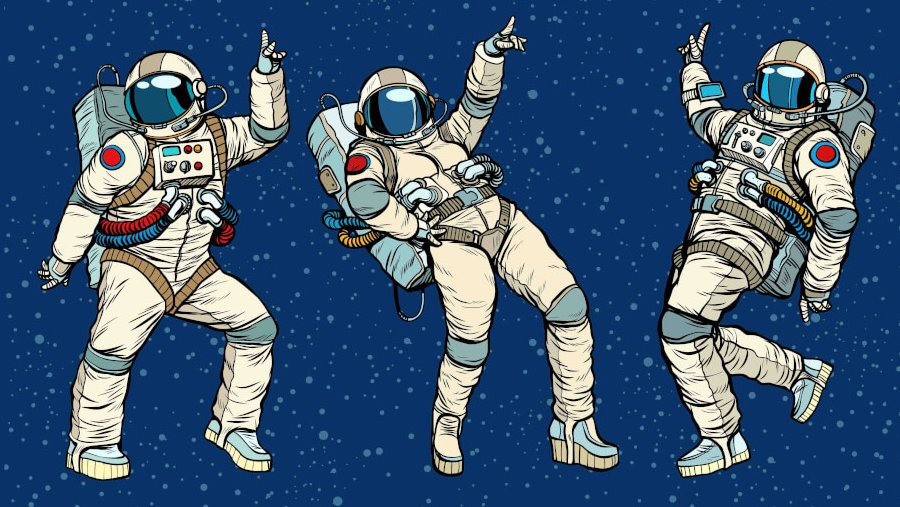
Not very good at dancing? Not a problem anymore! Now you can easily impress your friends with a stunning video, where you dance like a superstar.
Researchers from UC Berkeley propose a new method of transferring motion between human subjects in different videos. They claim that given a source video of a person dancing they can transfer that performance to a novel target after only a few minutes of the target subject performing standard moves.
But let’s first check what were the previous approaches to this kind of tasks.
Previous Works
Motion transfer or retargeting received a considerable attention from researchers over the last two decades. Early methods were creating new content by manipulating existing video footage.
- Video Rewrite approach, suggested by Bregler and his colleagues in 1997, creates videos of a subject saying a phrase they did not originally utter by finding frames where the mouth position matches the desired speech.
- Efros et al.’s method presented in 2003, uses optical flow as a descriptor matches different subjects performing similar actions allowing “Do as I do” and “Do as I say” retargeting.
- Another approach can imply using 3D transfer motion for graphics and animation purposes. Recently, Villegas et al. demonstrated how deep learning techniques can help with retargeting motion without supervised data.
- The current state-of-the-art methods can successfully generate detailed images of human subjects in novel poses (for instance, Joo et al.’s approach to generating a fusion image).
- Moreover, such images can be synthesized for temporally coherent video and future prediction.
So, what is the idea behind this new approach?
State-of-the-art Idea
This method poses the problem as a per-frame image-to-image translation with spatiotemporal smoothing. The researchers use pose detection, represented with the pose stick figures, as an intermediate representation between source and target. The aligned data is used to learn an image-to-image translation model between pose stick figures and images of the target person in a supervised way.
Two additional components improve the results: conditioning the prediction at each frame on that of the previous time step for temporal smoothness and a specialized GAN for realistic face synthesis.
Before diving deeper into the architecture of the suggested approach, let’s check the results with this short video:
So, in essence, the model is trained to produce personalized videos of a specific target subject. Motion transfer occurs when the pose stick figures go into the trained model to obtain images of the target subject in the same pose as the source.
Method
The pipeline of suggested approach includes three stages:
- Pose detection – using a pretrained state-of-the-art pose detector to create pose stick figures from the source video.
- Global pose normalization – accounting for differences between the source and target subjects in body shapes and locations within the frame.
- Mapping from normalized pose stick figures to the target subject.
