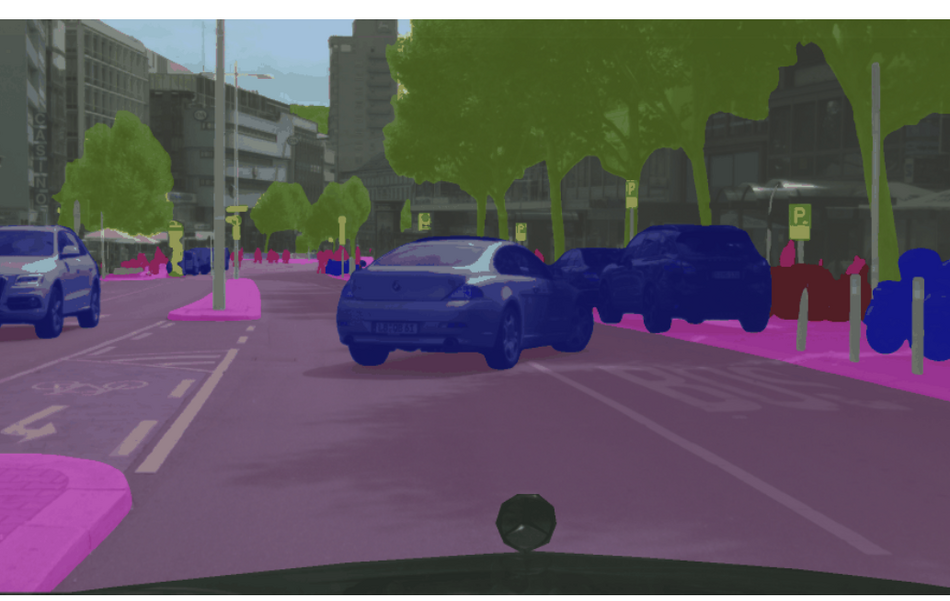
Researchers from Baidu Inc. have announced the release of a novel image segmentation toolkit called PaddleSeg.
The development kit was built in order to help researchers and engineers working in the field of image segmentation, achieve faster progress and seamless industry deployment. It also serves as a central point for image segmentation research, featuring a number of prepared models and datasets used in this area.
PaddleSeg was developed in a modular fashion taking into account the computational performance of the models. It’s core features include: high-quality efficient pre-trained models, modular code design and training efficiency. According to the developers, users of PaddleSeg can easily build over hundred different models using backbones, full networks and modular components present in the toolkit. All these models are highly efficient as their implementation is better than their open-source variants.
These are some of the models readily available in the Model Zoo of PaddleSeg: DeepLabv3, PSPNet, FastSCNN, UNet++, HardNet, GCNet etc. From the popular datasets the toolkit includes: Cityscapes, PascalVOC and ADE20K as of now. Besides the higher efficiency that the models within PaddleSeg exhibit, researchers report that for many of the open-source models a better accuracy is achieved when trained using the toolkit.
The toolkit implementation was open-sourced and can be found on Github. Also, a number of tutorials on how to get started, how to prepare data and use components from PaddleSeg are available in Baidu’s AI Studio. More details on the development, the architecture and components of the toolkit can be read in the paper published in arxiv.