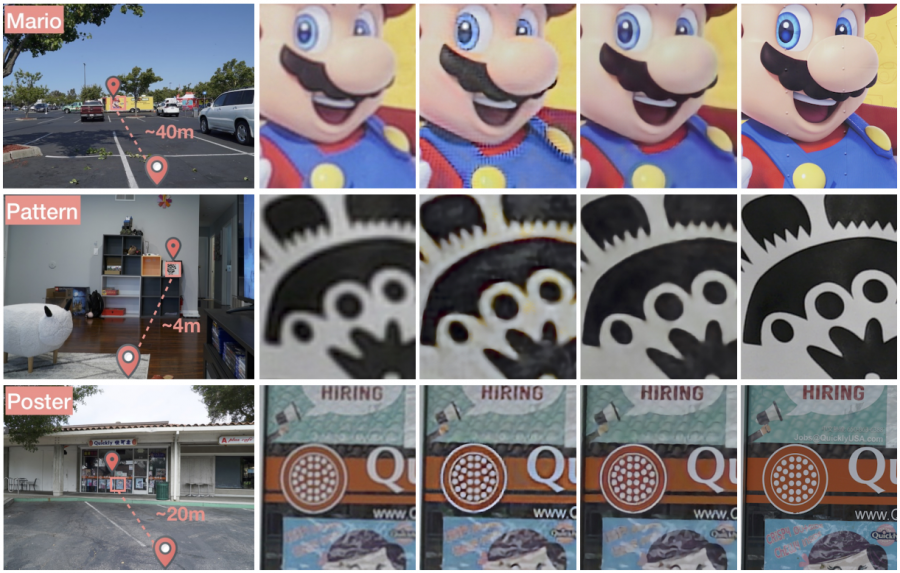
In a novel paper, researchers from UC Berkeley, HKUST and Intel Labs propose a deep learning based method that improves 4X computational zoom.
In the past, many researchers have focused their work on improving image quality for distant objects when using a zoom functionality. In general purpose solutions, it can often be seen that the zoom functionality is provided as a simple upsampling of a region of the image, therefore resulting in a poor quality image.
Recently, super-resolution methods i.e. methods that upscale and improve details in image regions have employed deep neural networks, which are able to learn from large-scale datasets. In order to improve these methods, researchers propose to apply machine learning methods directly on raw sensor data instead of processed images.
They argue that details can be recovered easier if working with (unprocessed) sensor data instead of RGB images and propose a deep learning method that improves zoom capabilities by four times over traditional methods.
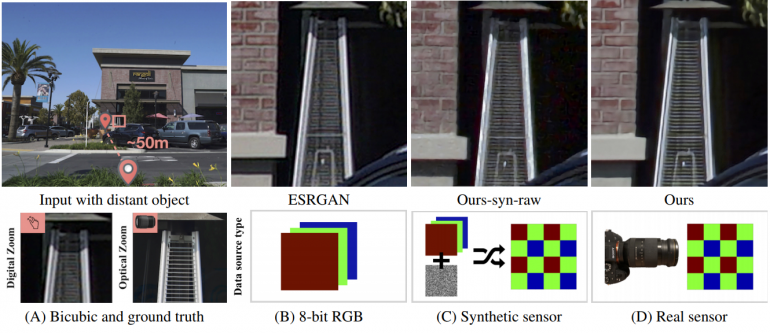
Since the idea was to apply a deep learning super-resolution method, researchers also created a dataset for a real-world computational zoom, called SR-RAW. They used this dataset to train a deep neural network model, introducing also a novel loss function.
They performed an extensive evaluation of the method, comparing it to a few baseline methods such as SRGAN, GAN-based SR, SRResnet, LapSRN, ESRGAN, PIRM. The evaluation showed that the proposed method overperforms other methods in 4X and 8X computational zoom.