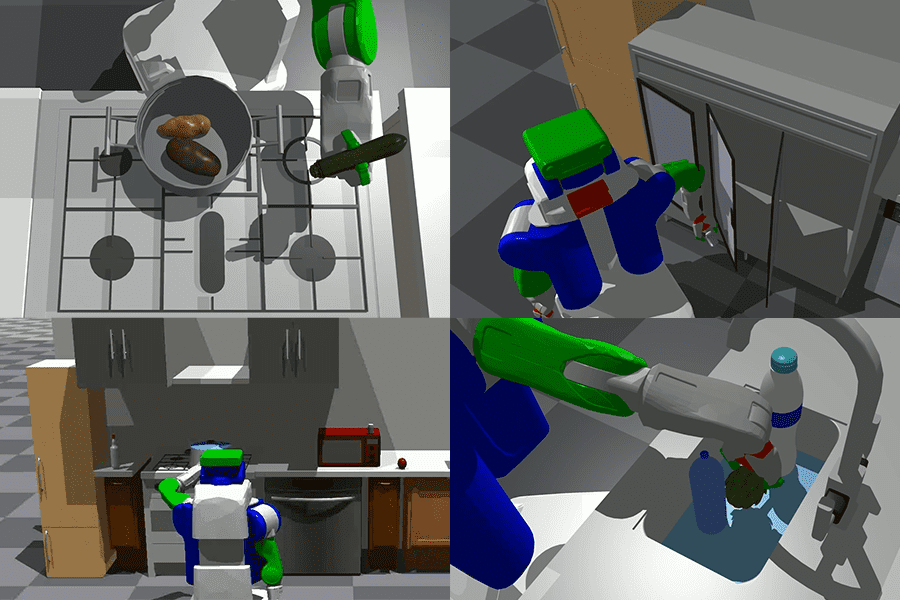
MIT researchers have introduced PIGINet, a neural network designed to teach robots how to navigate through various tasks. PIGINet evaluates potential action sequences based on task descriptions, scene images, and current states, selecting the most optimal sequence. The aim of PIGINet is to expedite the resolution of everyday tasks performed by robots by pre-determining the correct action plan, thereby reducing the occurrence of errors.
Despite robots acquiring fundamental skills through training in controlled environments, there exist numerous incorrect actions they might undertake when attempting a task. For example, in the process of making coffee, a robot might mistakenly turn on a faucet, drain water into the sink, clean a flour container, and so forth. However, there are only a few actions that lead to the desired outcome. PIGINet streamlines the iterative task planning process by assessing the likelihood of successful task execution for each potential scenario and eliminating improbable options.
A distinctive feature of the neural network, as highlighted by the researchers, is the multimodality of input data. This encompasses scene images, current status, and the assigned task, collectively enabling the robot to grasp intricate geometric relationships more comprehensively.
The model was trained on a relatively modest dataset of around 500 tasks compared to other algorithms. The research team synthesized hundreds of virtual environments, each distinct in layout and specific tasks, such as rearranging items between tables, refrigerators, cabinets, sinks, and cooking pots. By measuring the time taken to solve tasks, they compared PIGINet against previous approaches. The neural network reduced task planning and robot movement time by 50-80%. The most significant time reduction was observed in tasks closely resembling training scenarios and involving a small number of actions.