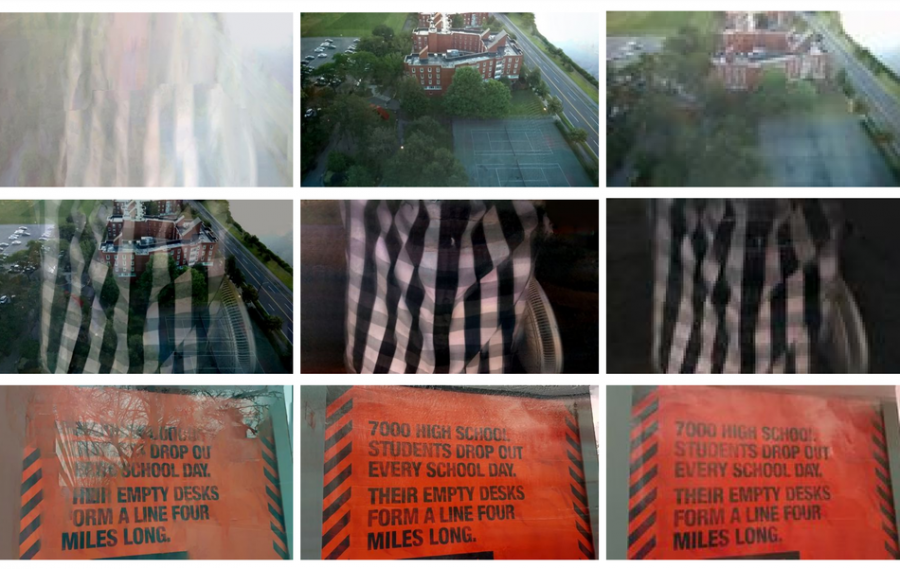
A group of researchers led by Yu-Lun Liu has proposed a deep learning-based method that removes unwanted obstructions such as raindrops, fences or window reflections from short videos.
Very often, videos captured “in-the-wild” have occlusions and unwanted obstructions in the frames. The removal of such noise or obstructions from videos is crucial, especially in cases where the videos are consumed by computers which need to perform some kind of reasoning.
In their novel paper named “Learning to See Through Obstructions“, researchers tackle this problem by capturing the motion differences between the background and the obstructing elements. Their method based on dense optical flow field estimation employs a deep convolutional neural network to be able to reconstruct several of the layers, separating the background from the unwanted “occlusion” layers. The first stage of this separation is flow decomposition, followed by two subsequent stages, which actually represent modules in the proposed method’s architecture: background and obstruction layer reconstruction stage and finally optical flow refinement.
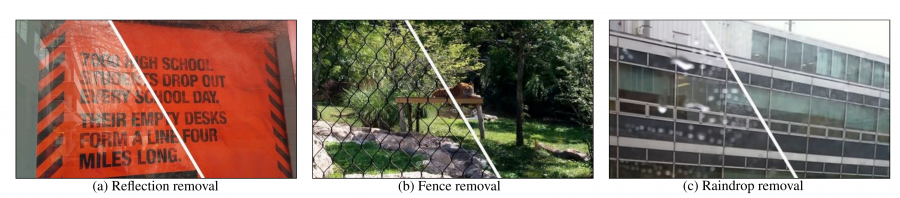
The proposed model was trained using a two-stage training procedure in order to ensure training stability. Researchers propose to first train an initial flow decomposition network, and then freeze this network and train the layer reconstruction networks.
Both synthetic and real data sequences were used to evaluate the performance of the proposed method. Researchers report that the new method performs well on a wide variety of scenes. They compared the method to some of the existing methods and showed that it performs on-par or better than existing methods.
More details about the method and the conducted experiments can be read in the paper. The implementation was open-sourced and is available on Github.