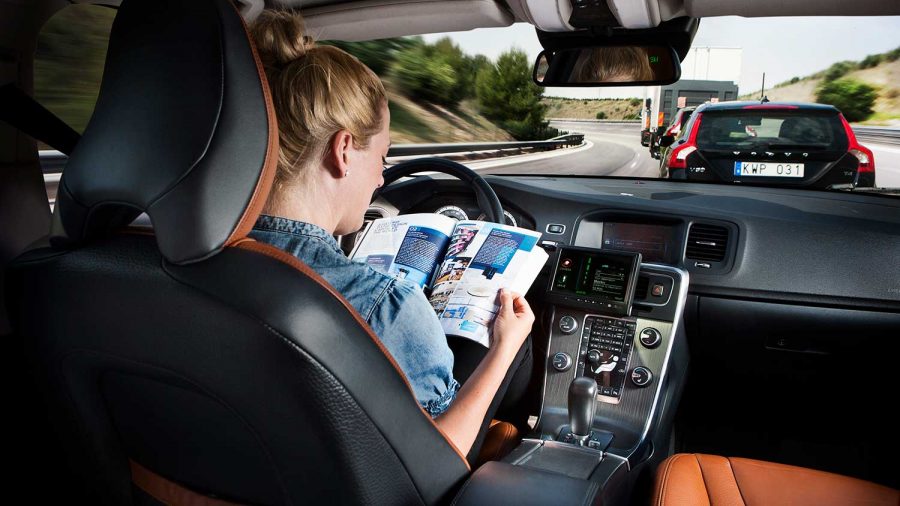
Within just a couple of years, self-driving cars have gone from science fiction to “now commercially available” road-bound reality. This year’s CES (the largest consumer electronics show) was “flooded” with self-driving demonstrations and showcases, showing how popular the topic of autonomous driving is right now.
Also, in the last few months, we have witnessed the first commercial self-driving taxi services. Waymo (former Google Project and now part of Alphabet) started it’s first commercial self-driving taxi service two months ago. Yandex launched Europe’s first commercial driverless taxi service last summer.
Despite all that, we can safely claim that self-driving cars are not there yet. One might argue that self-driving cars are already a reality because there are cars currently on the road able to drive by themselves (Tesla autopilot for example). But, is it the case?
What Is Autonomous Driving?
For the moment we only have partially automated driving, but not autonomous driving. We can only find self-driving cars that can ride in the fully autonomous mode in particular situations. And even worse, they have to be supervised by a human driver.
But what does autonomous driving mean? Is a vehicle being able to drive with little human intervention? A vehicle that is capable of navigating in an intelligent manner? A car that can drive without any human help, in any situation, and under any conditions? Well, there are many definitions. And the understanding of the term “autonomous driving” by the public varies troublesomely.
From a theoretical perspective:
“A fully autonomous system should be able to: gain information about the environment, work for an extended period without human intervention and gain knowledge like adjusting for new methods of accomplishing its tasks or adapting to changing surroundings.”
It is evident that at the moment, the state of self-driving cars is quite far from this definition, and even more it is an open question if we are ever getting close to an autonomous system as defined above.
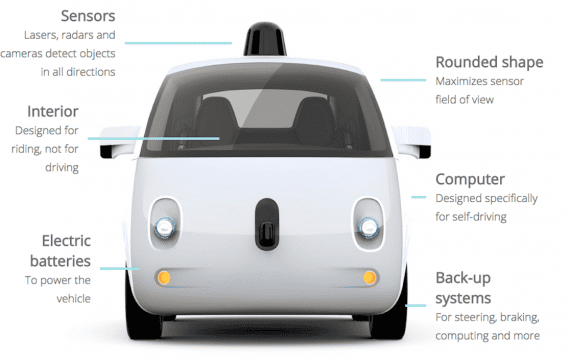
We have to be careful because we could end up in the quagmire of an endless semantic argument, which would add little value. Instead, it is essential to focus on things that matter and advance the technology itself.
