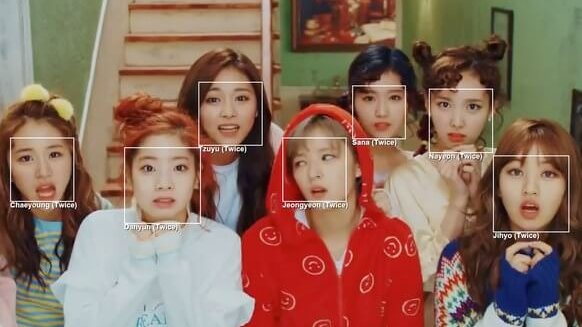
Is it correct to ask such a question at all? The latest study showed that racial differences have to be taken into account by developers of neural networks to improve the accuracy of face recognition. But in society, it is formally considered that all people are equal and bias in this matter should be avoided.
Gfycat software engineer Gurney Gan said that last summer his software successfully identified most of his colleagues, but “stumbled” on one group.
“It got some of our Asian employees mixed up. Which was strange because it got everyone else correctly”, — says Gan.
However, even the largest companies have similar problems. For example, Microsoft and IBM face analysis services are 95 % more accurate when recognizing white men than women with darker skin.
The Google Photos service does not respond to requests “gorilla”, “chimpanzee” or “monkey”. Thus, the risk of repetition of the embarrassment of 2015 is eliminated. The search engine back then mistakenly took black people in photos for monkeys.
A universal standard for testing and eliminating bias in AI systems has not been developed yet.
“Lots of companies are now taking these things seriously, but the playbook for how to fix them is still being written”, — says Meredith Whittaker, co-director of AI Now.
Gfycat started face recognition development to create a system that would allow people to find the perfect GIFs to use in messengers. The search system of the company works with about 50 million GIF-files — from cats to presidents’ faces. With the help of face recognition, developers wanted to facilitate the search for famous personalities — from politicians to stars
“Asian detector”
The company used open source software based on Microsoft research and trained it on millions of photos from collections issued by the Universities of Illinois and Oxford.
But the neural network could not distinguish Asian celebrities, such as Constance Wu and Lucy Liu, and did not distinguish reliably people with dark skin.
First, Gan tried to solve the problem by adding more photos of “problematic” faces to machine learning examples. But adding a large number of photos of black and Asian celebrities to the data array helped only partially.
It was possible to solve the problem only by creating a sort of “Asian detector”. Finding an Asian face, the system goes into a mode of hypersensitivity.
According to Ghana, this was the only way to make the program distinguish Asians from each other.
The company says that the system is now 98% accurate when identifying white people, and 93 % when dealing with Asians.
Intentional search for racial differences may seem a strange way to combat prejudice. But this idea was supported by a number of scientists and companies. In December, Google published a report on improving the accuracy of the smile recognition system. This was achieved by determining whether the person in the photo is a man or a woman, and to which of the four racial groups he belongs. But the document also says that artificial intelligence systems should not be used to determine the person’s race and that using only two gender and four racial categories is not sufficient in all cases.
Some researchers have suggested forming industry standards for transparency and decreasing AI bias. Thus, Eric Learned-Miller, professor of the University of Massachusetts, suggests that organizations utilizing face recognition, such as Facebook and the FBI, should disclose the accuracy of their systems for different gender and racial groups.