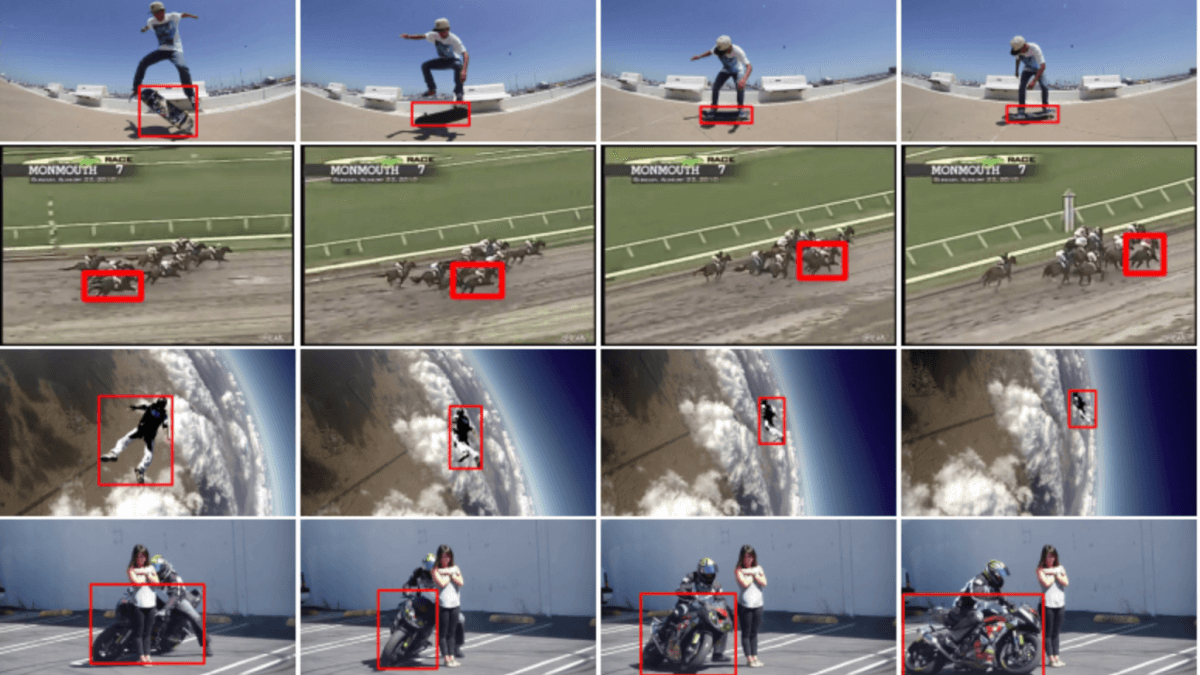
Object tracking in the wild is far from being solved. Existing object trackers do quite a good job on the established datasets (e.g., VOT, OTB), but these datasets are relatively small and do not fully represent the challenges of real-life tracking tasks. Deep learning is at the core of the most state-of-the-art trackers today. However, a dedicated large-scale dataset to train deep trackers is still lacking.
In this article, we discuss three recently introduced datasets for object tracking. They differ in scale, annotations and other characteristics but all of them can contribute something to solving object tracking problem: TrackingNet is a first large-scale dataset for object tracking in the wild, MOT17 is a benchmark for multiple object tracking, and Need for Speed is the first higher frame rate video dataset.