To keep up with automated vehicle technology advances and provide global engineering community common platform to work from, SAE, the automotive standardization body published the SAE Information Report: (J3016) “Taxonomy and Definitions for Terms Related to On-Road Motor Vehicle Automated Driving Systems.” With this report, SAE formally defined six different levels of automation of autonomous vehicles, and this helps keep track of the advancement of driverless technology.
Self-Driving Cars Levels of Autonomy
Level 0: Automated system issues warnings and may momentarily intervene but has no sustained vehicle control.
Level 1 (“hands-on”): The driver and the automated system share control of the vehicle. Examples are Adaptive Cruise Control (ACC), where the driver controls steering and the automated system controls speed; and Parking Assistance, where steering is automated while speed is under manual control. The driver must be ready to retake full control at any time. Lane Keeping Assistance (LKA) Type II is a further example of level 1 self-driving.
Level 2 (“hands-off”): The automated system takes full control of the vehicle (accelerating, braking, and steering). The driver must monitor the driving and be prepared to intervene immediately at any time if the automated system fails to respond properly. The shorthand “hands off” is not meant to be taken literally. Contact between hand and wheel is often mandatory during SAE 2 driving, to confirm that the driver is ready to intervene.
Level 3 (“eyes off”): The driver can safely turn their attention away from the driving tasks, e.g., the driver can text or watch a movie. The vehicle will handle situations that call for an immediate response, like emergency braking. The driver must still be prepared to intervene within some limited time, specified by the manufacturer when called upon by the vehicle to do so. As an example, the 2018 Audi A8 Luxury Sedan was the first commercial car to claim to be capable of level 3 self-driving. This particular car has a so-called Traffic Jam Pilot. When activated by the human driver, the car takes full control of all aspects of driving in slow-moving traffic at up to 60 kilometers per hour (37 mph). The function works only on highways with a physical barrier separating one stream of traffic from oncoming traffic.
Level 4 (“mind off”): As level 3 the driver may safely go to sleep or leave the driver’s seat. Self-driving is supported only in limited spatial areas (geofenced) or under special circumstances, like traffic jams. The vehicle must be able to safely abort the trip, e.g., park the car, if the driver does not retake control.
Level 5 (“steering wheel optional”): No human intervention is required at all. An example would be a robotic taxi.
In the next sections of this article, I am going to briefly explain the main components of current self-driving cars and highlight the current state of development and importance of each as well as key players involved.
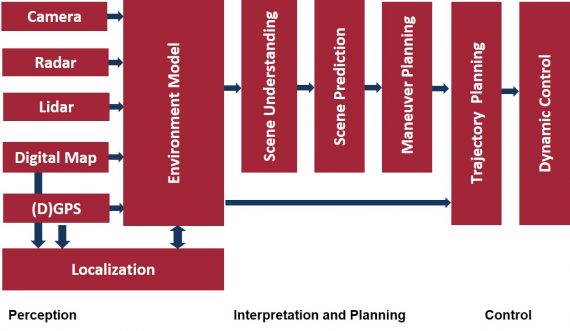
Autonomous Driving Hardware
When it comes to autonomous driving, a big part of the discussion is around AI, algorithms, and software. But hardware plays a crucial role as the components mentioned above for the advances of autonomous vehicle’s technology.
On the hardware side, autonomous driving components include sensors, connectivity technology, actuators and also specialized driverless platforms.
Sensors
A very crucial component for self-driving vehicles are the sensors. They provide the car with all the information about its environment and surroundings. The ability to perform automated tasks or full autonomous driving heavily depends on the ability to get enough, correct and relevant data about the state of the environment.
