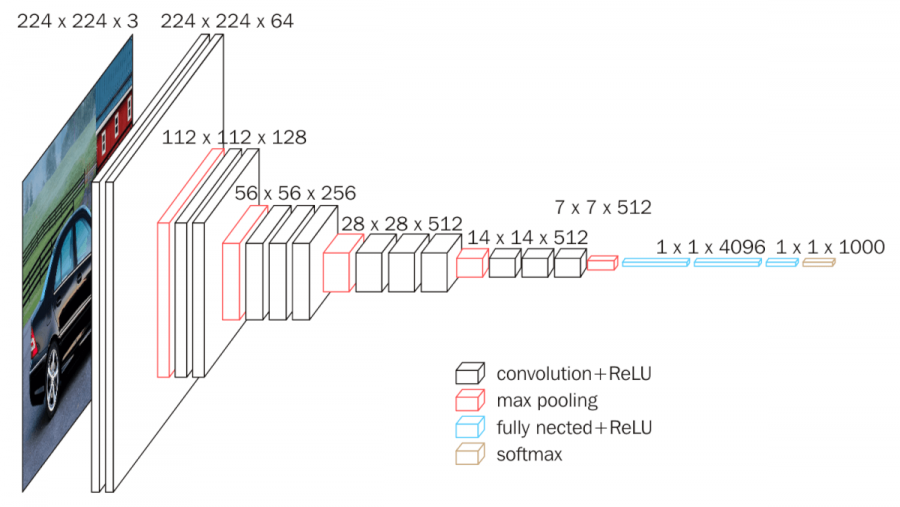
VGG16 is a convolutional neural network model proposed by K. Simonyan and A. Zisserman from the University of Oxford in the paper “Very Deep Convolutional Networks for Large-Scale Image Recognition”. The model achieves 92.7% top-5 test accuracy in ImageNet, which is a dataset of over 14 million images belonging to 1000 classes. It was one of the famous model submitted to ILSVRC-2014. It makes the improvement over AlexNet by replacing large kernel-sized filters (11 and 5 in the first and second convolutional layer, respectively) with multiple 3×3 kernel-sized filters one after another. VGG16 was trained for weeks and was using NVIDIA Titan Black GPU’s.
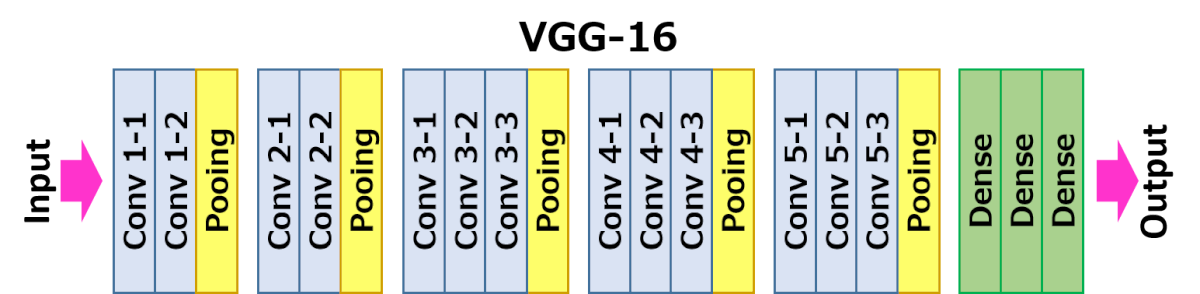
DataSet
ImageNet is a dataset of over 15 million labeled high-resolution images belonging to roughly 22,000 categories. The images were collected from the web and labeled by human labelers using Amazon’s Mechanical Turk crowd-sourcing tool. Starting in 2010, as part of the Pascal Visual Object Challenge, an annual competition called the ImageNet Large-Scale Visual Recognition Challenge (ILSVRC) has been held. ILSVRC uses a subset of ImageNet with roughly 1000 images in each of 1000 categories. At all, there are roughly 1.2 million training images, 50,000 validation images, and 150,000 testing images. ImageNet consists of variable-resolution images. Therefore, the images have been down-sampled to a fixed resolution of 256×256. Given a rectangular image, the image is rescaled and cropped out the central 256×256 patch from the resulting image.
The Architecture
The architecture depicted below is VGG16.