TrackingNet
Number of videos: 30,132 (train) + 511 (test)
Number of annotations: 14,205,677 (train) + 225,589 (test)
Year: 2018
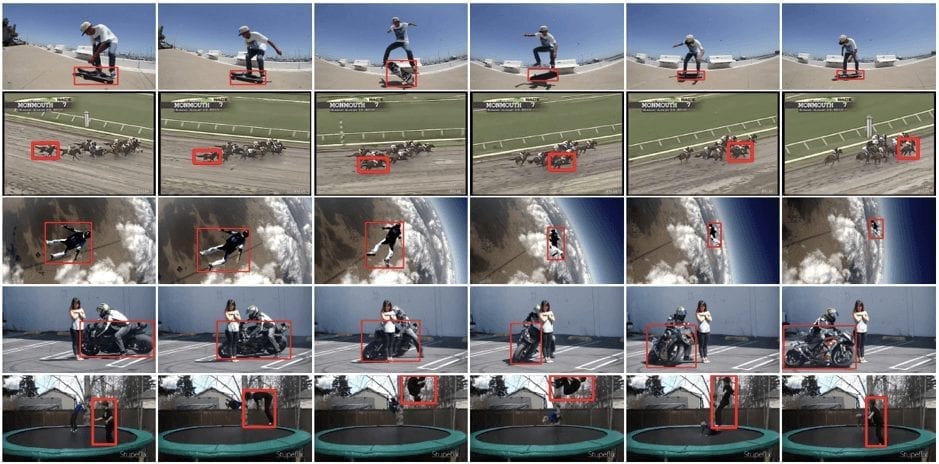
TrackingNet is a first large-scale dataset for object tracking in the wild. It includes over 30K videos with an average duration of 16.6s and more than 14M dense bounding box annotations. The dataset is not limiting to a specific context but instead covers a wide selection of object classes in a broad and diverse context. TrackingNet has a number of notable advantages:
- a large scale of this dataset enables the development of deep design specific for tracking;
- by being specifically created for object tracking, the dataset enables model architectures to focus on the temporal context between the consecutive frames;
- the dataset was sampled from YouTube videos and thus, represents real-world scenarios and contains a large variety of frame rates, resolutions, context and object classes.
TrackingNet training set was derived from the YouTube-Bounding Boxes (YT-BB), a large-scale dataset for object detection with roughly 300K video segments, annotated every second with upright bounding boxes. To build TrackingNet, the researchers filtered out 90% of the videos by selecting the videos that a) are longer than 15 seconds; b) include bounding boxes that cover less than 50% of the frame; c) contain a reasonable amount of motion between bounding boxes.
To increase annotation density from 1fps provided by YT-BB, the creators of TrackingNet rely on a mixture of state-of-the-art trackers. They claim that any tracker is reliable on a short interval of 1 second. So, they have densely annotated 30,132 videos using a weighted average between a forward and a backward pass using the DCF tracker. Furthermore, the code for automatically downloading videos from YouTube and extracting the annotated frames is also available.
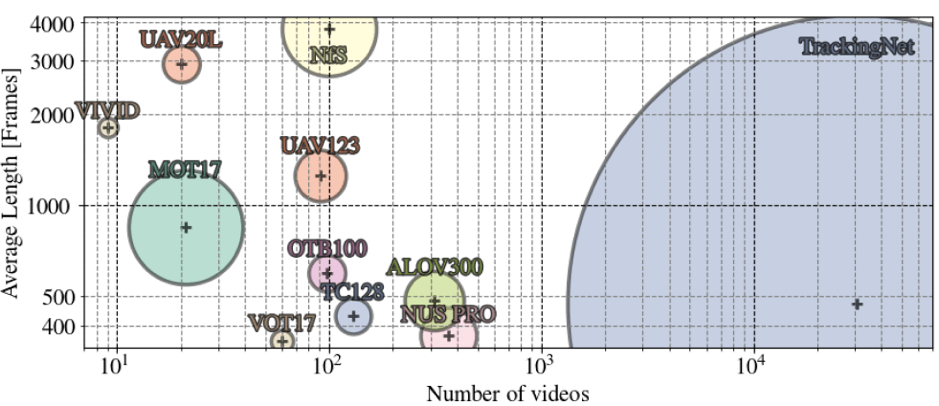
Finally, TrackingNet dataset comes with a new benchmark composed of 511 novel videos from YouTube with Creative Commons license, namely YT-CC. These videos have the same object class distribution as the training set and are annotated with the help of Amazon Mechanical Turk workers. With the tight supervision in the loop, TrackingNet team has ensured the quality of the annotations after a few iterations, discouraged bad annotators and incentivized the good ones.
