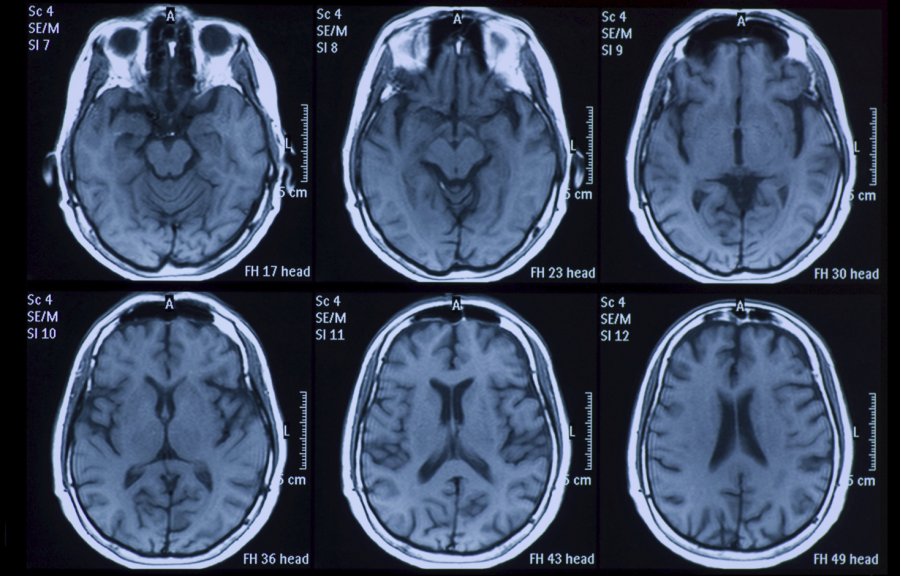
Researchers from Facebook AI Research (FAIR), the University of Florida and NYU School of Medicine have proposed a neural network model that reduces uncertainty in MRI scans reconstruction.
MRI reconstruction is, in essence, an ill-posed inverse problem where a high-fidelity MRI image has to be reconstructed using partially observed measurements from MRI scanner. Due to the nature of the problem, a large amount of uncertainty is present in the process of MRI reconstruction.
To tackle this problem, researchers proposed a novel reconstruction method based on deep neural networks. The proposed method works in a way that it dynamically selects which measurements are the best to observe with the objective of minimizing the uncertainty in the reconstruction.
Opposite of many general approaches for MRI reconstruction that work with a fixed set of measurements, the novel method acquires k-space measurements (kMA) at inference time and refines the reconstruction at each step while reducing the error and therefore the uncertainty.
The researchers proposed a network architecture comprised of two modules which are trained jointly.
“..we introduce a novel neural network-based system composed of a reconstruction network and an evaluator network, which are trained jointly to minimize the reconstruction error while maximizing the acquisition speed.“, say Adriana Romero, Michal Drozdzal, and Nafissa Yakubova, researchers at FAIR.
The first module which is the reconstruction network is responsible for generating the MRI reconstruction and providing the uncertainty measure. On the other hand, the evaluator network has to rate all of the unobserved k-space measurements kMA of a reconstruction. The evaluator network is, in fact, learning how to guide the measurement selection.
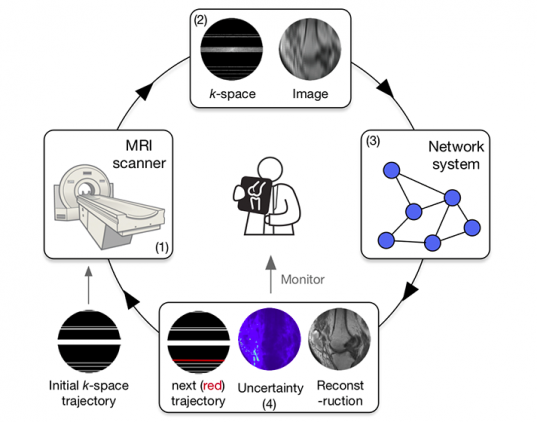
Researchers show that the method outperforms active acquisition baseline methods, and it is able to produce high-fidelity reconstructions. More about the proposed method can be found in the paper, which was accepted as a conference paper at CVPR 19.