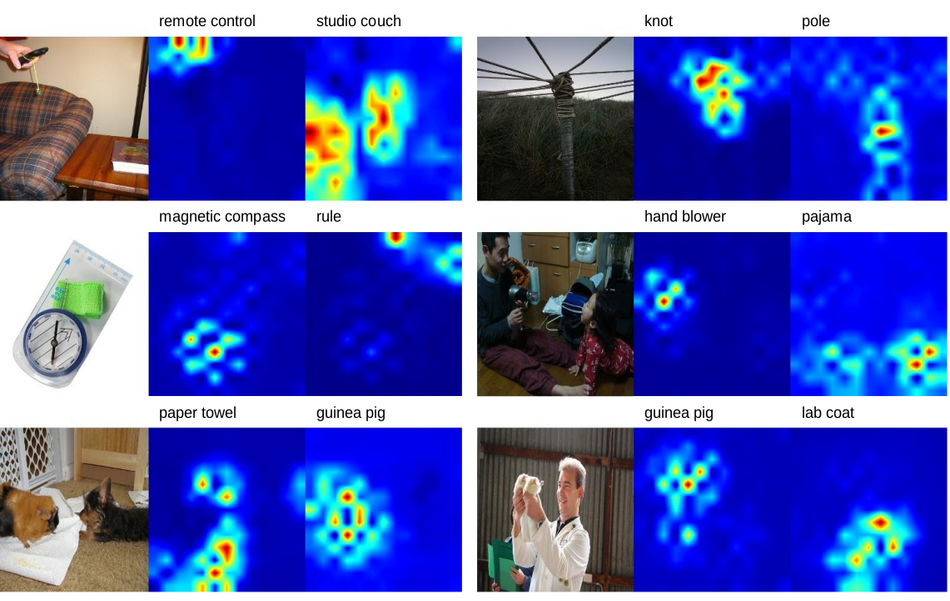
A large portion of the research experiments in Computer Vision in the past decade were using the ImageNet dataset. Over the years it has become the default and standard benchmark for advances in the fields of machine learning and vision.
In a recent paper, researchers from NAVER AI Lab argue that ImageNet as an image dataset contains a significant amount of label noise. Also several prior studies have confirmed this hypothesis. Based on this reason, researchers set to re-label the whole ImageNet dataset and introduce multi-labels instead of single labels. According to them single labels introduce a lot of noise in the training data and that’s the case especially when there are random crops applied to the training images.
In order to introduce multi-labels, researchers used a much stronger pre-trained state-of-the-art classifier that can precisely annotate the images and produce high-quality dense labels. The model that they used was pre-trained on large-scale image datasets such as JFT-300M and InstagramNet-1B. In addition to the introduction of multi-labels, researchers wanted to have more localized labels, instead the ImageNet’s global ones. To achieve this, they modified the classifier network and turned it into a fully-convolutional network (FCN) that could produce location-wise predictions. The image below shows an example of such annotations.
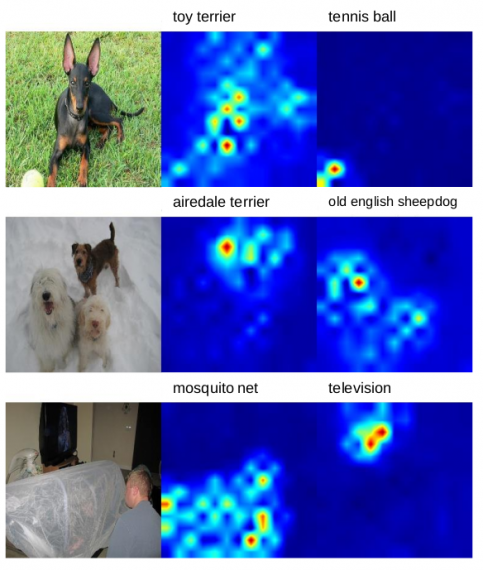
of top-2 class (right).
After re-labeling the entire ImageNet dataset, researchers conducted a series of experiments to evaluate the performance of various models trained on this dataset. They also conducted experiments that showcase the transfer-learning performance. The results from these experiments showed that a ResNet-50 model trained on Re-label ImageNet can achieve a top-1 accuracy of 78.9% that can be further boosted to 80.2%. Similar improvement was noticed in transfer learning to object detection and instance segmentation tasks.
The new dataset called Re-label ImageNet together with the source code was open-sourced and can be found here. The paper was published on arxiv.