Thus, by sequestering the annotation of the test set and maintaining an online evaluation server, the researchers behind TrackingNet provide a fair benchmark for the development of object trackers.
MOT17
Number of videos: 21 (train) + 21 (test)
Number of annotations: 564,228
Year: 2017

MOT17 (Multiple Object Tracking) is an extended version of the MOT16 dataset with new and more accurate ground truth. As evident from its name, the specific focus of this dataset is on multi-target tracking. It should be also noted that the context of MOTChallenge datasets, including this last MOT17 dataset, is limited to the street scenes.
The new MOT17 benchmark includes a set of 42 sequences with crowded scenarios, camera motions, and weather conditions. The annotations for all sequences have been carried out by qualified researchers from scratch following a strict protocol. Even more, to ensure the highest annotations accuracy, all the annotations were double-checked. Another thing that distinguishes this dataset from the earlier versions of MOTChallenge datasets is that here not only pedestrians are annotated, but also vehicles, sitting people, occluding objects, as well as other significant object classes.
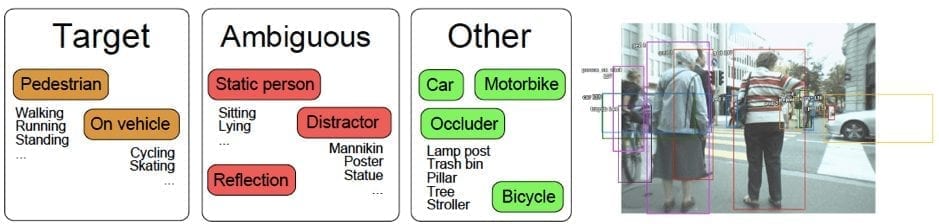
The researchers have defined some classes as the target ones – they are depicted with orange in the above image; these classes are the central ones to evaluate on. The red classes include ambiguous cases such that both recovering and missing them will not be penalized in the evaluation. Finally, the classes in green are annotated for training purposes and for computing the occlusion level of all pedestrians.
An exemplar of an annotated frame demonstrates how partially cropped objects are also marked outside of the frame. Also, note that the bounding box encloses the entire person but not the white bag of the pedestrian.
