and McGill University has released a new state-of-the-art method for active MRI acquisition that uses Reinforcement Learning.
In their novel paper, “Active MR k-space Sampling with Reinforcement Learning”, researchers describe an interesting idea, where they tackle the problem of active MRI acquisition from the perspective of acquisition trajectory instead of MRI scan reconstruction. In simple words, researchers decided to focus on which k-space measurements to be taken instead of taking (more or less) arbitrary measurements and focusing on the reconstruction of the full scan.
They frame the problem of trajectory optimization as a sequential decision process and used Double Deep Q-Networks (DDQN) to learn how to select the optimal scanning trajectory. DDQN was chosen as the state-of-the-art reinforcement learning method for problems that are defined in the framework of partially observable Markov processes.
The proposed method was trained and evaluated using knee MRI scans which are part of the large fastMRI dataset. Results showed that the method (using the DDQN model) outperforms all baselines that researchers defined for a large number of acceleration factors measured. Researchers used metrics such as MSE, NMSE, SSIM, PSNR, etc., to quantify the improvement that the proposed method brings over existing methods and baselines.
This research is part of the larger research project from Facebook AI, which aims at improving the speed and accuracy of MRI scanning procedures – fastMRI. In addition to the new method, researchers announced that they are releasing a Reinforcement Learning environment for active MRI acquisition.
More details about the method, results or the novel training environment can be read in the official blog post or in the arxiv paper.