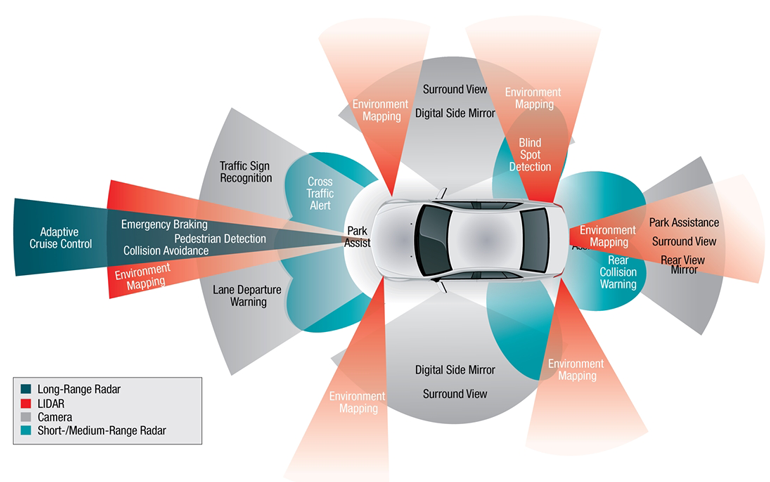
LIDAR
LIDAR sensors are said to be the future or self-driving cars. They provide 3D scans of the environment and therefore much more information than camera sensors. Cameras deliver the detail but require machine-learning-powered software that can translate 2D images into 3D understanding. Lidar, by contrast, offers hard, computer-friendly data in the form of exact measurements.
Most of the serious players in autonomous driving consider LIDAR sensor as an indispensable component of the self-driving car. However, due to the high cost of the sensor itself (not to mention the difficulties processing large amounts of 3D scans from LIDAR in real-time), it is not certain if LIDAR sensors will make it as a component of the future, level 5 autonomous car.
The LIDAR sensor market grows at a fast pace due to its potential use in autonomous vehicles. However, at the moment the considerable cost poses a significant threat to the LIDAR market.
Key Players are
- Continental entered the business of LIDAR sensors by acquiring the Hi-Res 3D Flash LIDAR business from Advanced Scientific Concepts, Inc. (ASC) back in March 2016.
Now continental offers it’s 3D flash LIDAR (with no moving parts) and a short range flash LIDAR (SRL-121). - Velodyne offers it’s AlphaPuck LIDAR sensor specialized for AD. It costs around 12 000$, and it’s the first 300m sensor for autonomous fleets with 128 channels(layers). Other than AlphaPuck (improved and specialized VelodynePuck), Velodyne offers Veladome and Velarray. The older version of AlphaPuck had its price reduced by 50% last year, due to improvements in Velodyne’s manufacturing process.
The HDL-64E is the most powerful LIDAR sensor from Velodyne, having 64 layers, 360 FOW and up to ~2.2 Million Points per Second. HDL-64E is extremely expensive, having a price of around 100 000$. - Valeo’s Scala laser scanner (LIDAR) is the world’s first automotive-grade commercialized laser scanner. It is a mechanical LIDAR covering 145 degrees. Valeo partnered with Ibeo and Audi to make its Scala LIDAR available commercially.
So, the LIDAR market appears to be fragmented, and with the presence of several companies including Velodyne LiDAR and Valeo, the competitive environment is quite intense.
Camera
When it comes to camera sensors, the notable difference is the cost. Cameras are relatively cheap sensors, and an autonomous vehicle can have more than a few, to cover a wide range of the environment. We can see companies like Tesla relying mostly on camera and vision (Tesla tries to avoid LIDAR in their sensor set), to automate the task of driving. Also, when it comes to cameras on the market, we usually see them tightly coupled with vision software solutions (with or without AI) or embedded in specialized sensors for AD. We must note that as of now, cameras are the primary computer perception solution for advanced driver assistance systems (ADAS).
Ambarella is a company that works on a camera-only self-driving car. Ambarella’s vehicles employ dozen of their SuperCam3 4K stereo cameras, each with a 75-degree field of view.
