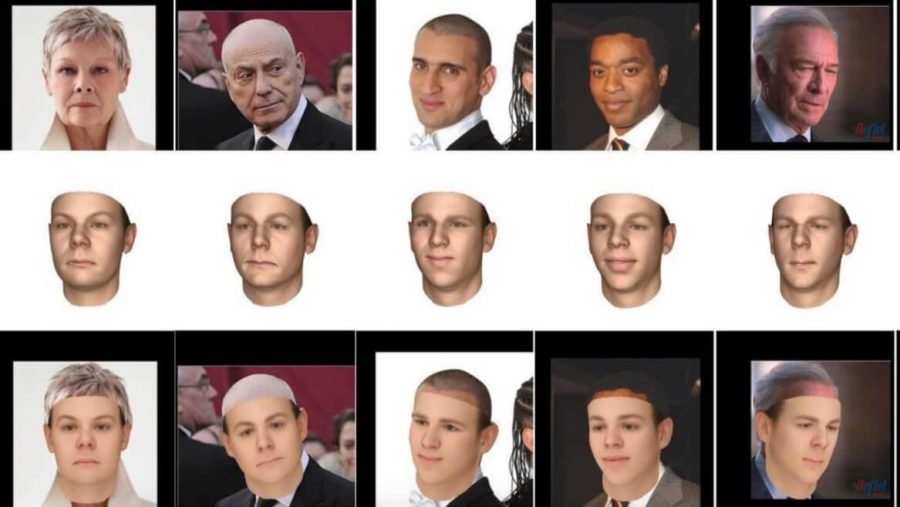
3D face geometry needs to be recovered from 2D images in many real-world applications, including face recognition, face landmark detection, 3D emoticon animation etc. However, this task remains challenging especially under the large pose, when much of the information about the face is unknowable.
Jiang and Wu from Jiangnan University (China) and Kittler from University of Surrey (UK) suggest a novel 3D face reconstruction algorithm, which significantly improves the accuracy of reconstruction even under extreme pose.
But let’s first shortly review the previous work on 3D face models and 3D face reconstruction.
Related Work
The research mentions four publicly available 3D deformation models:
- BFM model proposed by the University of Basel;
- 3DMM models developed by Brunton et.al;
- a multi-resolution 3D face model provided by University of Surrey (UK);
- the large-scale face model (LSFM) built by Imperial College.
This paper uses a BFM model, which is the most popular.
There are several approaches to reconstructing 3D model from 2D images, including:
- a cascaded regression method;
- combining face key point detection and 3D face reconstruction and using 3d-shape-feature-indexing to construct the tree-based regression model;
- 3D morphable model based on Pose and Expression normalization method;
- an extended 3DMM (E-3DMM), which accounts for the variation of face expression;
- performing a weighted landmark 3DMM fitting based on the traditional regression method.
State-of-the-art idea
The paper by Jiang, Wu, and Kittler proposes a novel Pose-Invariant 3D Face Reconstruction (PIFR) algorithm based on 3D Morphable Model (3DMM).
Firstly, they suggest generating a frontal image by normalizing a single face input image. This step allows restoring additional identity information of the face.
The next step is to use a weighted sum of the 3D parameters of both images: the frontal one and the original one. This allows to preserve the pose of the original image, but also enhance the identity information.
The pipeline for the suggested approach is provided below.
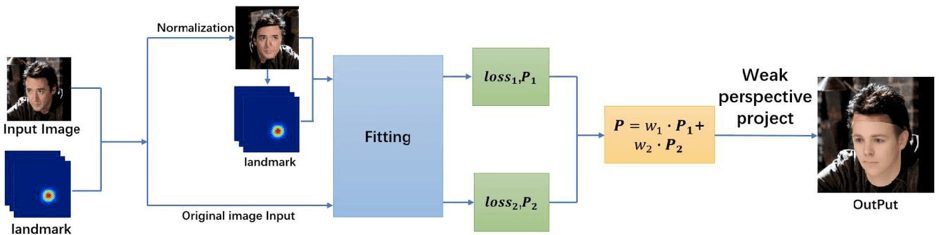
The experiments show that PIFR algorithm has significantly improved the performance of 3D face reconstruction compared to the previous methods, especially in the extreme pose cases.