Rich ground truth information provided within the MOT17 dataset can be very useful for developing more accurate tracking methods and advancing the field further.
NfS
Number of videos: 100
Number of annotations: 383,000
Year: 2017
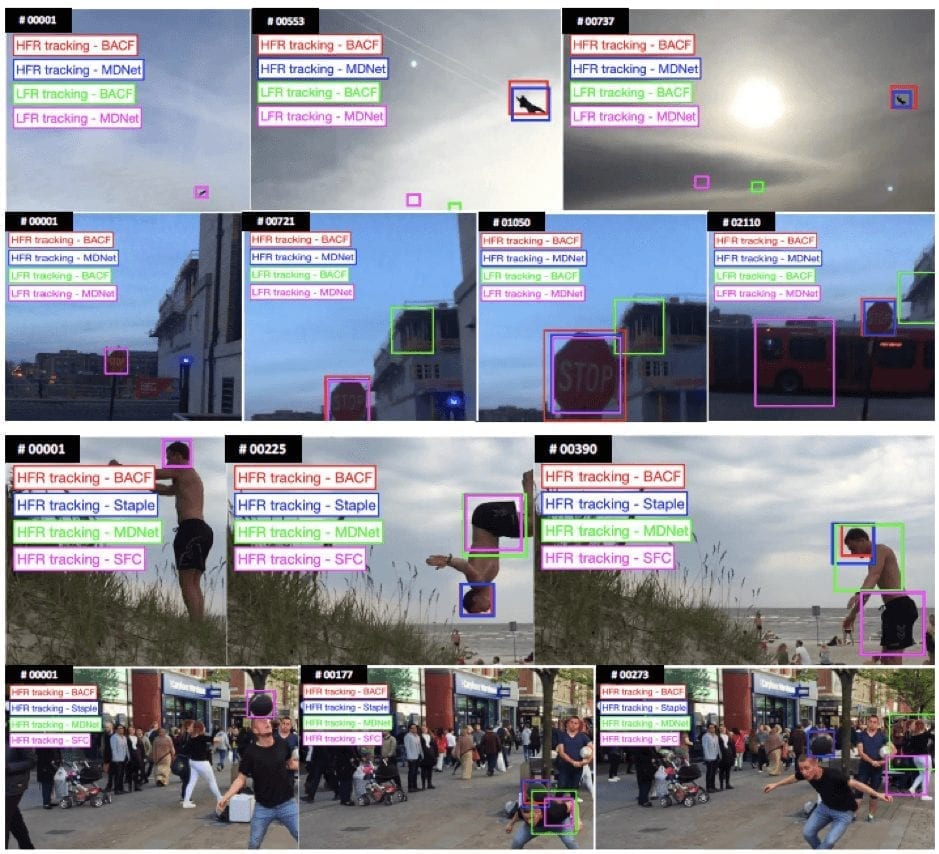
NfS (Need for Speed) is the first higher frame rate video dataset and benchmark for visual object tracking. It includes 100 videos comprised out of 380K frames and captured with 240 FPS cameras, which are now often used in real-world scenarios.
Particularly, 75 videos were captured using the iPhone 6 (and above) and the iPad Pro, while 25 videos were taken from YouTube. The tracking targets include vehicles, humans, faces, animals, aircraft, boats and generic objects such as sport balls, cups, bags etc.
All frames in NfS dataset are annotated with axis-aligned bounding boxes using the VATIC toolbox. Moreover, all videos are manually labeled with nine visual attributes: occlusion, illumination variation, scale variation, object deformation, fast motion, viewpoint change, out of view, background clutter, and low resolution.
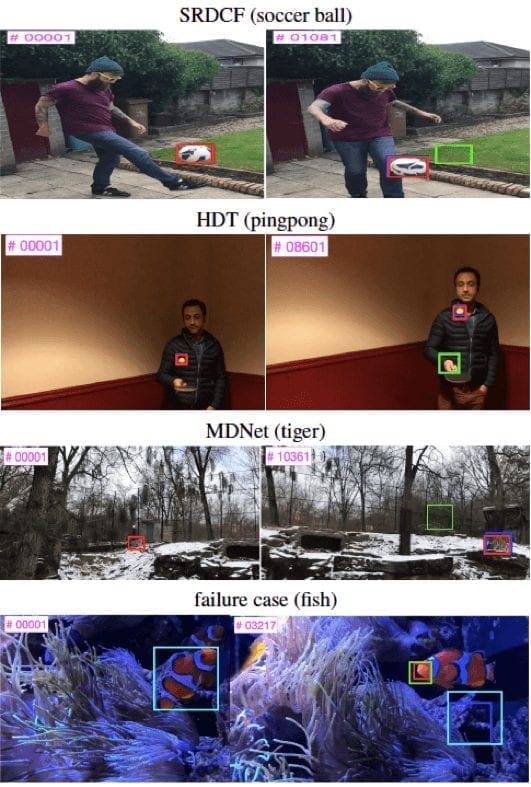
NfS benchmark provides a great opportunity to evaluate state-of-the-art trackers on higher frame rate sequences. Actually, some surprising results were already revealed thanks to this dataset: apparently, at higher frame rates, simple trackers such as correlation filters outperform complex deep learning algorithms.
Bottom Line
The scarcity of the dedicated large-scale tracking datasets leads to the situation when object trackers based on the deep learning algorithms are forced to rely on the object detection datasets instead of the dedicated object tracking ones. Of course, this limits advances in object tracking field. Fortunately, the object tracking datasets introduced recently, especially the large-scale TrackingNet dataset, provide data-hungry trackers with the great opportunities for significant performance upgrades.