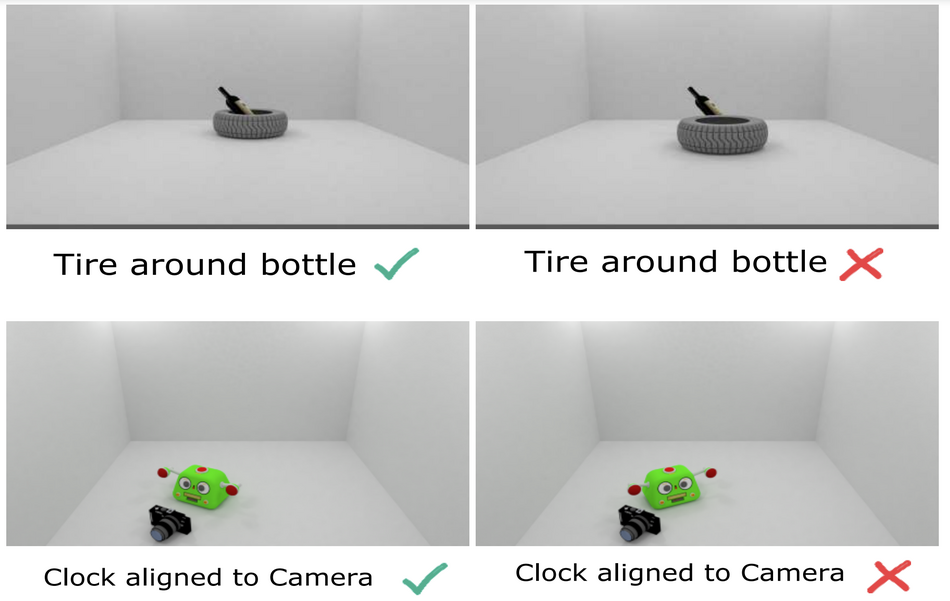
Rel3D – A novel large-scale benchmark for spatial relations in 3D was recently released by researchers from the University of Michigan and Princeton University.
The new benchmark tries to overcome issues that existing 3D relations benchmarks have, such as limited scale, limited variety, or lack of human-annotated data. Additionally, researchers introduced an important feature of this benchmark called minimally contrastive pairs. This means that the benchmark contains even two identical scenes with the only difference being the spatial relation, which holds in one and doesn’t in another scene.
The data that is part of Rel3D was collected in a two-stage process using humans as annotators. Each sample or scene in the dataset contains two objects: subject and object that may or may not satisfy a spatial relation. The final dataset that researchers collected consists of 9990 scenes and 27336 images containing varied objects from 67 categories.
Researchers conducted a number of experiments to validate the efficiency and data quality of their novel benchmark. They used Rel-3D to benchmark popular and state-of-the-art methods in visual relationship detection models. The interesting results that researchers shared in the paper suggest that all state-of-the-art models fail to outperform the simple 2D baseline, while humans achieve a score of 94%. According to them, this is a strong confirmation of the quality of the benchmark and it can be a good asset for model evaluation and detecting issues in spatial relationship models.
Instructions on how to use the benchmark are available in the Github repository.