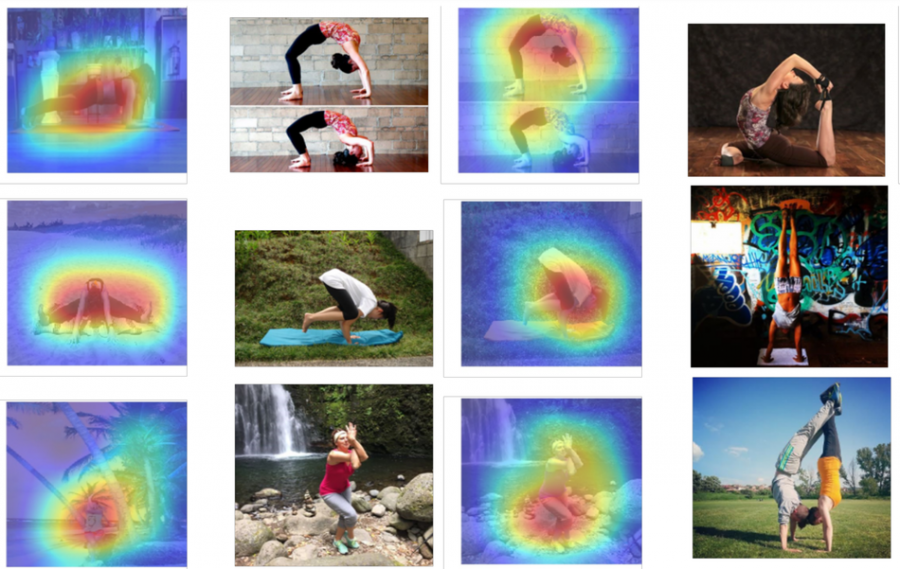
A group of researchers from Osaka University and IIT Gandhinagar have released a new dataset of diverse yoga poses for pose estimation.
The limitations in terms of pose variety that existing pose estimation datasets exhibit, have served as a motivation for researchers to delve deeper and propose a new concept of fine-grained hierarchical pose classification along with a diverse dataset of 82 complex poses. In the paper, the researchers argue that fine annotations may not be an option for pose estimation datasets that contain a large variety of poses, which are usually difficult to describe and label in an exclusive manner.
To overcome the problem of fine annotations for the newly created dataset, they introduce a three-level hierarchy that defines body positions and variations. The six main categories among which all other poses are divided are standing, sitting, balancing, inverted, reclining, and wheel. Each of these categories contains several subgroups that contain the actual poses. The dataset contains more than 28.4 K yoga pose images along with class annotations from the defined class hierarchy. The images were collected from the Internet and the taxonomy was collected from various sources such as books, websites etc.
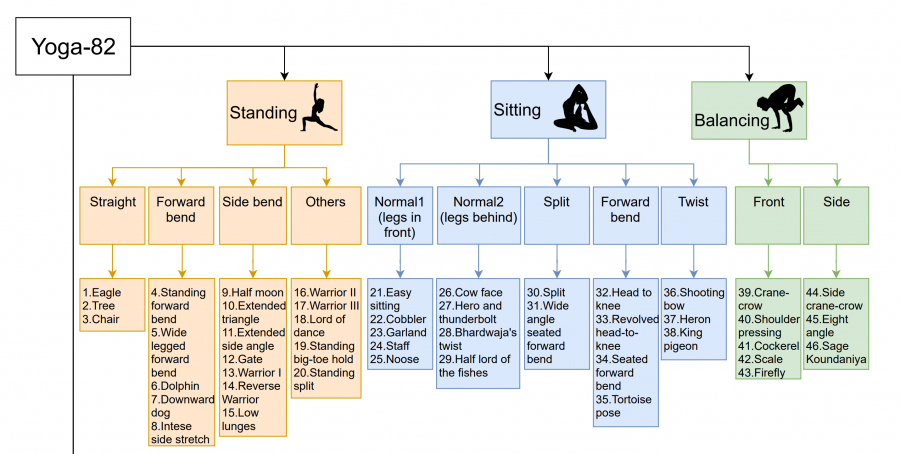
To evaluate the performance of the novel dataset, researchers trained several popular CNN models: ResNet, DenseNet, MobileNet, etc. In order to utilize the hierarchical structure of the Yoga-82 dataset, researchers propose several modifications to the DenseNet model which consider the three-level classification defined in the dataset. In fact, they propose three variants of the model which take this into account in a slightly different manner.
According to the results, the best-performing model on the novel pose dataset is DenseNet, which performed better than models with sparse connections such as ResNet or MobileNet. The dataset will be available soon. More in detail about the data collection and the experiments can be read in the paper.