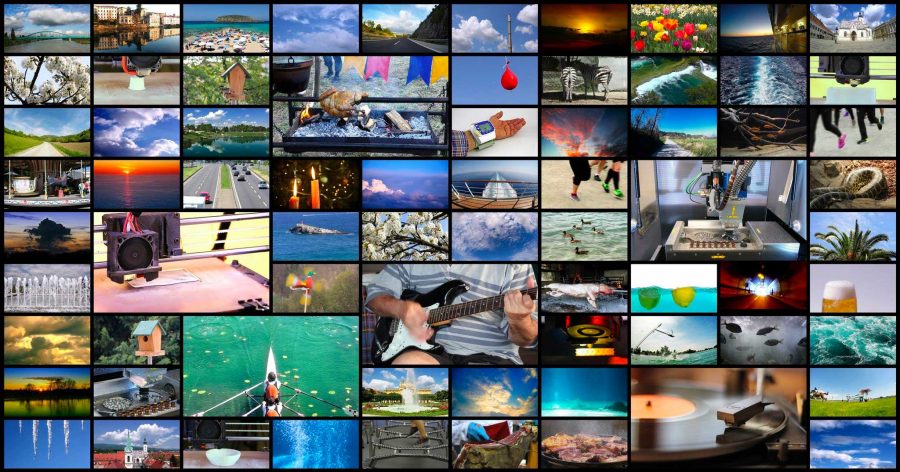
SEER is FAIR’s self-supervised billion-parameter neural network for computer vision applications. The model pre-trained on the Instagram pictures can be further trained on your tasks. The developers have published the VISSL library for training the SEER model.
More about model architecture
SEER combines RegNet architecture and online self-supervised learning format. SwAV was used as an algorithm for online learning. RegNet, in turn, is a scalable convolutional neural network that circumvents training and memory constraints. This combination allows SEER to scale to billions of parameter and training images.
Testing SEER performance
After pre-training on a billion random, untagged Instagram images, SEER has bypassed most state-of-the-art self-supervised models. According to the results of the experiments, the maximum prediction accuracy of the neural network was 84.2% on the ImageNet dataset.
SEER has also bypassed state-of-the-art supervised learning approaches on tasks such as low-shot, object detection, image segmentation and classification.s
Using 10% of data from ImageNet to train, the maximum SEER accuracy is 77.9% for the entire ImageNet. If you train the neural network on 1% of labeled images from ImageNet, the accuracy will be 60.5%.
The SEER results show that the self-supervised learning format is also suitable for computer vision tasks.