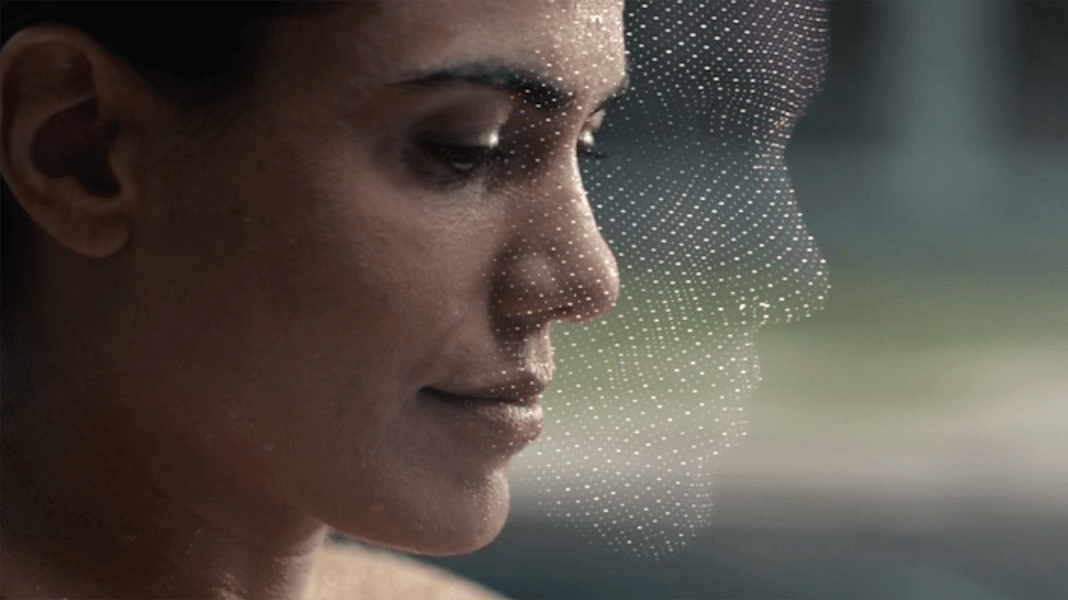
With the rapid progress of deep learning in the past few years, many computer vision problems have been tackled and solved with human or even beyond human performance. One of these tasks, in fact, a very popular one, is face recognition.
Until the recent past, face recognition was seen as something straight out of science fiction. But, over the past decade or two face recognition has not only become a solved problem but a widespread technology with applications in several industries.
Previous Works
Since face recognition is a challenging task it took some time for researchers in different domains to reach satisfactory results. Researchers the domain of pattern recognition, computer vision and artificial intelligence have proposed many solutions in the past. The main goal was to reduce difficulties such as highly variable face poses, image quality etc., so as to improve the robustness and recognition accuracy.
A number of deep learning-based face recognition methods have been proposed in the past few years. Starting with remarkable results with DeepFace (2014), then methods like DeepID (2014), FaceNet (2015), VGGFace (2015), all the way to more recent methods like Cosface (2018), ArcFace (2018).
State-of-the-art Idea
Recently, researchers from Pohang University of Science and Technology in Korea have proposed a novel face recognition method that achieved state-of-the-art results on some of the benchmark datasets. The new method, called pairwise relational network (PRN) takes local appearance features around landmark points on the feature map and captures unique pairwise relations with the same identity and discriminative pairwise relations between different identities.
In fact, the idea is to build a method which is able to represent an image of a face in such a manner that the features extracted will be discriminative across faces of different people.
Method
The proposed method works by taking local appearance features as input by ROI projection around landmark points on the feature map. These features are used to train a PRN (pairwise relational network) to capture unique pairwise relations between pairs of local appearance features. Arguing that the existence of such pairwise relations is identity dependent, the researchers employ LSTM to learn additional facial identity state feature. The architecture of the method as well as of the pairwise relational network is given in the figure below.