Here is an overview of the method:
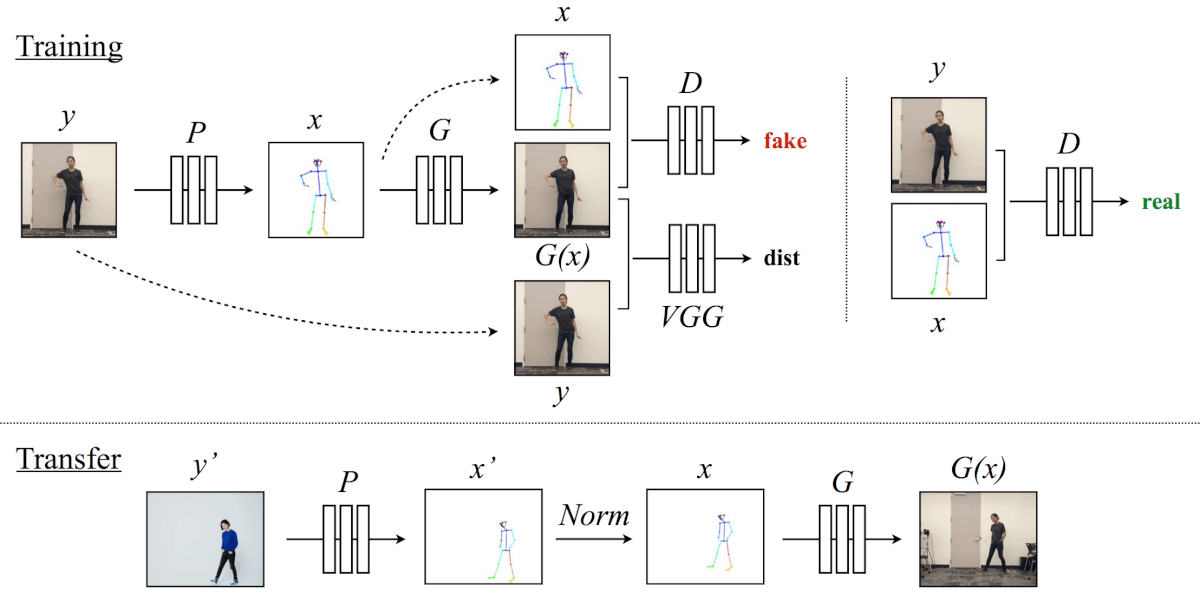
For the training purposes, the model uses a pose detector P to create pose stick figures from video frames of the target subject. Then, the mapping G is learned alongside an adversarial discriminator D, which attempts to distinguish between the “real” correspondence pair (x, y) and the “fake” pair (G(x), y).
Next, for the transferring purposes, a pose detector P helps to obtain pose joints for the source person. These are then transformed with the normalization process Norm into the joints for the target person for which pose stick figures are created. Finally, the trained mapping G is applied.
The researchers base their method on the objective presented in pix2pixHD with some extensions to produce temporally coherent video frames and synthesize realistic face images.
Temporal Smoothing
To create video sequences, they modify the single image generation set up to enforce temporal coherence between adjacent frames as shown in the figure below.
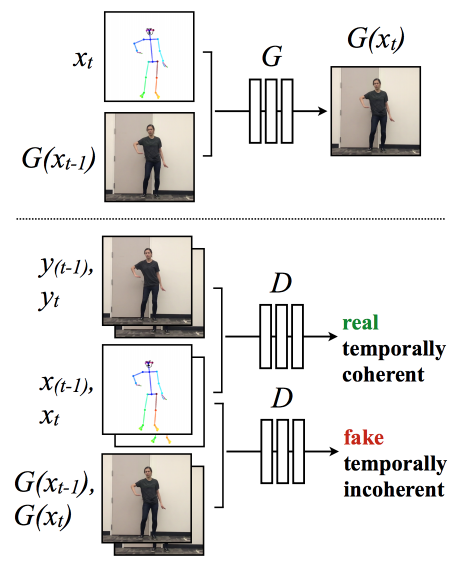
In brief, the current frame G(xt) is conditioned on its corresponding pose stick figure xt and the previously synthesized frame G(xt−1) to obtain temporally smooth outputs. Discriminator D then attempts to differentiate the “real” temporally coherent sequence (xt−1, xt , yt−1, yt ) from the “fake” sequence (xt−1, xt , G(xt−1), G(xt)).
