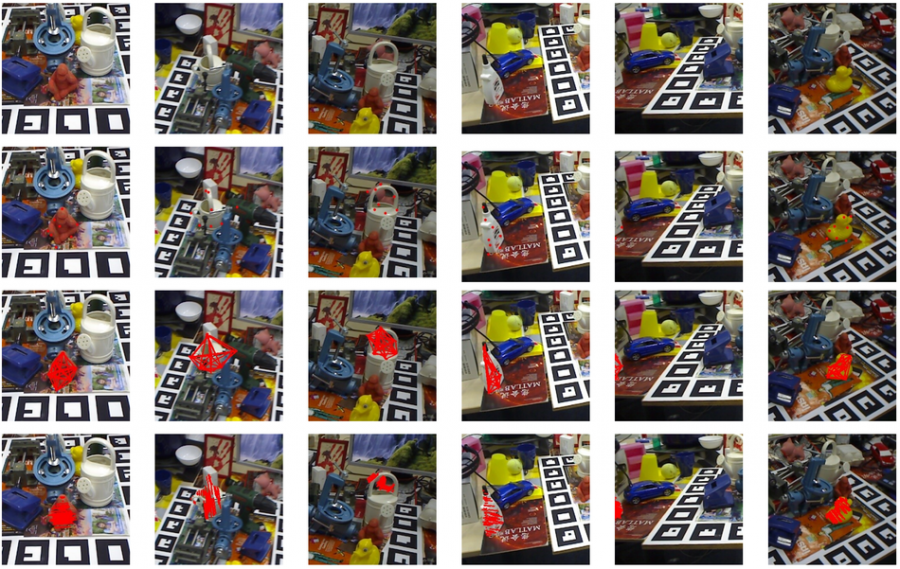
Group of researchers from the University of Texas, Austin has developed a novel 6D pose estimation method that significantly outperforms existing methods.
Their new approach, called HybridPose, predicts an intermediate representation which is used to obtain the final 6D position of an object. It does so by incorporating two modules: a neural network that encodes the input into the intermediate representation and a pose regression module that extracts the pose from this representation. The task of the regression module is to refine the predictions and remove potential outliers in the intermediate representation.
The output of the first network is in fact a combination of predicted keypoints on the object, edge vectors, and symmetry correspondences. According to researchers, they wanted to achieve robustness in their representation by including more information than merely 2D keypoints, which are often subject to inaccurate predictions. The proposed method leverages multiple representations that express the necessary geometric information for pose estimation. The diagram below shows the architecture of the proposed method.
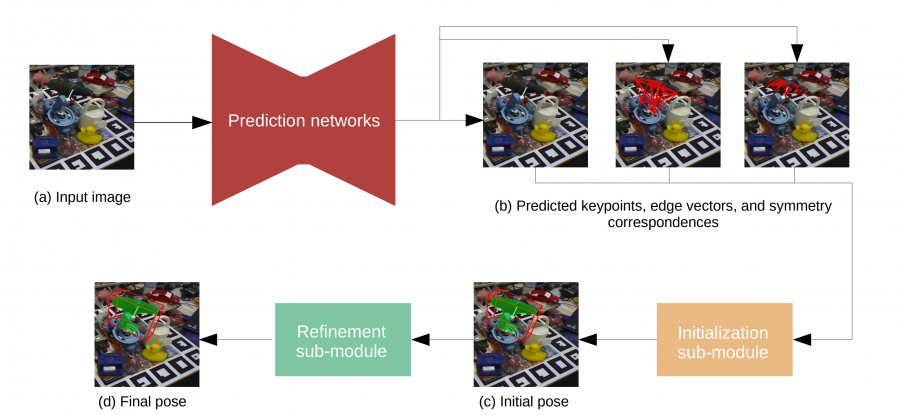
Researchers evaluated the method on popular benchmark datasets such as Linemod and Occlusion Linemod. They report that HybridPose achieves an accuracy of 79.2% significantly outperforming the current state-of-the-art approach by more than 67%.
The implementation of the method was open-sourced and can be found on Github. The paper was published on arxiv.