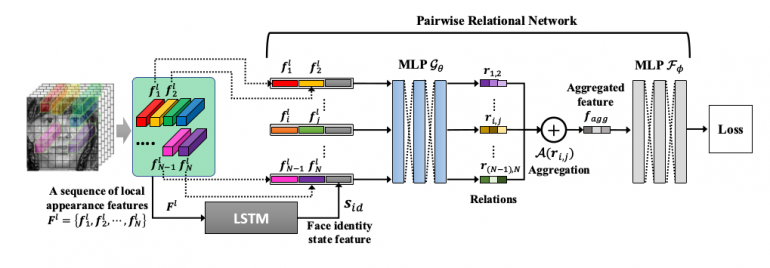
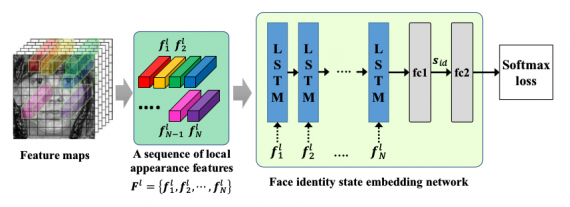
From a perspective of learning and optimization, the method uses combined triplet ratio loss, pairwise loss, and softmax loss. Stochastic Gradient Descent was used as the optimization method with an initial learning rate of 0.1.
Evaluation and Comparison
The proposed method was evaluated on the LFW dataset which reveals the state-of-the-art face verification in unconstrained environments. It is a popular and good dataset for evaluating face recognition methods. It contains 13, 233 of highly variable images of faces from 5, 749 different identities. The PRN method reaches 99.76% accuracy on this dataset which is almost the same accuracy as the state-of-the-art method ArcFace (99.78%).
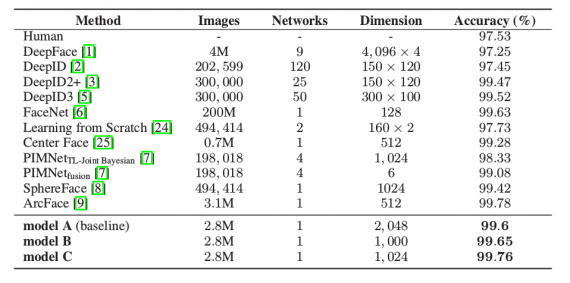
However, when evaluated on the YTF dataset (with similar characteristics as LFW dataset) the PRN method achieves state-of-the-art results – 96.3%.
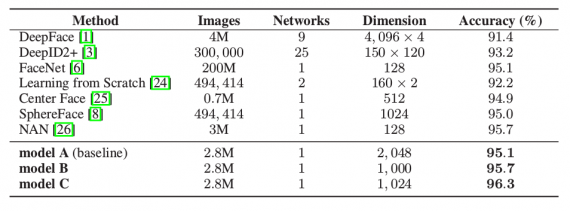
Additionally, the method was evaluated on IJB-A and IJB-B datasets for evaluating face verification and face identification. The results obtained on these datasets as well as on LFW and YTF compared with other methods are reported in the tables below.

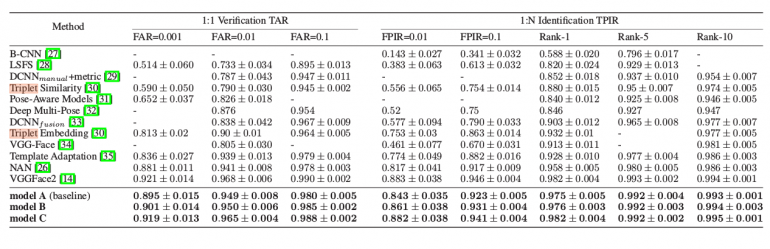
IJB-A dataset.
Conclusion
The researchers proposed an interesting approach to a well-known problem – face recognition. In their paper, they show that capturing those kinds of unique and discriminative pairwise relations actually solves the problem of face identification to a high degree of accuracy. Extensive experiments have been done on popular datasets and the method achieves very good results on all of them and state-of-the-art performance on one of them.