Mobileye is another company working in the field of camera and vision for AD. Mobileye’s mono-camera is coupled with Mobileye’s vision solutions to support Advanced Driver Assistance Systems (ADAS).
Other players in the sector of cameras and vision for AD include DeepVision, Vaya Vision, Chronocam, Roadsense, Nvidia, etc.
Radar
Radar is the third most common sensor for self-driving vehicles. Compared to LIDAR and optical sensing technologies, radar is mostly insensitive to environmental conditions like fog, rain, wind, darkness, or bright sun, but technical challenges remain. Many OEMs and big players in the self-driving market are investing in Radar technology. Tesla is using RADAR as one of the primary sensors for their autonomous cars.
Some of the key players include Bosch, Valeo, 6th sense, SDS, Toposens, etc.
V2X
V2X refers to communication from a vehicle to any entity that may affect the vehicle. V2X technology is a significant component of self-driving cars. V2X systems include communication technologies such as vehicle-to-vehicle (V2V), vehicle-to-infrastructure (V2I), vehicle-to-pedestrian (V2P), and vehicle-to-network (V2N).
The most prominent challenge for V2X growth today is the requirement of high reliability, low latency communications system, with the two main technologies at play here — IEEE 802.11p and Cellular V2X both having their respective advantages and disadvantages.
A few days ago, Ford announced that by 2022 all its cars and trucks sold in the US would feature C-V2X capabilities provided by Qualcomm. Also as part of V2X, some demonstrations recently focused on roadside units (RSUs) that support Vehicle-to-Infrastructure communications.
Key players: Continental (Germany), Qualcomm (US), NXP Semiconductors (Netherlands), Robert Bosch (Germany), Denso (Japan) and Delphi Automotive (U.K.).
Drive Platforms
NVIDIA is one of the few companies into manufacturing specialized hardware platforms for autonomous driving that are supposed to provide the necessary computing power and optimize the self-driving software.
NVIDIA DRIVE AGX is a scalable, open, autonomous vehicle computing platform that serves as the brain for autonomous vehicles. The only hardware platform of its kind, NVIDIA DRIVE AGX delivers high-performance, energy-efficient computing for functionally safe AI-powered self-driving.
Self-Driving Cars Software
When discussing autonomous vehicles most of the discussion is towards vehicle’s intelligence and ability to make decisions. As I mentioned before, software seems to be in the spotlight. This is probably because Artificial Intelligence comes in the form of software (as of now).
ADAS
ADAS, or Advanced Driving-Assistance Systems encapsulates all algorithms that assist in performing a driving task. ADAS also includes algorithms that are part of automated driving not only algorithms and software specific for autonomous vehicles. Most of the ADAS software can be divided into three major groups: Perception, Planning, and Control.
Perception
Perception is probably one of the most important and crucial components of an autonomous vehicle, as it is the only connection point between the car and the surrounding environment. Artificial Intelligence is used pretty much in perception algorithms to make sense of “raw” sensor data (or V2X data since V2X data comes as external observations also). Algorithms such as Sensor Fusion, Object Detection, Object Tracking, Object Fusion, etc. fall into the perception part. Latest state-of-the-art perception algorithms use mostly deep neural networks.
Planning
The planning module refers to the ability of the autonomous vehicle to make certain decisions to achieve some higher order goals. The planning system works by combining the processed information about the environment (from perception output, i.e., from the sensors and V2X components) with established policies and knowledge about how to navigate in the environment. Planning makes heavy use of maps and rule catalogs that define “valid” driving operations. This part includes algorithms such as scenario detection, scenario classification, trajectory planning.
Control
The control system takes care of converting the intentions and goals derived from the planning system into actions. The control system tells the hardware (the actuators) the necessary inputs that will lead to the desired motions (according to the output of the trajectory planning process). There are many algorithms in the control module that take care to make driving smooth and more human-like.
