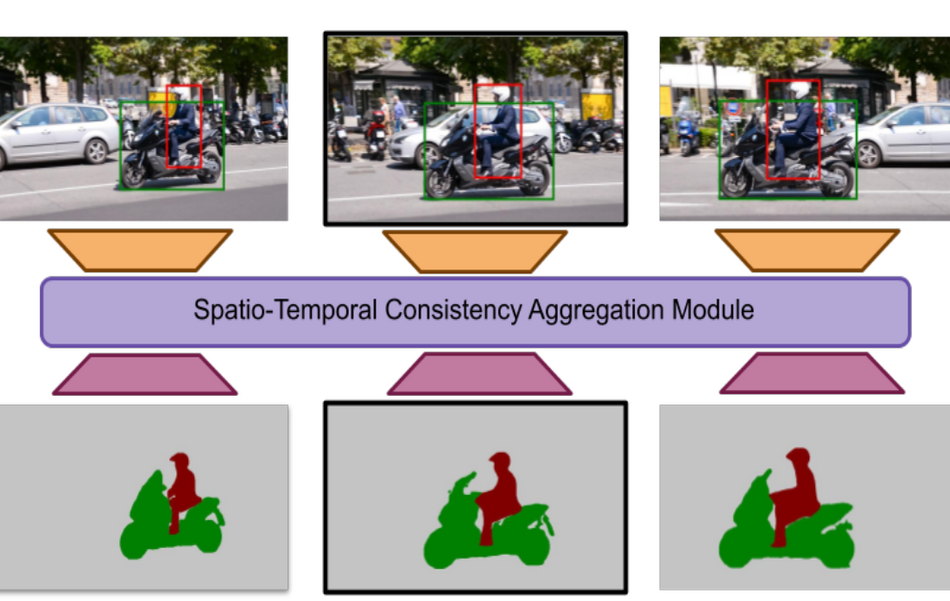
A group of researchers from ETH University has proposed a novel method for object semantic segmentation that exploits video data and spatio-temporal consistencies.
Arguing that current approaches for object segmentation in images rely on data-hungry solutions and heavy models, researchers propose to use bounding box annotations from object detectors in a framework that filters foreground objects by enforcing spatio-temporal consistency. Their approach is based on extracting deep features per-object and per-frame in a short video clip that are aggregated and decoded into segmentation masks. The approach takes a series of frames from a video and extracts deep features for each frame. Then object detection bounding boxes are used to feed object local information into an object decoder that generates a representation for the specific object. These features are then fused and provided to a module called Spatio-Temporal Aggregation module that decodes this information to obtain a sequence of segmentation masks. The architecture of the approach is shown in the image below.
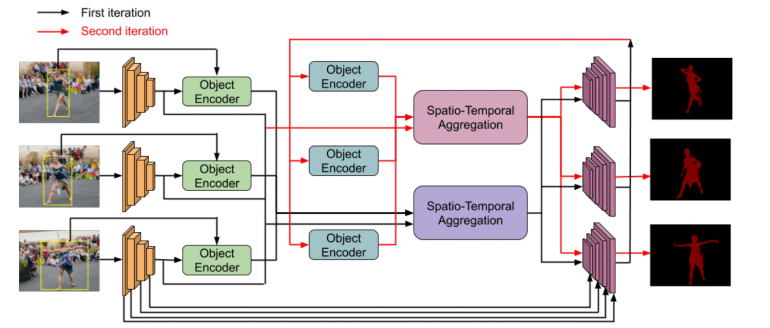
The resulting model was trained in an end-to-end manner using existing labeled video datasets. More specifically, researchers used YouTube-VOS and DAVIS-2017 datasets with a ResNet backbone in their model to conduct a series of experiments. Results from these experiments showed that the model is able to learn successfully how to segment objects in videos and verified the effectiveness of this weakly supervised approach.
Researchers open-sourced the implementation of the proposed approach and it is available on Github. More details about the method, the training parameters and the experiments and ablation studies can be read in the paper published on arxiv.