Face GAN setup
The researchers further extend the model with a specialized GAN setup designed to add more detail and realism to the face region as shown in the figure below. To be specific, the model uses a single 70×70 Patch-GAN discriminator for the face discriminator.
Now let’s move to the results of the experiments…
Results
The target subjects were recorded for around 20 minutes of real time footage at 120 frames per second. Moreover, considering that the model does not encode information about clothes, the target subjects wear tight clothing with minimal wrinkling.
Videos of the source subjects were found online – these videos just need to be of the reasonably high quality and include the subject performing a dance.
Here are the results with the top row showing the source subject, the middle row showing the normalized pose stick figures, and the bottom row depicting the model outputs of the target person:
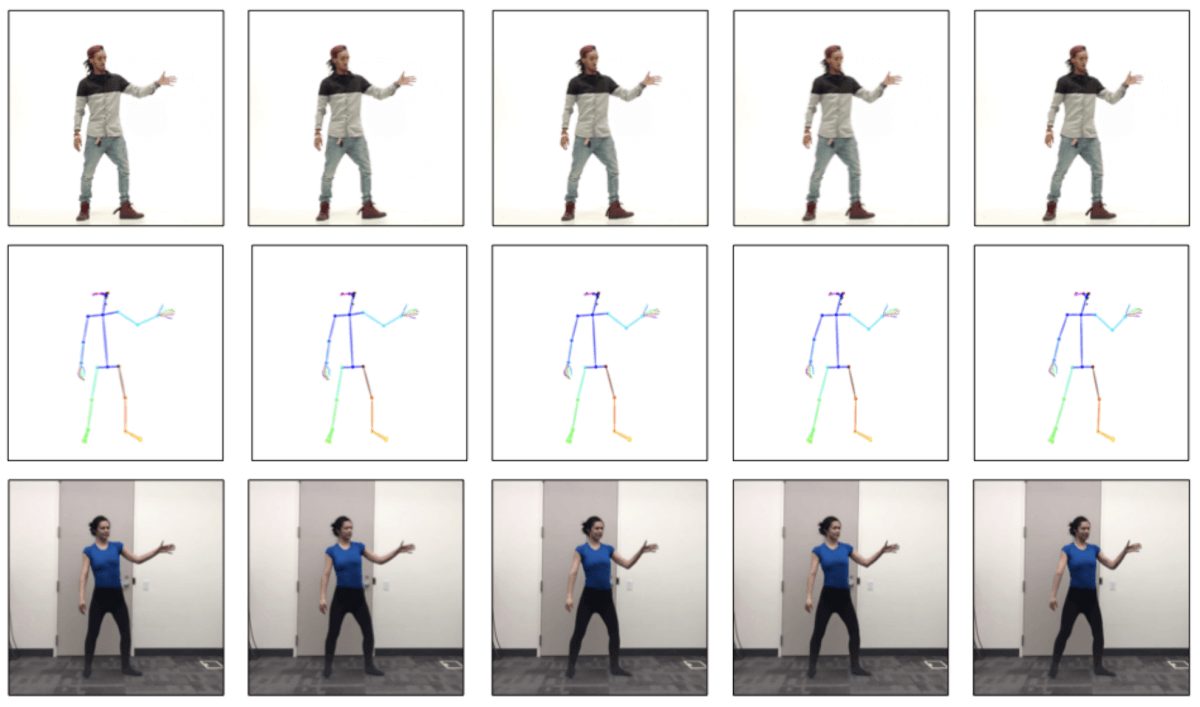
The tables below demonstrate the performance of the full model (with both temporal smoothing and Face GAN setups) in comparison to the baseline model (pix2pixHD) alone and the baseline model with a temporal smoothing setup. The quality of individual frames was assessed with the measure of Structural Similarity (SSIM) and Learned Perceptual Image Patch Similarity (LPIPS).