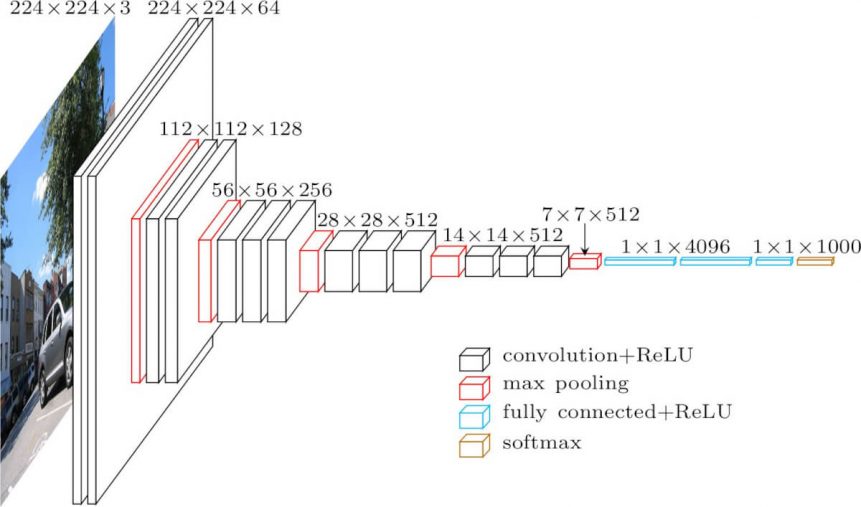
The input to cov1 layer is of fixed size 224 x 224 RGB image. The image is passed through a stack of convolutional (conv.) layers, where the filters were used with a very small receptive field: 3×3 (which is the smallest size to capture the notion of left/right, up/down, center). In one of the configurations, it also utilizes 1×1 convolution filters, which can be seen as a linear transformation of the input channels (followed by non-linearity). The convolution stride is fixed to 1 pixel; the spatial padding of conv. layer input is such that the spatial resolution is preserved after convolution, i.e. the padding is 1-pixel for 3×3 conv. layers. Spatial pooling is carried out by five max-pooling layers, which follow some of the conv. layers (not all the conv. layers are followed by max-pooling). Max-pooling is performed over a 2×2 pixel window, with stride 2.
Three Fully-Connected (FC) layers follow a stack of convolutional layers (which has a different depth in different architectures): the first two have 4096 channels each, the third performs 1000-way ILSVRC classification and thus contains 1000 channels (one for each class). The final layer is the soft-max layer. The configuration of the fully connected layers is the same in all networks.
All hidden layers are equipped with the rectification (ReLU) non-linearity. It is also noted that none of the networks (except for one) contain Local Response Normalisation (LRN), such normalization does not improve the performance on the ILSVRC dataset, but leads to increased memory consumption and computation time.
Configurations
The ConvNet configurations are outlined in figure 2. The nets are referred to their names (A-E). All configurations follow the generic design present in architecture and differ only in the depth: from 11 weight layers in the network A (8 conv. and 3 FC layers) to 19 weight layers in the network E (16 conv. and 3 FC layers). The width of conv. layers (the number of channels) is rather small, starting from 64 in the first layer and then increasing by a factor of 2 after each max-pooling layer, until it reaches 512.
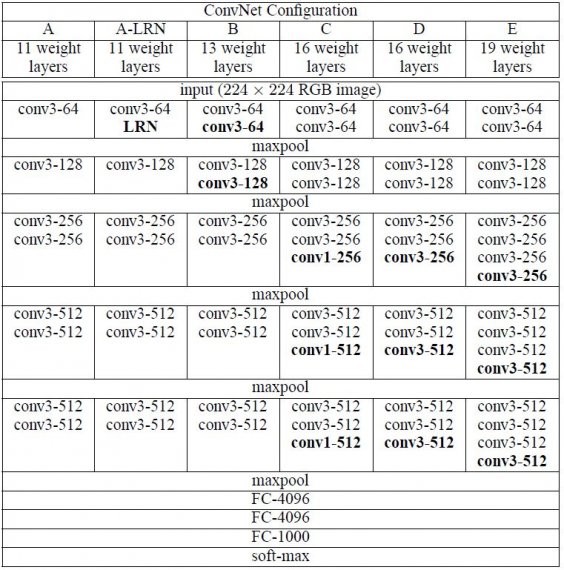
Use-Cases and Implementation
Unfortunately, there are two major drawbacks with VGGNet:
- It is painfully slow to train.
- The network architecture weights themselves are quite large (concerning disk/bandwidth).
Due to its depth and number of fully-connected nodes, VGG16 is over 533MB. This makes deploying VGG a tiresome task.VGG16 is used in many deep learning image classification problems; however, smaller network architectures are often more desirable (such as SqueezeNet, GoogLeNet, etc.). But it is a great building block for learning purpose as it is easy to implement.
