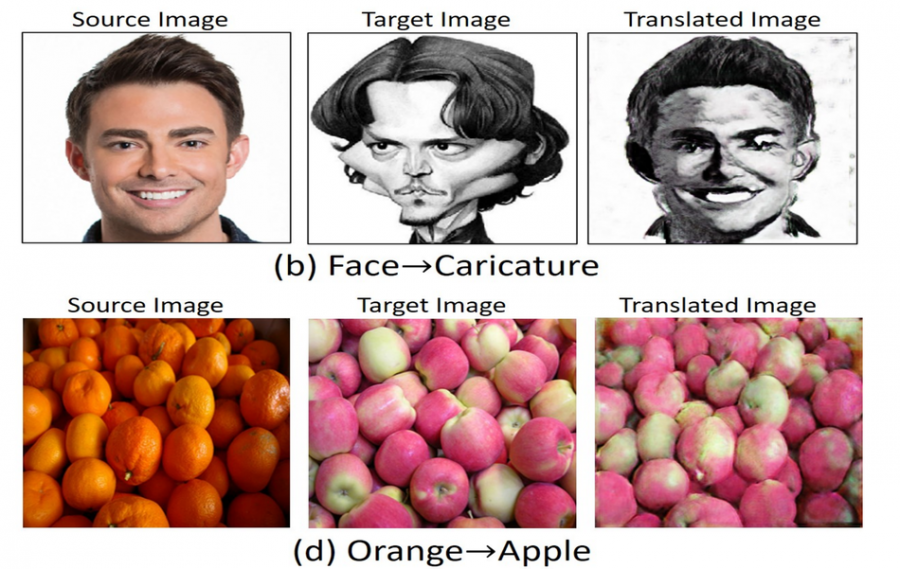
Researchers from the University of Science and Technology of China, Microsoft and the University of Rochester have presented TuiGAN – a novel GAN model for image-to-image translation trained using only two unpaired images.
In their paper, named “TuiGAN: Learning Versatile Image-to-Image Translation with Two Unpaired Images”, researchers propose an unsupervised method for image-to-image translation based on the GAN architecture. The idea was to develop a model that will be able to inherit the domain-invariant features of the source image and replace the domain-specific features from the target one. In order to do so, researchers designed a scale-to-scale framework for learning these features from only a single pair of images.
The architecture of the proposed TuiGAN model consists of two symmetric pyramids of generators and discriminators. The generators take the source input image downscaled and prepared at different scales together with previously translated image and generate a new “translated” image. On the other hand, the discriminators learn the domain distribution by narrowing down the receptive fields using the downscaling in the framework.
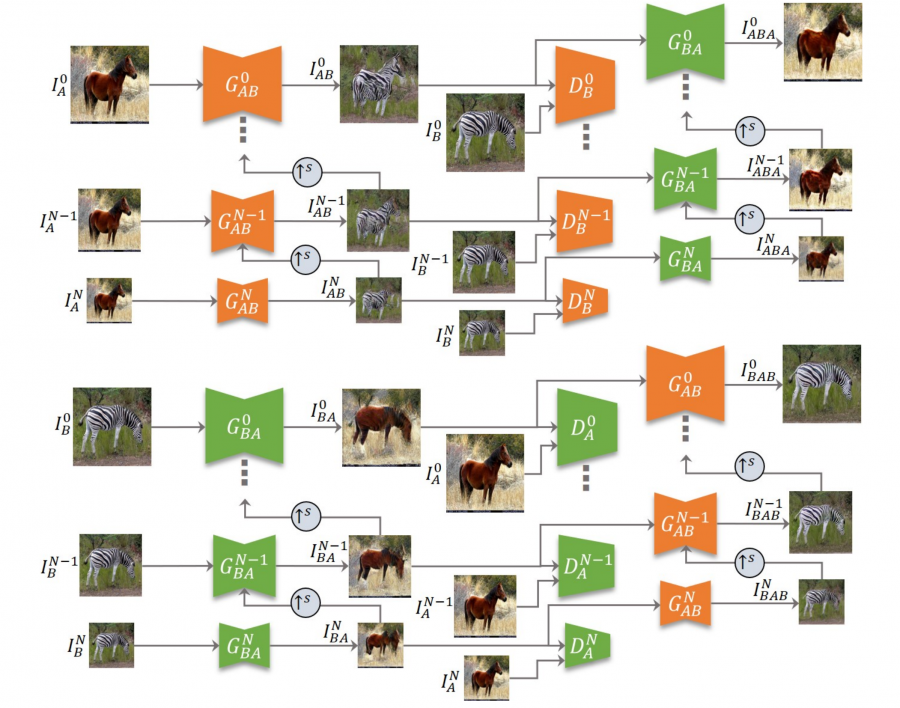
They show that the proposed framework can deal with image-to-image translation problems and provides satisfactory results. The method was evaluated on several of these problems such as image style transfer, animal face translation, paint-to-image translation, etc.
In order to prove the effectiveness of TuiGAN, the model was compared to strong baselines in the image-to-image translation domain: CycleGAN, DRIT, SinGAN, ArtStyle and others. Researchers used “Single Image Fr´echet Inception Distance” SIFID as one of the evaluation metrics and showed that TuiGAN achieves best SIFID score among all defined baselines. Some outputs from the method can be seen below.
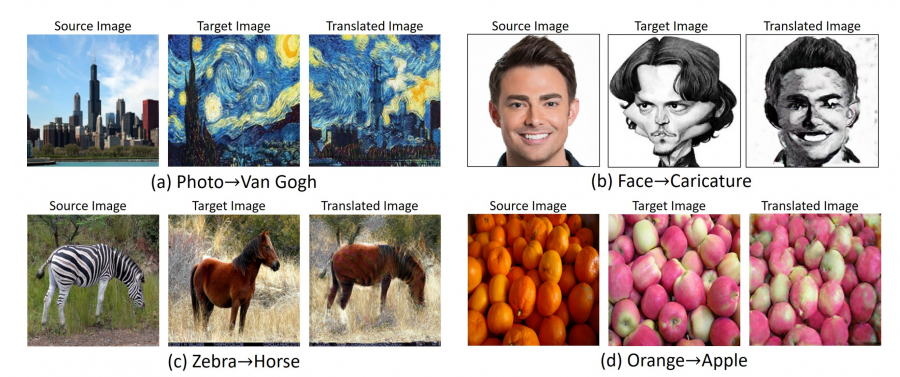
The implementation of TuiGAN was open-sourced and can be found here. More details about the model and the experiments can be read in the paper.