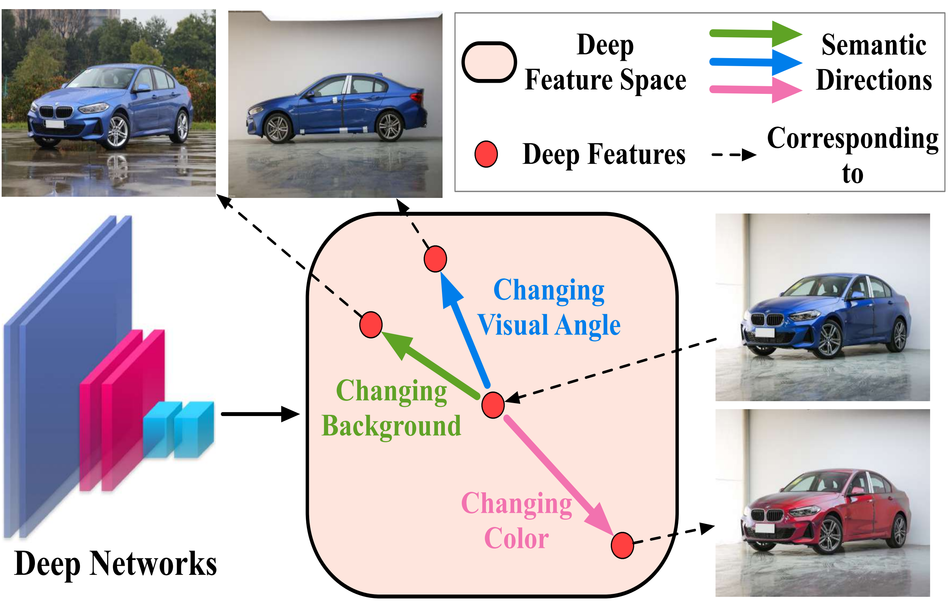
A group of researchers from the University of Beijing has proposed a novel implicit semantic data augmentation method that improves the generalization capabilities of deep neural networks.
Data augmentation has proved as a very efficient strategy for regularizing deep neural network models and has allowed training very large models (with billions of parameters) with smaller amounts of data. However, more advanced augmentations such as semantic transformations are usually difficult to obtain automatically and require manual effort meaning it is an expensive and time-consuming process.
In their recent paper, researchers propose a way to overcome the problem of automatic generation of semantic augmentations. Their method named ISDA – Implicit Semantic Data Augmentation, is relying on the observation that deep neural network models are learning linearized features, which means that directions in the latent space are actually (semantically) meaningful. Using this knowledge about this property of deep networks, researchers design a method which first identifies the meaningful directions in the feature space and introduces a modified cross-entropy loss function that uses estimates for covariance matrices of deep features of each class. According to them, this is an effective way to achieve the needed trade-off between increasing the capacity (through a large number of augmentations) and efficiency (in terms of processing).
The final method introduces very minimal computational overhead over regular neural network training and improves generalization capabilities significantly. Experiments and evaluations were done on several popular datasets including CIFAR-10, CIFAR-100, ImageNET, MS-COCO, Cityscapes, SVHN, etc.
The implementation of the new data augmentation technique was open-sourced and it is available on Github. The paper can be found here.
__
Content-Based Image Retrieval (CBIR) technology enables users to search for images based on visual features such as color, texture, and shape rather than relying solely on textual descriptions. By analyzing the visual content of images, CBIR systems can efficiently retrieve relevant images from large databases. These systems are widely used in various applications, including image search engines, medical image analysis, and digital asset management. To experience the power of CBIR firsthand, you can try out this image search service.