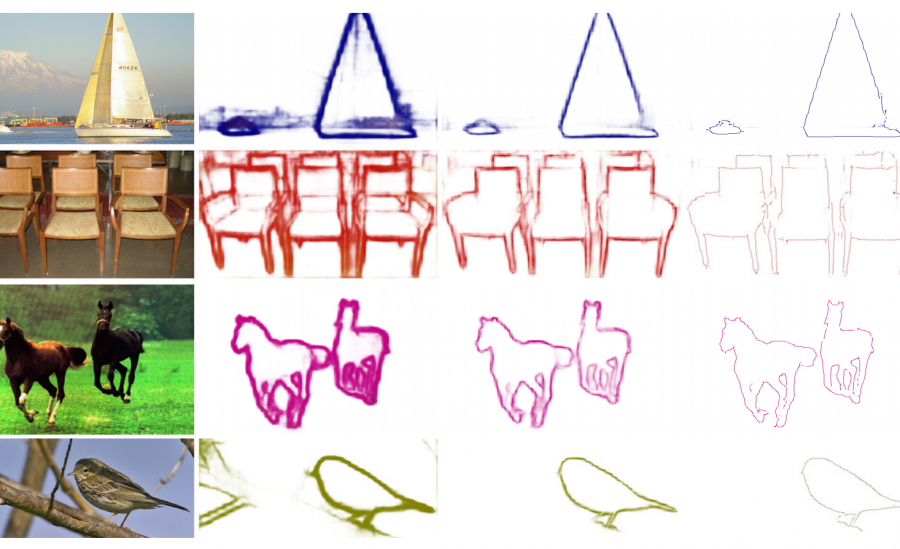
Researchers from NVIDIA have proposed a novel state-of-the-art method for learning semantic boundaries of objects in images. In their novel paper, “Devil is in the Edges: Learning Semantic Boundaries from Noisy Annotations”, they propose a new neural network architecture and loss function that learns do detect precise semantic boundaries.
Semantic segmentation is one of the main tasks in computer vision and in the past few years has been tackled via deep neural networks. Usually, the goal of semantic segmentation is to assign a class label to each pixel so that each object will be identified and therefore “segmented” in the image.
A slightly different task is semantic boundary prediction where the goal is to detect the boundaries of semantic objects. The key problem is the difficulty of extracting or labeling the precise boundaries of the objects.
In order to learn sharp and precise object boundaries, researchers propose an approach named STEAL: “Semantically Thinned Edge Alignment Learning (STEAL) “. The novel method consists of a new boundary thinning layer and a loss function that enforces the output of more precise semantic boundaries (edges). STEAL can be plugged on any existing semantic boundary prediction network in order to improve the obtained results.
The experiments that the researchers performed showed that the proposed method outperforms all of the current state-of-the-art methods. The implementation of the method can be open-sourced and can be found on the project’s page. More in detail, about the proposed method, can be read in the official paper.