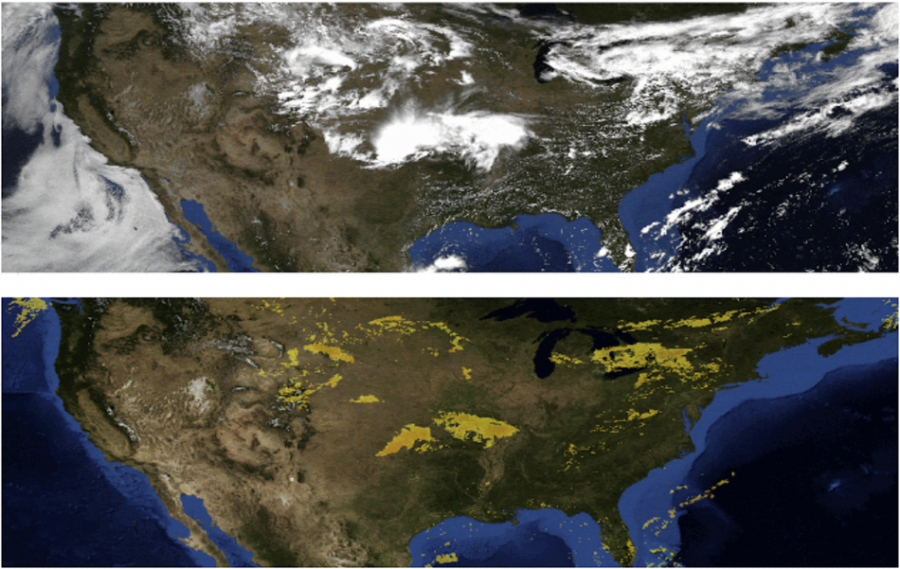
In a recent paper, researchers from Google AI Research propose a method for precipitation forecasting using deep learning and convolutional neural networks.
Their approach, different than traditional weather models, does not incorporate any prior knowledge about the atmosphere, it’s physical or chemical properties. The problem of precipitation forecasting was framed as an image-to-image translation problem and it was addressed using advanced computer vision methods that involve CNNs.
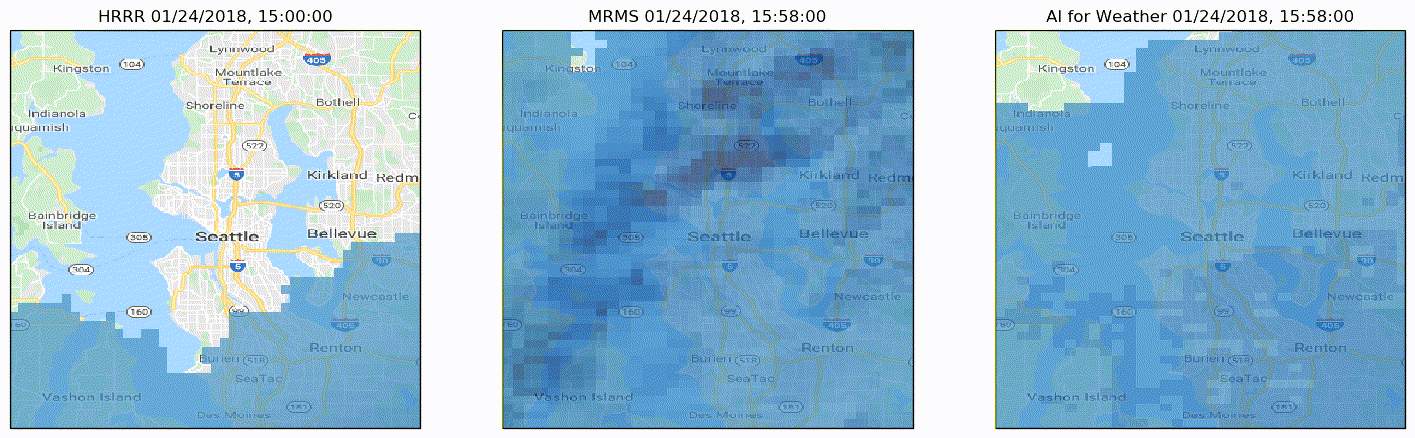
According to researchers, weather forecasting over short time ranges or also called “nowcasting” can be actually tackled using machine learning in a completely data-driven approach. In the paper, they argue that physics weather models are heavy and computationally expensive, therefore limiting the power of prediction in several ways. One of them is the typical problem of resolution in weather models, where the limits demand up to 5 kilometers which is not useful for many use cases.
To show that weather (or more precisely precipitation) forecasting can be done in a data-driven way without any physics knowledge, researchers proposed a method that learns directly from a sequence of radar images. Using convolutional neural networks, researchers designed an architecture that takes multispectral satellite images as input (as a sequence of observations) and provides output prediction, again in image format for the next several hours.
Evaluations show that the model outperforms traditional models such as HRRR (High-Resolution Rapid Refresh). More results and details about the method can be read in the paper or in the official blog post from Google AI.