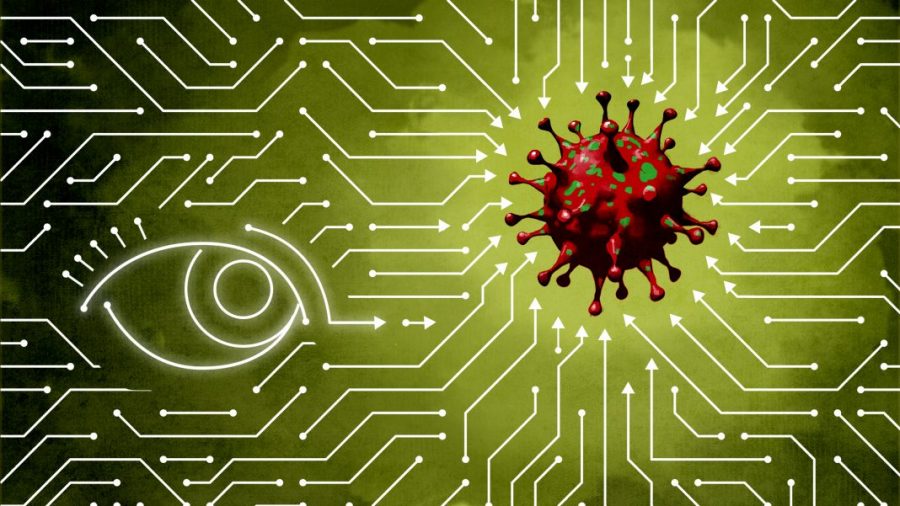
A group of researchers has created an approach to automatically predict the deterioration of patients’ condition using a neural network. The system was trained on chest x-rays and non-visual clinical parameters and showed a high result. The use of this system can play a role in helping patients with a new coronavirus infection.
Relevance
The main complication of COVID-19 is viral pneumonia. Radiography is one of the main tools for diagnosing infection. Although computed tomography provides higher resolution, a chest x-ray is less expensive, produces less radiation, and reduces the risk of transmission of infection to the equipment. Knowledge of the new disease is advancing rapidly but the understanding of the correlation between infected areas of the lungs shown on radiographs and patient deterioration remains limited.
Description of the technology
The system consists of two deep convolutional neural networks based on GMIC architecture. The first model, COVID-GMIC, predicts an overall risk of deterioration over 24, 48, 72, and 96 hours. Produces significance maps showing areas that justify its predictions. COVID-GBM learns from regularly collected clinical patient data such as weight, body temperature, immune cell levels, and connects its predictions with COVID-GMIC. The connection takes place using a fusion module that combines information from both the global context and local details to make a holistic prediction. The second model, COVID-GMIC-DRC, predicts how a patient’s deterioration changes over time in plots.
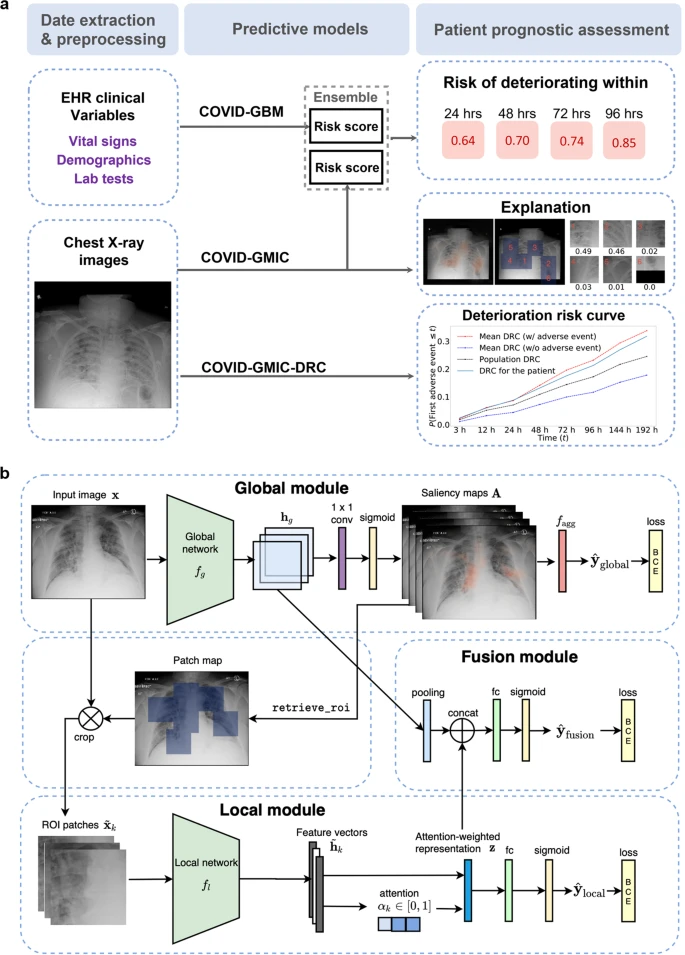
The system was evaluated using a dataset of chest radiographs of patients who were tested for COVID-19 by PCR and tested positive. We also collected the latest clinical data on the patient’s condition. A training set of 5617 chest scans was used to model and tune hyperparameters using Monte Carlo cross-validation. The 832m test set was used to report the final image results.
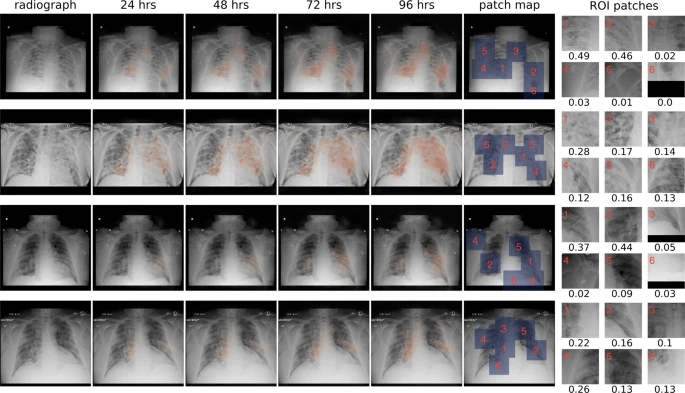
Results
The model provided an accuracy comparable to that of two experienced physicians: AUC value of 0.786, PR AUC (Precision Call AUC) 0.517. This emphasizes the potential of the developed approach. According to the developers, the preliminary version was implemented at NYU Langone Health during the first wave of the pandemic.
Conclusion
The developed system of assistance to doctors of the emergency department to predict the condition of patients, thereby optimizing the processes of care. The researchers made the code and trained models publicly available.