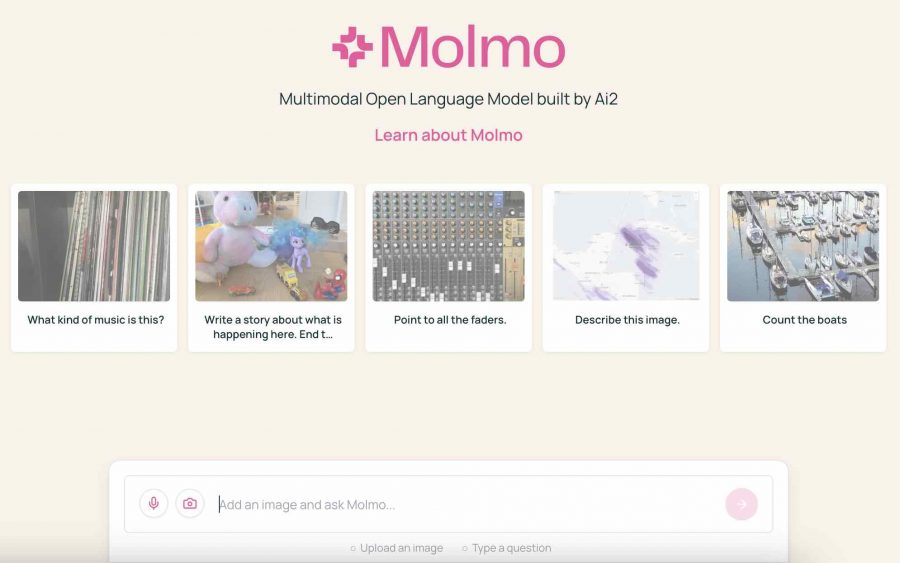
Molmo is a new series of multimodal vision-language models (VLMs) created by researchers at the Allen Institute for AI and the University of Washington. The Molmo family outperforms many state-of-the-art proprietary and open-source models on both academic benchmarks and human evaluations, making it suitable for a wide range of vision-language tasks.
The researchers have released four models:
- MolmoE-1B, based on the OLMoE-1B-7B mixture-of-experts LLM
- Molmo-7B-O, using the fully open OLMo-7B-1024 LLM.5
- Molmo-7B-D, using the open-weight Qwen2 7B LLM
- Molmo-72B, the best-performing model, using the open-weight Qwen2 72B LLM
These models code and weights are available on Huggingface. Dataset access can be requested with this form.
This development demonstrates that open-source models can achieve state-of-the-art performance in vision-language tasks without relying on proprietary systems or synthetic data, potentially democratizing access to advanced AI capabilities and accelerating progress in the field. Molmo addresses key challenges in open-source VLM development, including model performance, data quality, and reproducibility without reliance on proprietary systems.
Molmo Performance Highlights
- MolmoE-1B nearly matches GPT-4V on academic benchmarks and human preference evaluations
- Molmo-7B models perform between GPT-4V and GPT-4o
- Best-in-class Molmo-72B achieves the highest academic benchmark score (81.2% average) and ranks second in human preference, just behind GPT-4o,
- and outperforms proprietary systems like Gemini 1.5 Pro, Gemini 1.5 Flash, and Claude 3.5 Sonnet
Multimodal Vision-Language Architecture
Molmo uses a simple and standard architecture combining a pre-processor for multi-scale, multi-crop image processing, a ViT image encoder (OpenAI’s ViT-L/14 336px CLIP), a connector MLP for vision-language projection and pooling, and a decoder-only Transformer language model with various options.
Dataset
The key innovation behind Molmo’s success is the PixMo-Cap dataset, a novel collection of highly detailed image captions gathered from human speech-based descriptions. This dataset comprises 712,000 images with approximately 1.3 million captions. The researchers employed a simple two-stage training approach: caption generation pre-training followed by supervised fine-tuning on a diverse mixture of datasets.
Molmo incorporates a novel 2D pointing capability, allowing it to respond to queries using both textual answers and visual indicators. This feature enables the model to interact with images more intuitively, pointing to specific areas to support its responses or to answer spatial questions directly.
Results
The Molmo family of models demonstrates impressive performance across various benchmarks and evaluations. MolmoE-1B, the most efficient model, nearly matches GPT-4V on both academic benchmarks and human preference evaluations. The Molmo-7B models (7B-O and 7B-D) perform comfortably between GPT-4V and GPT-4o. The flagship Molmo-72B achieves the highest average score (81.2%) across 11 academic benchmarks and ranks second in human preference evaluations, just behind GPT-4o.
Notably, Molmo outperforms several state-of-the-art proprietary systems, including Gemini 1.5 Pro, Gemini 1.5 Flash, and Claude 3.5 Sonnet. In extensive testing, Molmo was evaluated on 11 academic benchmarks and underwent a comprehensive human evaluation involving 15,000 image-text prompt pairs, with over 325,000 pairwise preference ratings from approximately 870 human annotators.
AI agents
How to Use New Multimodal Vision-Language Models
The Molmo team is committed to open science, with plans for a full release of model weights, training data, and code. Their first release includes a demo, inference code, and select model weights, is available now. This approach promotes reproducibility and advancement of open VLM research.
“Molmo demonstrates that it’s possible to build state-of-the-art vision-language models using only open data and training techniques,” the researchers state in their paper. Their innovative approach of using speech-based image descriptions and diverse fine-tuning data offers a new paradigm for developing high-quality, open-source multimodal models without relying on proprietary systems or synthetic data.
The success of Molmo paves the way for future developments in open-source VLMs, potentially revolutionizing applications in areas such as image understanding, visual question answering, and multimodal reasoning.