Let’s now have a closer look at the suggested model…
Model details
PIFR method is largely relying on the 3DMM fitting process, which can be expressed as minimizing the error between the 2D coordinates of the 3D point projection and the ground truth. However, the face generated by the 3D model has about 50,000 vertices, and thus iterative calculations result in the slow and ineffective convergence. To overcome this problem, the researchers suggest using the landmarks (e.g., eye center, mouth corner, and nose tip) as the ground truth in the fitting process. Specifically, they use a weighted landmark 3DMM fitting.
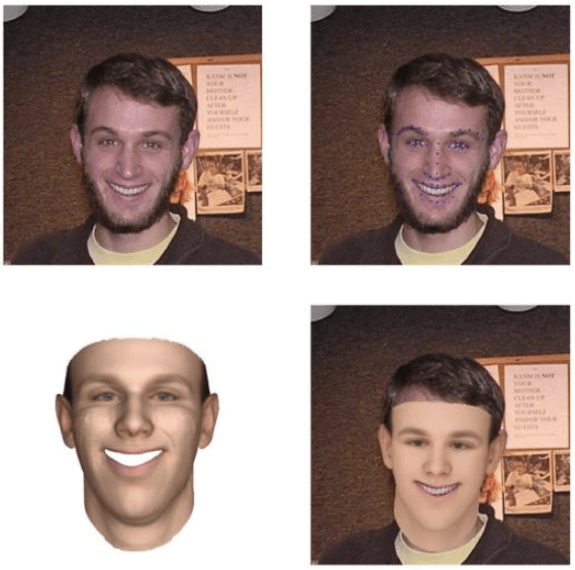
The next challenge is to reconstruct 3D faces in large poses. To solve this problem, the researchers use High-Fidelity Pose and Expression Normalization (HPEN) method, but only for normalization of the pose and not expression. Also, Poisson Editing is used to recover the occluded area of the face due to the angle.
Performance Comparison with Other Methods
The performance of PIFR method was evaluated for the face reconstruction:
- in small and medium poses;
- large poses;
- extreme poses (±90 yaw angles).
For this purpose, the researchers used three publicly available datasets:
- AFW dataset, which was created using Flickr images, contains 205 images with 468 marked faces, complex backgrounds and face poses.
- LFPW dataset, which has 224 face images in the test set and 811 face images in the training set; each image is marked with 68 feature points; 900 face images from both sets were selected for testing in this research.
- AFLW dataset is a large-scale face database, which contains around 250 million hand-labeled face images, and each image is marked with 21 feature points. This study used only extreme pose face images from this dataset for qualitative analysis.
Quantitative analysis. Using the Mean Euclidean Metric (MEM), the study compares the performance of PIFR method to E-3DMM and FW-3DMM on AFW and LFPW datasets. Cumulative errors distribution (CED) curves look like this:
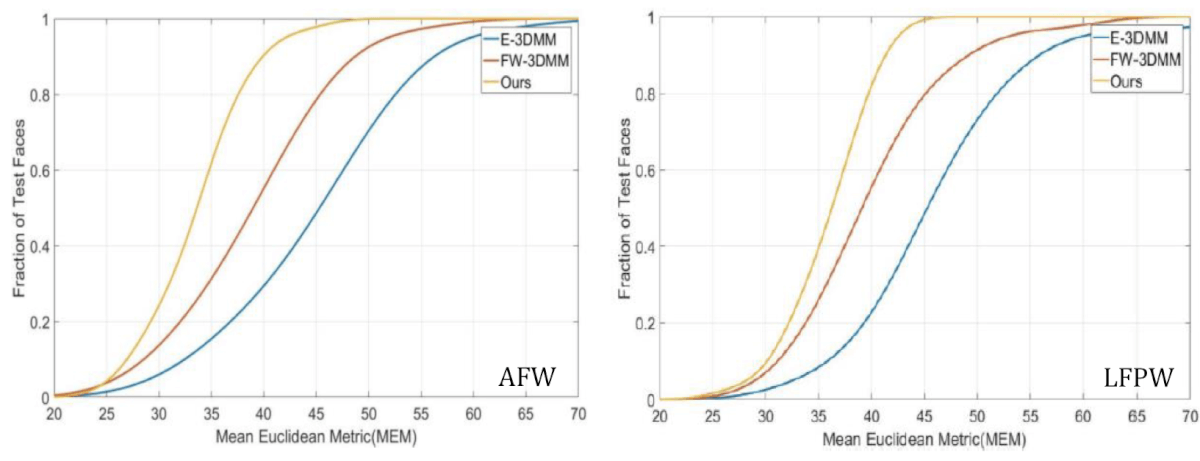
As you can see from these plots and the tables below, PIFR method shows superior performance compared to the other two methods. Its reconstruction performance in large poses is particularly good.
