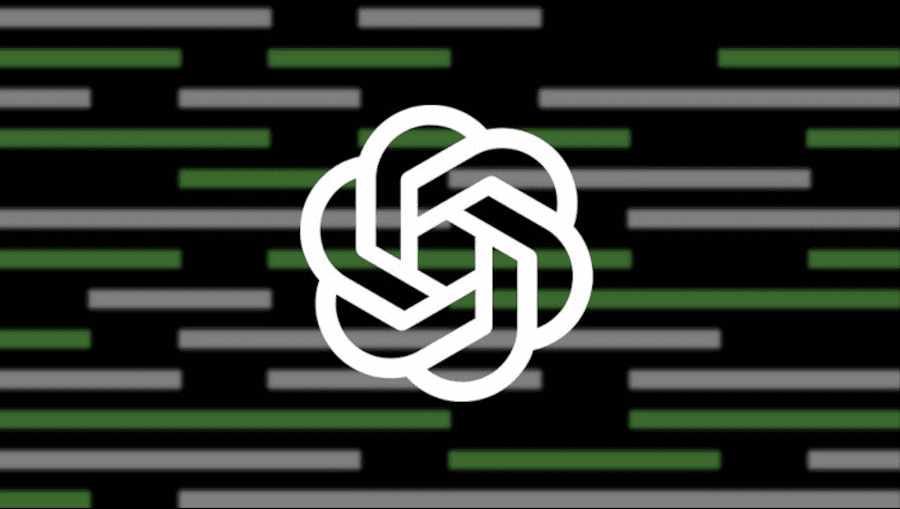
OpenAI has introduced a significant update to its GPT-3.5 Turbo model, allowing developers to fine-tune the model for their specific tasks and applications. This enhancement opens up the opportunity for developers to fully harness the capabilities of GPT-3.5 Turbo. Now, developers have the ability to perform subtle adjustments (finetuning) to the model’s parameters, enhancing its performance and accuracy within their respective domains. Initial tests indicate that the finely-tuned GPT-3.5 Turbo aligns with or even surpasses the baseline metrics of GPT-4 for specific tasks.
Applications of GPT-3.5 Turbo Fine-Tuning
Fine-tuning enhances the model’s capability to consistently format responses—a crucial aspect for applications that demand specific response formats. Examples include code completion, generating API calls, or transforming user queries into JSON format. When crafting marketing materials, the model can be trained to adopt text formatting and tone of voice that align with the brand. Additionally, the model can be trained to respond in a specific language, ensuring that clients always receive answers in the language of the question posed, without explicitly specifying it. By default, GPT-3.5 responds in English.
For more detailed insights into the capabilities of model fine-tuning, refer to the OpenAI documentation.
Enhancing Performance
Initial tests reveal that fine-tuning enables companies to reduce the length of requests while maintaining comparable efficiency. Testers managed to condense request sizes by 90% by embedding instructions directly into the model. This accelerates each API call and reduces costs. The fine-tuned models can process contexts of up to 4,000 tokens, doubling the previous capacity. This enhancement further accelerates calls and reduces API usage expenses.
Support for fine-tuning, along with function calls and gpt-3.5-turbo-16k, will be available in the autumn.
Cost
The cost of fine-tuning includes both training and model usage. For instance, fine-tuning on a dataset of 100,000 tokens with 3 epochs will amount to approximately $2.4.