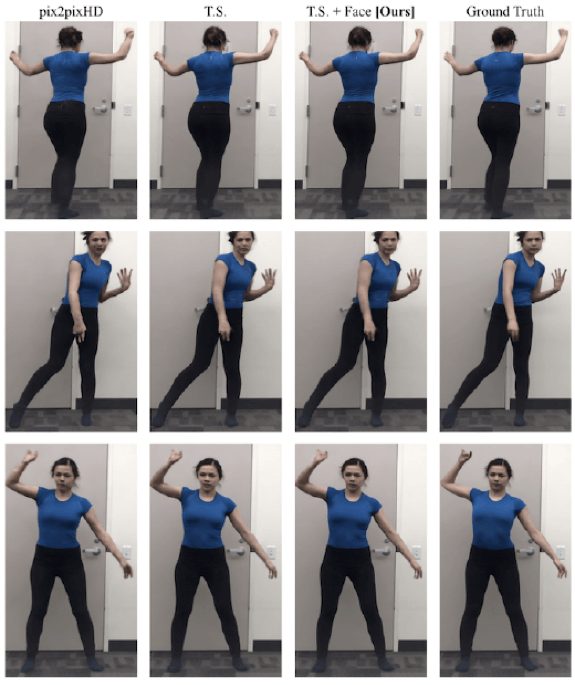
To further analyze the quality of the results, the researchers run the pose detector P on the outputs of each model and compare these reconstructed key points to the pose detections of the original input video. If all body parts are synthesized correctly, then the reconstructed pose should be close to the input pose on which the output was conditioned. See the results in the tables below:
As you can see from the tables, the temporal smoothing setup doesn’t seem to add much to the baseline if looking only on the quantitative results. However, qualitatively, the temporal smoothing setup helps with smooth motion, color consistency across frames, and also in individual frame synthesis.
Face GAN setup, on the other hand, improves both the quantitative and qualitative results of the model. As obvious from the pictures below, this component adds considerable detail to the output video and encourages synthesizing realistic body parts.
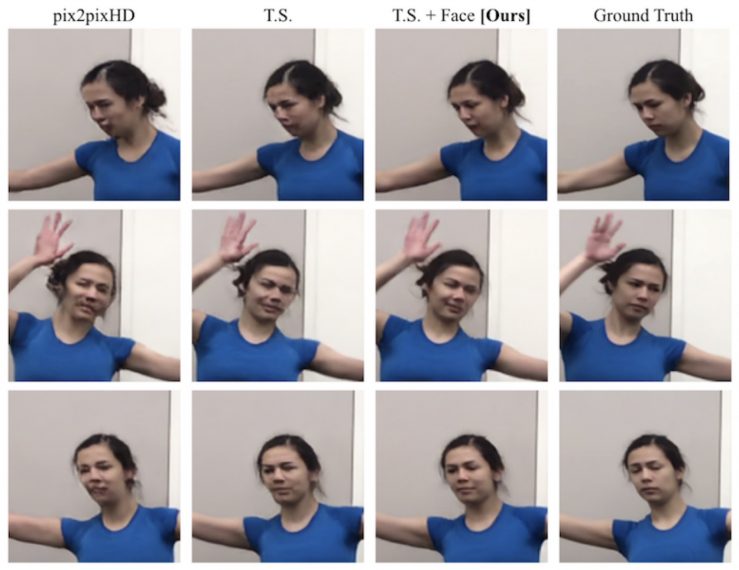
Conclusion
The presented model is able to create reasonable and arbitrarily long videos of a target person dancing given body movements to follow through an input video of another subject dancing. However, the results still often suffer from jittering. This is especially the case when the input movements or movement speed is different from the movements seen at the training time.
Considering that jittering and shakiness remain even if the target person tries to copy the movements of the source subject during the training sequence, the researchers suppose that jittering could also result from the underlying difference between how the source and target subjects move given their unique body structures. Still, this approach to motion transfer is able to generate compelling videos given a variety of inputs.