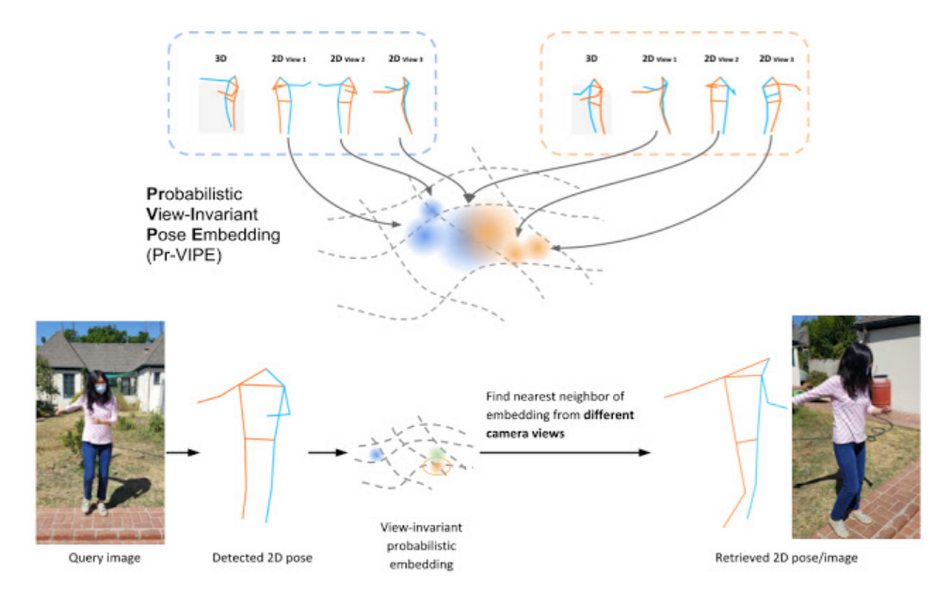
Researchers from Google AI have presented a new method for recognizing poses and pose similarity in images and videos.
Images and videos contain 2D information about some portion of the 3D world captured with some camera. For example an image of a person provides a single view of that person and two different cameras could provide two completely different images. In order to be able to recognize a specific 3D pose from different images capturing the pose from different angles, that requires learning a view-invariant representation.
In their paper, researchers from Google propose to learn such representation or embedding space using a probabilistic model trained on a set of 2D keypoints. Their approach was based on the fact that a direct 2D-keypoint-to-3D mapping would not be sufficient to capture view invariance and ambiguity. The model that researchers proposed was called Pr-VIPE – View-invariant Probabilistic Embedding for Human Pose. It takes as an input a set of 2D keypoints (that can come from any source) and it outputs mean and variance of the pose embedding i.e. a sample from a Gaussian distribution.
The proposed model was trained using combined data from: existing multi-view image datasets on one hand and 2D projections of 3D poses on the other. The 2D poses are mapped to a probabilistic embedding i.e. a multivariate Gaussian distribution and a pose similarity score is computed as the similarity score between distributions.
In order to evaluate the proposed method, pose retrieval was used as the benchmark task. The idea is that if the model learned a meaningful embedding space of poses, it would be able to successfully retrieve a query pose given as a monocular image.
Results from the experiments showed that the method achieves higher accuracy on pose retrieval tasks than direct mapping methods. Additionally, researchers conducted some experiments on action recognition and video alignment, where the proposed method proved effective too.
More details about the method and the experiments can be read in the official blog post or in the paper published on arxiv.