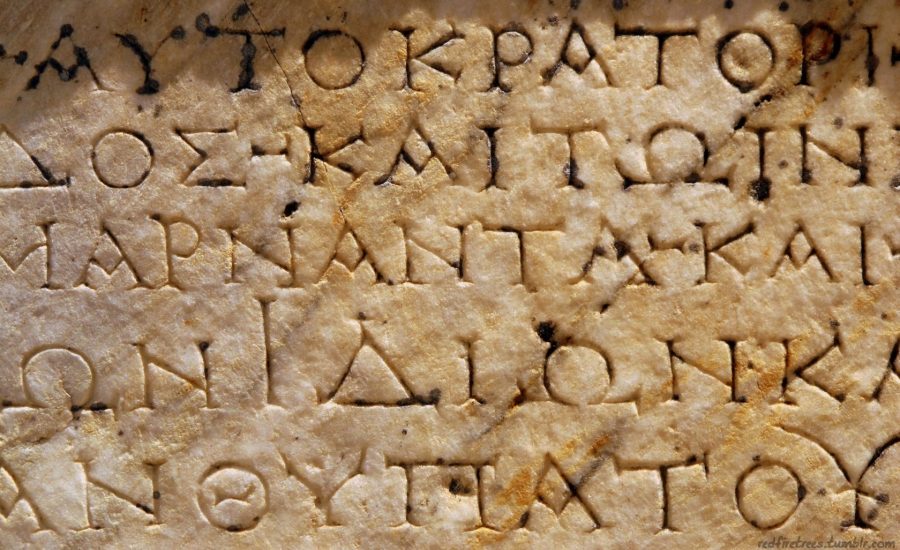
A group of researchers from CSAIL at MIT and Google Brain used machine learning to automatically translate lost languages. The team developed a neural network-based system that can decipher texts from long lost languages.
In their paper, named “Neural Decipherment via Minimum-Cost Flow: from Ugaritic to Linear B”, researchers explain how they tackle this challenging problem using deep learning. The proposed method is a bit different than existing methods for neural machine translation of languages. This is due to the fact that in the case of ancient lost languages there is a lack of data for supervision.
To overcome this issue, researchers exploit the assumption that languages are known to evolve over time. Therefore, they were able to build an expressive sequence-to-sequence model that is trained to model character-level relations between cognates.
The proposed approach leverages 4 defined properties which are guiding the deciphering process: distributional similarity (matching characters provides context match), monotonic character mapping (character alignment should be preserved), structural sparsity (cognates match mostly one-to-one) and significant cognate overlap (assuming that there will be sufficient overlap within related languages).
Researchers developed a generative framework based on these principles. They also introduce a novel unsupervised training procedure to train the proposed model. The final model was evaluated on the decipherment of Linear B and Ugaritic language where it overperforms existing state-of-the-art methods by 5.5%.
More details about the proposed method can be read in the pre-print paper.