[Pytorch]
[Keras]
Result
VGG16 significantly outperforms the previous generation of models in the ILSVRC-2012 and ILSVRC-2013 competitions. The VGG16 result is also competing for the classification task winner (GoogLeNet with 6.7% error) and substantially outperforms the ILSVRC-2013 winning submission Clarifai, which achieved 11.2% with external training data and 11.7% without it. Concerning the single-net performance, VGG16 architecture achieves the best result (7.0% test error), outperforming a single GoogLeNet by 0.9%.
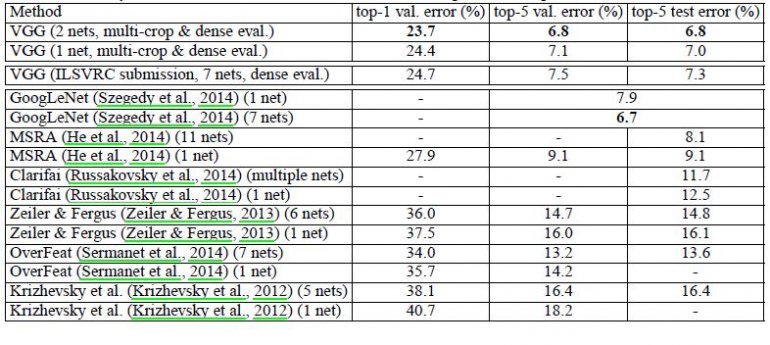
It was demonstrated that the representation depth is beneficial for the classification accuracy, and that state-of-the-art performance on the ImageNet challenge dataset can be achieved using a conventional ConvNet architecture with substantially increased depth.
[…] will use VGG16 as our deep learning model. We could have trained our own model from ground up, but this takes time […]
[…] Taken from: https://neurohive.io/en/popular-networks/vgg16/ […]
[…] map) at shallow layer(conv-1) of each conv block. A typical pre-trained classification CNN like VGG16 is consist of a few conv blocks, which has 2 or 3 convolution(Conv2D) layers(conv1,conv2… Read more »
[…] map) at shallow layer(conv-1) of each conv block. A typical pre-trained classification CNN like VGG16 is consist of a few conv blocks, which has 2 or 3 convolution(Conv2D) layers(conv1,conv2… Read more »
[…] המאומנות כמו VGG16, VGG19, Resnet101 ורבות אחרות נמצאות ברשת לשימוש הכל, עם […]
[…] a predição em diferentes elevações. Para agilizar o treinamento, eles usaram a estrutura VGG-16 inicializada com pesos oriundos de um pré-treinamento no dataset ImageNet. A tarefa específica do […]
[…] here’s some visualization of what the actual filters that pass over the images look like in a VGG16 network. (more specifically, an image on which the filter activates the… Read more »
[…] here’s some visualization of what the actual filters that pass over the images look like in a VGG16 network. (more specifically, an image on which the filter activates the… Read more »
[…] Nuerohive: Convolution Network for Classification and Detection […]
[…] Referencehttps://neurohive.io/en/popular-networks/vgg16/https://www.quora.com/What-is-the-VGG-neural-network […]
[…] this topic have focused on learning from famous, high-performance deep learning networks, such as VGGNet-16, ResNet-50, or Inception-V3/V4, etc. These networks were trained on the massive ImageNet database, […]
[…] have targeted on studying from well-known, high-performance deep studying networks, similar to VGGNet-16, ResNet-50, or Inception-V3/V4, and many others. These networks had been educated on the […]
[…] the advancement of deep learning such as convolutional neural network (i.e., ConvNet) [1], computer vision becomes a hot scientific research topic again. One of the main goals of […]
[…] of every picture within the coaching set are calculated utilizing a hidden layer of the pretrained VGG-16 convolutional neural community. A single absolutely related feed-forward layer is skilled to… Read more »
[…] 20 novembre 2018 VGG16 è un modello di rete neurale convoluzionale proposto da K. Simonyan e A. Zisserman dell’Università di Oxford nel documento “Reti convoluzionali molto profonde per il… Read more »
[…] features of each image in the training set are calculated using a hidden layer of the pretrained VGG-16 convolutional neural network. A single fully connected feed-forward layer is trained… Read more »
[…] features of each image in the training set are calculated using a hidden layer of the pretrained VGG-16 convolutional neural network. A single fully connected feed-forward layer is trained… Read more »
[…] ciascuno aspetto nel set nel corso di sono calcolate usando unico mano introvabile del pretrattatoVGG – 16 neurale convoluzionale. Un specifico calibro feed-forward del tutto collegato viene preparato verso… Read more »
[…] [3] “VGG16 – Convolutional Network for Classification and Detection”. [Online]. Available: https://neurohive.io/en/popular-networks/vgg16/. […]
[…] Layered architecture of VGG16 (Source) […]
[…] will use the pre-trained AlexNet and VGG16. They both receive 224*224 (3 channel) as input, so we need to resize our image to 224*224. Because […]
[…] An occasion of options at totally different ranges within the VGG-16 architecture […]
[…] An instance of features at different levels in the VGG-16 architecture […]
[…] Reference : 1. https://neurohive.io/en/popular-networks/vgg16/ […]