Qualitative analysis. The method was also assessed qualitatively based on the face images in extreme poses from AFLW dataset. The results are shown in the figure below.
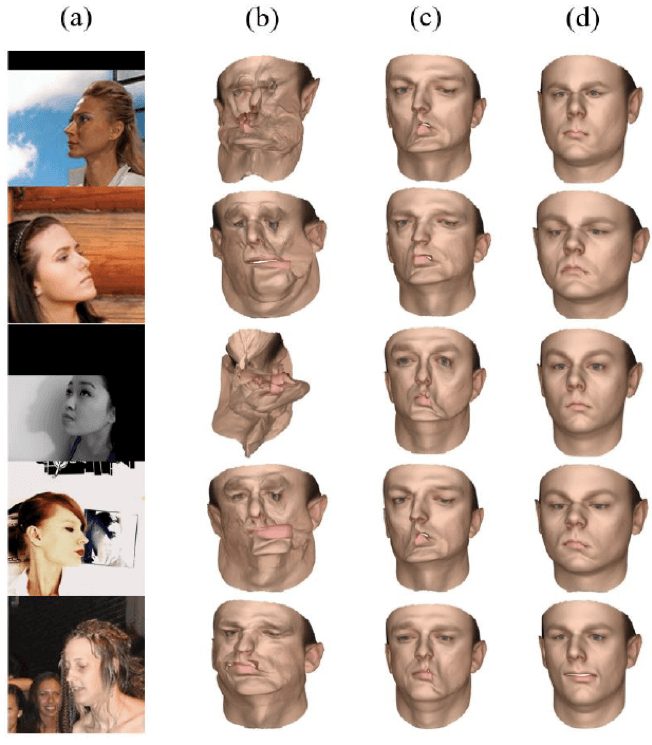
Even though half of the landmarks are invisible due to extreme posture, which leads to large errors and failures of other methods, the PIFR method still performs quite well.
Here are some additional examples of the PIFR method performance based on the images from the AFW dataset.
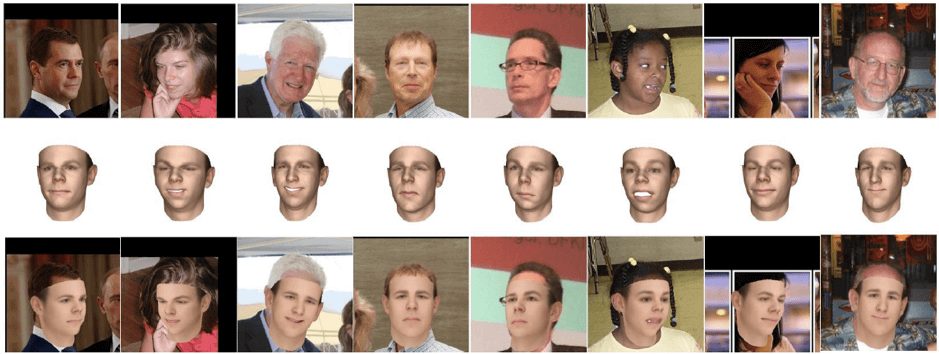
Bottom Line
A novel 3D face reconstruction framework PIFR demonstrates good reconstruction performance even in extreme poses. By taking both the original and the frontal images for weighted fusion, the method allows restoring enough face information to reconstruct the 3D face.
In the future, the researchers plan to restore even more facial identity information to improve the accuracy of reconstruction further.
[…] Представлем вашему вниманию перевод статьи «PIFR: Pose Invariant 3D Face Reconstruction». […]
[…] Представлем вашему вниманию перевод статьи «PIFR: Pose Invariant 3D Face Reconstruction». […]