Virtual Simulations
To build complex AI algorithms and get a satisfactory result, large amounts of driving data is required. However, the cost of collecting massive amounts of data, and especially the cost of collecting enough data of critical (rare-occurring) driving scenarios is very high, and this makes it difficult to train (good enough) AI models using real-world driving data.
Moreover, to demonstrate that a self-driving system has a lower collision rate than human drivers requires a sizable test fleet driving many miles. As a result, it’s complicated to verify and validate vehicle self-driving capabilities only using on-road testing.
This is where a different solution comes into play – Simulations. Researchers in machine learning and AI have realized over time that it is a good idea to use synthetic data and simulations to train large models. Now it is evident that Simulations will play a crucial role in the autonomous driving world. Both issues (of training deep models and validation) can be solved using data from simulations (solely or in combination with real-world data).
There are a few companies now specializing in virtual simulations for autonomous driving: Automotive Artificial Intelligence (AAI), rFpro, NVIDIA (Drive Constellation Software), etc.
Autonomous Vehicles Companies Testing Solutions
- Aptiv began offering rides in their autonomous cars during the Consumer Electronics Show in January last year. The company currently has 30 autonomous vehicles on the roads in Sin City; they cruise around 20 hours a day, seven days a week.
- Aurora is a company working with Volkswagen, Hyundai, and Byton. Their self-driving VW e-Golfs and Lincoln MKZs are on the streets of Palo Alto, San Francisco, and Pittsburgh.
- BMW Group is working with Intel and Mobileye to create semi- and fully automated cars, and they are also working with Waymo to include its technologies for sensor processing, connectivity and artificial intelligence. Also there are rumours that rivals BMW and Daimler are considering joining forces on autonomous driving.
- General Motors has owned self-driving car company Cruise since 2016, and their gen-three autonomous Chevy Bolts are the roads of San Francisco, Scottsdale, Arizona, and Orion, Michigan.
- Drive.ai is already offering a self-driving service in two places in Texas: Frisco and Arlington. Both of those towns are in the Dallas, Fort Worth area, and both services use self-driving vans and safety drivers to shuttle people around in a specific, geofenced region. Andrew Ng, a deep learning pioneer, and the professor is a board member of Drive.ai, which raised more than 77 million euros in funding, to date.
- Ford is working with a company called Argo AI for its self-driving cars. The company has autonomous vehicles on the road in Dearborn, Michigan, and Miami and Pittsburgh. Last week, VW announced a collaboration with Ford on electric and self-drive technology.
- Tesla has all their cars produced with the hardware needed for full self–driving capability. Tesla already offers its Autopilot, which means some degree of autonomy.
- Uber‘s cars will cruise in self-driving mode on a route in Pittsburgh. Uber says those vehicles will only run on weekdays and during the day—but that they could expand their testing area, and the conditions (like weather) under which they drive, as time goes on. Uber canceled their self-driving experiments after a tragic accident that killed a woman in the first half of 2018. Recently they have restarted their program.
- Yandex is currently testing their self-driving cars in two Russian cities. They were the first to offer commercial self-driving services in Europe.
- Volvo is also investing a lot in autonomous cars but also in trucks. Yesterday they announced that Volvo self-driving prototypes are back on the road and this time the Swedish carmaker will be testing them in Sweden. As informed by Veoneer with whom Volvo has partnered to develop self-driving vehicles, the joint venture (JV) has been permitted by the Swedish government to conduct the tests on country highways.
- Waymo is one of the companies that are advancing at a very fast pace. Waymo already has their self-driving cars commercially available.
More companies are testing their autonomous vehicles at the moment. The ones described above serve only as a reference, and the list is non-exhaustive.
To conclude, 2019 will be an exciting year which is expected to bring a lot regarding the advancement of autonomous driving. It is yet to be seen if the technology that we are developing can bring self-driving cars on the roads, or a technological breakthrough will be needed to pursue the ambitions.
[…] assistance systems are another common Level 1 autonomous feature. The driver controls the accelerator and brake […]