[…] https://neurohive.io/en/popular-networks/vgg16/ — VGG16 – Convolutional Network for Classification and Detection (emphasis mine) […]
[…] example, the neural nets, which can include VGG-16, RESNET-50, and others, have the following size when used as a tf.keras application (for example, […]
[…] VGG16 – Convolutional network for classification and detection. (2018, November 21). Neurohive – Neural Networks. https://neurohive.io/en/popular-networks/vgg16/ […]
[…] For image Detecting, we are using a pre-trained model which is VGG16. VGG16 is already installed in the Keras library.VGG 16 was proposed by Karen Simonyan and Andrew Zisserman… Read more »
[…] This isn’t an easy question because this is a full separate paper on its own, but I will give you an idea of what it is. Encoder decoder frameworks… Read more »
[…] This isn’t an easy question because this is a full separate paper on its own, but I will give you an idea of what it is. Encoder decoder frameworks… Read more »
[…] We will first run the images through the VGG16 base model. VGG16 is a pre-trained model that takes in (224,224) RGB images and converts them into features. It comes… Read more »
[…] We will first run the images through the VGG16 base model. VGG16 is a pre-trained model that takes in (224,224) RGB images and converts them into features. It comes… Read more »
[…] a single image prediction faster, I could always resort to using a network with fewer layers like VGG16, […]
[…] a single image prediction faster, I could always resort to using a network with fewer layers like VGG16, […]
[…] of this algorithm is that it takes a large scale of enterprise logos from a database and, utilizing VGG16 for feature extraction, forms K clusters of visually similar […]
[…] of this algorithm is that it takes a large scale of enterprise logos from a database and, utilizing VGG16 for feature extraction, forms K clusters of visually similar […]
[…] I was able to find the VGG16 CNN model (For more information of VGG16 model: VGG16 Paper and Blog post) and implemented the VGG16 model provided by the Python Tensorflow and Keras.… Read more »
[…] 引用: https://neurohive.io/en/popular-networks/vgg16/ […]
[…] [3] “VGG16 – Convolutional Network for Classification and Detection”. [Online]. Available: https://neurohive.io/en/popular-networks/vgg16/. […]
[…] [1] Detection of novel coronavirus from chest X-rays using deep convolu…[2] Fast coronavirus tests: what they can and can’t do[3] VGG16 – Convolutional Network for Classification and Detection […]
[…] [1] Detection of novel coronavirus from chest X-rays using deep convolutional neural networks [2] Fast coronavirus tests: what they can and can’t do [3] VGG16 – Convolutional Network for Classification… Read more »
[…] deep convolutional neural networks [2] Fast coronavirus tests: what they can and can’t do [3] VGG16 – Convolutional Network for Classification and DetectionSee […]
[…] [1] Detection of novel coronavirus from chest X-rays using deep convolu…[2] Fast coronavirus tests: what they can and can’t do[3] VGG16 – Convolutional Network for Classification and Detection […]
[…] is true that science never puts their feet apart from the chain of innovations and inventions. The VGG16 is similarly another invention by the industry’s intelligent performers, which led to the… Read more »
[…] the Convolutional Neural Network (CNN) model being attacked in the above example is VGGFace (VGG-16), trained on Columbia University’s PubFig dataset. Other attack samples developed by the […]
[…] Convolutional Neural Community (CNN) mannequin being attacked within the above instance is VGGFace (VGG-16), skilled on Columbia College’s PubFig dataset. Different assault samples developed by the […]
[…] the Convolutional Neural Network (CNN) model being attacked in the above example is VGGFace (VGG-16), trained on Columbia University’s PubFig dataset. Other attack samples developed by the […]
[…] Convolutional Neural Network (CNN) mannequin being attacked within the above instance is VGGFace (VGG-16), educated on Columbia College’s PubFig dataset. Different assault samples developed by the […]
[…] Convolutional Neural Community (CNN) mannequin being attacked within the above instance is VGGFace (VGG-16), educated on Columbia College’s PubFig dataset. Different assault samples developed by the […]
[…] the Convolutional Neural Network (CNN) model being attacked in the above example is VGGFace (VGG-16), trained on Columbia University’s PubFig dataset. Other attack samples developed by the […]