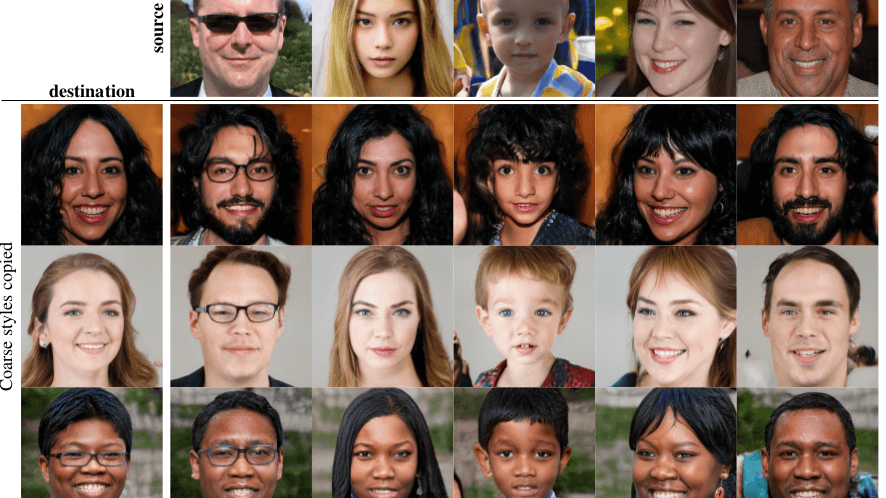
NVIDIA Research has just released a new paper introducing a groundbreaking generator architecture called StyleGAN. This innovative method is making waves in the image synthesis community due to its superior interpolation properties and the ability to better disentangle the latent factors of variation. By automatically separating high-level attributes such as face pose and identity, from stochastic variations like freckles and hair, the StyleGAN architecture creates stunningly realistic images.
While Generative Adversarial Networks (GANs) have enjoyed a great deal of success in terms of image quality, the image synthesis process remains elusive, and efforts to understand internal representations of GANs have been ongoing. However, StyleGAN is a game-changer that adjusts the image style at each convolution layer based on the latent code, allowing for greater control over image features at different scales.
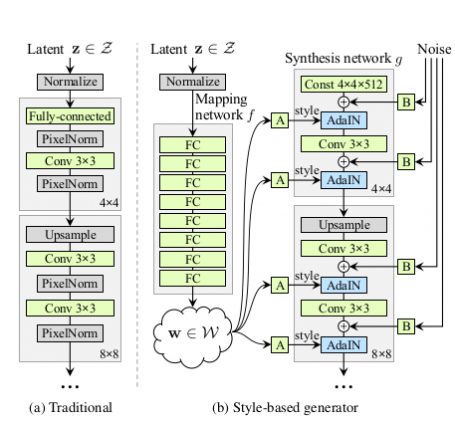
Unlike traditional Generator networks, StyleGAN maps the latent vector to an intermediate space, which is then fed into the synthesis network along with some noise. This approach has yielded excellent results, with the image quality staying good and even improving compared to ordinary GANs. The research team evaluated their findings using the CELEBA-HQ and FFHQ datasets, which demonstrate the power and potential of StyleGAN.
The paper is available on ArXiv, and a video explaining the method is also available. Stay tuned as the source code is expected to be published soon. With StyleGAN, the future of image synthesis is looking brighter than ever.
The researchers show that the generated image quality stays sufficiently good and even improved compared to ordinary GAN. Evaluation has been done using the famous CELEBA-HQ dataset and a new dataset called FFHQ.
The paper is published on ArXiv, and a video explaining the method is available here. The source code is expected to be published soon.