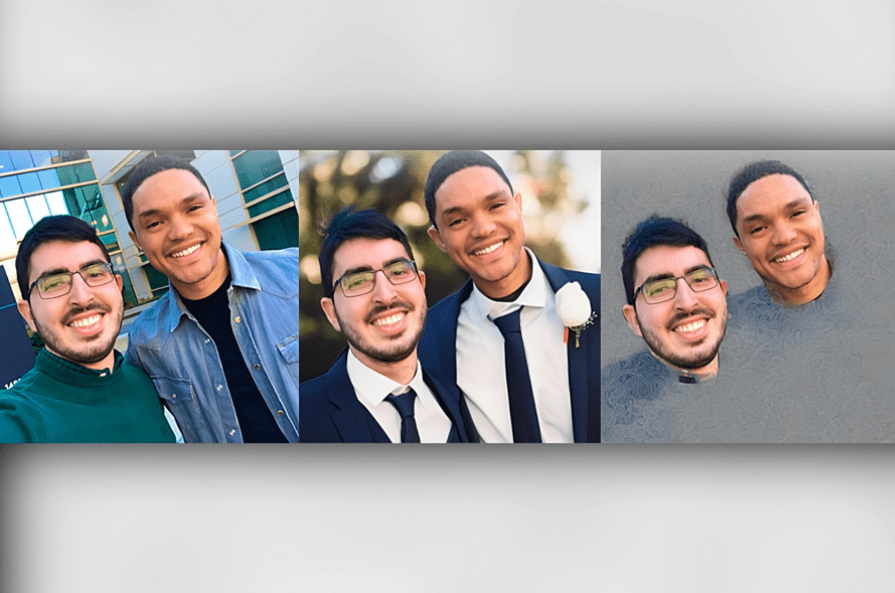
Researchers at MIT have introduced PhotoGuard, an algorithm designed to safeguard images from unauthorized alterations by generative models, ensuring the authenticity of images.
The widespread use of generative models such as DALL-E and Midjourney has made it easy for even ordinary users to create high-quality images and modify them using natural language text queries. Malevolent actors can exploit these models for potential reputation, financial, and legal risks associated with falsifications.
PhotoGuard addresses this issue by introducing perturbations — minor pixel changes that are imperceptible to the human eye but affect the model’s output significantly. These perturbations effectively disrupt the model’s ability to manipulate images.
PhotoGuard employs two distinct strategies to introduce perturbations. In the simpler strategy, referred to as “encoding,” pixel alterations affect the hidden representation of the image, causing the generative model to perceive the image as random noise. As a result, any attempt to manipulate the image using models becomes nearly impossible. The introduced changes are so subtle that they remain unnoticed by the human eye, preserving the visual integrity of the image while simultaneously ensuring protection against editing.
The second strategy, known as “diffusion,” shapes perturbations in a way that makes the image appear similar to an entirely different (“target”) image, distinct from what the human eye perceives. Consequently, when modified, the model makes changes to the “target” image, thereby safeguarding the original image from falsification.
Currently, “diffusion” requires significantly greater computational resources than “encoding.” However, scientists believe that they can optimize this strategy in the future.